One of the most important processes that we can apply machine learning to is the preprocessing of feature vectors. Data in the real world is undoubtedly dirty and noisy in general. We need to pick the most useful features when it comes to prediction. Due to this, it is important to clean the data. This contributes not only to improving the accuracy of the prediction, but also reducing the time it takes for training by saving the size of the input data. In this chapter, we are going to introduce two popular ways to extract meaningful features from the original data. The first approach we will look at is principal component analysis (PCA), an unsupervised learning technique that projects the original data into a low-dimensional space. The other is an embedding technique that's typically used by NLP problems with t-SNE, which is a more advanced dimensionality...




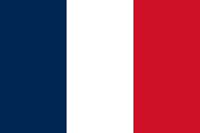


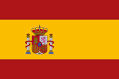



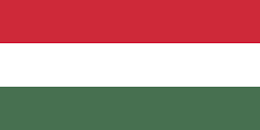

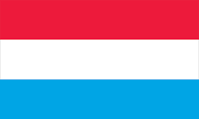





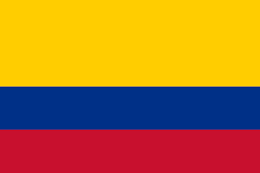
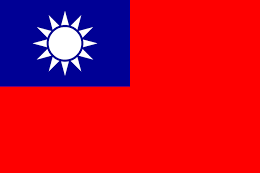
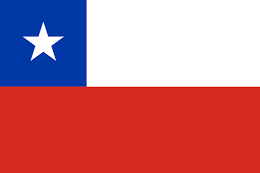



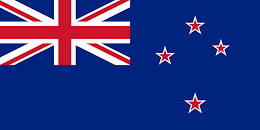
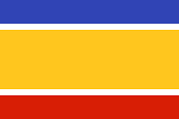
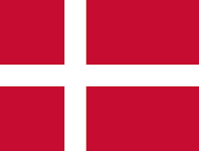
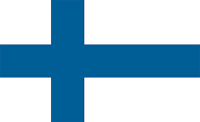







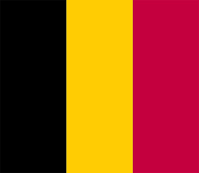

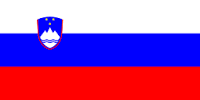





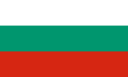


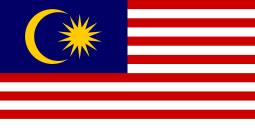



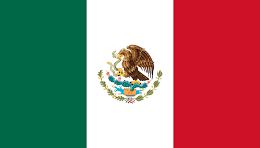
