ML engineering in the real world
The majority of us who work in machine learning, analytics, and related disciplines do so for for-profit companies. It is important therefore that we consider some of the important aspects of doing this type of work in the real world.
First of all, the ultimate goal of your work is to generate value. This can be calculated and defined in a variety of ways, but fundamentally your work has to improve something for the company or their customers in a way that justifies the investment put in. This is why most companies will not be happy for you to take a year to play with new tools and then generate nothing concrete to show for it (not that you would do this anyway, it is probably quite boring) or to spend your days reading the latest papers and only reading the latest papers. Yes, these things are part of any job in technology, and especially any job in the world of machine learning, but you have to be strategic about how you spend your time and always be aware of your value proposition.
Secondly, to be a successful ML engineer in the real world, you cannot just understand the technology; you must understand the business. You will have to understand how the company works day to day, you will have to understand how the different pieces of the company fit together, and you will have to understand the people of the company and their roles. Most importantly, you have to understand the customer, both of the business and of your work. If you do not know the motivations, pains, and needs of the people you are building for, then how can you be expected to build the right thing?
Finally, and this may be controversial, the most important skill for you being a successful ML engineer in the real world is one that this book will not teach you, and that is the ability to communicate effectively. You will have to work in a team, with a manager, with the wider community and business, and, of course, with your customers, as mentioned above. If you can do this and you know the technology and techniques (many of which are discussed in this book), then what can stop you?
But what kind of problems can you solve with machine learning when you work in the real world? Well, let's start with another potentially controversial statement: a lot of the time, machine learning is not the answer. This may seem strange given the title of this book, but it is just as important to know when not to apply machine learning as when to apply it. This will save you tons of expensive development time and resources.
Machine learning is ideal for cases when you want to do a semi-routine task faster, with more accuracy, or at a far larger scale than is possible with other solutions. Some typical examples are given in the following table, along with some discussion as to whether or not ML would be an appropriate tool for solving the problem:
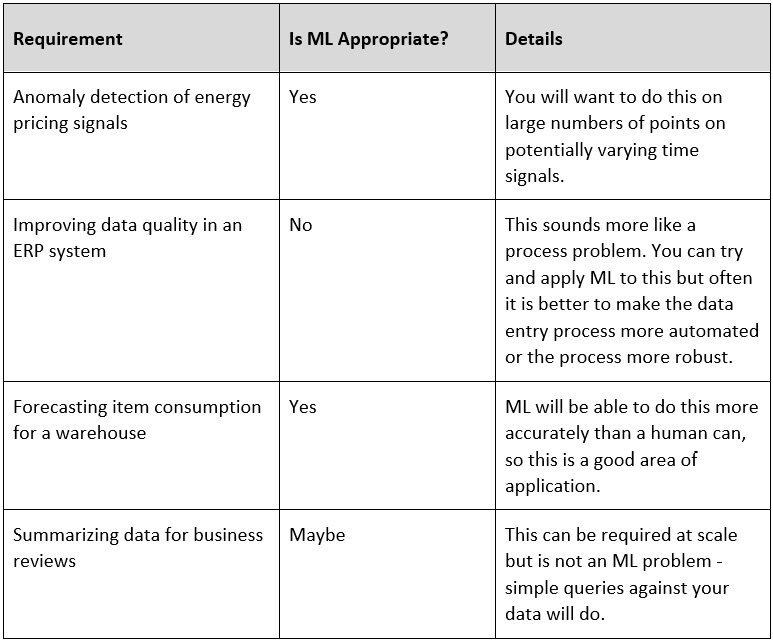
Figure 1.2 – Potential use cases for ML
As this table of simple examples hopefully starts to make clear, the cases where machine learning is the answer are ones that can usually be very well framed as a mathematical or statistical problem. After all, this is what machine learning really is; a series of algorithms rooted in mathematics that can iterate some internal parameters based on data. Where the lines start to blur in the modern world are through advances in areas such as deep learning or reinforcement learning, where problems that we previously thought would be very hard to phrase appropriately for standard ML algorithms can now be tackled.
The other tendency to watch out for in the real world (to go along with let's use ML for everything) is the worry that people have that ML is coming for their job and should not be trusted. This is understandable: a report by PwC in 2018 suggested that 30% of UK jobs will be impacted by automation by the 2030s (Will Robots Really Steal Our Jobs?: https://www.pwc.co.uk/economic-services/assets/international-impact-of-automation-feb-2018.pdf). What you have to try and make clear when working with your colleagues and customers is that what you are building is there to supplement and augment their capabilities, not to replace them.
Let's conclude this section by revisiting an important point: the fact that you are working for a company means, of course, that the aim of the game is to create value appropriate to the investment. In other words, you need to show a good Return On Investment (ROI). This means a couple of things for you practically:
- You have to understand how different designs require different levels of investment. If you can solve your problem by training a deep neural net on a million images with a GPU running 24/7 for a month, or you know you can solve the same problem with some basic clustering and a bit of statistics on some standard hardware in a few hours, which should you choose?
- You have to be clear about the value you will generate. This means you need to work with experts and try to translate the results of your algorithm into actual dollar values. This is so much more difficult than it sounds, so you should take the time you need to get it right. And never, ever over-promise. You should always under-promise and over-deliver.
Adoption is not guaranteed. Even when building products for your colleagues within a company, it is important to understand that your solution will be tested every time someone uses it post-deployment. If you build shoddy solutions, then people will not use them, and the value proposition of what you have done will start to disappear.
Now that you understand some of the important points when using ML to solve business problems, let's explore what these solutions can look like.