An overview and the intuition of CNN
CNN consists of multiple layers of convolutions, polling and finally fully connected layers. This is much more efficient than pure feedforward networks we discussed in Chapter 2, Deep Feedforward Networks.
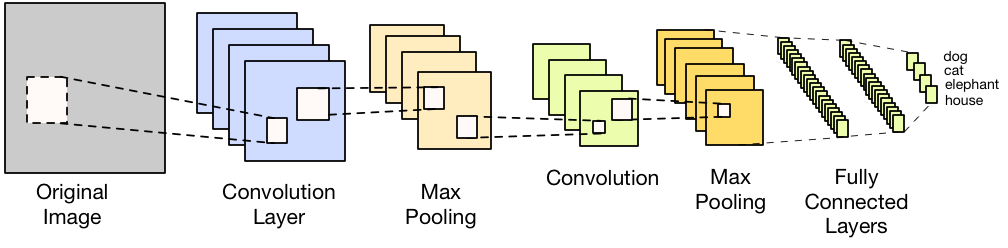
The preceding diagram takes images through Convolution Layer | Max Pooling | Convolution | Max Pooling | Fully Connected Layers this is an CNN architecture
Single Conv Layer Computation
Let's first discuss what the conv layer computes intuitively. The Conv layer's parameters consist of a set of learnable filters (also called tensors). Each filter is small spatially (depth, width, and height), but extends through the full depth of the input volume (image). A filter on the first layer of a ConvNet typically has a size of 5 x 5 x 3 (that is, five pixels width and height, and three for depth, because images have three depths for color channels). During the forward pass, filters slide (or convolve) across the width and height of the input volume and compute...