Data analytics
Data analytics is basically a structured process of using statistical modeling, machine learning, knowledge discovery, predictive modeling and data mining to discover and interpret meaningful patterns in the data, and to communicate them effectively as actionable insights which help in driving business decisions. Data analytics, data mining and machine learning are often said to be covering similar concepts and methodologies. While machine learning, being a branch or subset of artificial intelligence, is more focused on model building, evaluation and learning patterns, the end goal of all three processes is the same: to generate meaningful insights from the data. In the next section, we will briefly discuss the industry standard process followed in analytics which is rigorously followed by organizations.
Analytics workflow
Analyzing data is a science and an art. Any analytics process usually has a defined set of steps, which are generally executed in sequence. More than often, analytics being an iterative process, it leads to several of these steps being repeated many times over if necessary. There is an industry standard that is widely followed for data analysis, known as CRISP-DM, which stands for Cross Industry Standard Process for Data Mining. This is a standard data analysis and mining process workflow that describes how to break up any particular data analysis problem into six major stages.
The main stages in the CRISP-DM model are as follows:
- Business understanding: This is the initial stage that focuses on the business context of the problem, objective or goal which we are trying to solve. Domain and business knowledge are essential here along with valuable insights from subject matter experts in the business for planning out the key objectives and end results that are intended from the data analysis workflow.
- Data acquisition and understanding: This stage's main focus is to acquire data of interest and understand the meaning and semantics of the various data points and attributes that are present in the data. Some initial exploration of the data may also be done at this stage using various exploratory data analysis techniques.
- Data preparation: This stage usually involves data munging, cleaning, and transformation. Extract-Transform-Load (ETL) processes of ten come in handy at this stage. Data quality issues are also dealt with in this stage. The final dataset is usually used for analysis and modeling.
- Modeling and analysis: This stage mainly focuses on analyzing the data and building models using specific techniques from data mining and machine learning. Often, we need to apply further data transformations that are based on different modeling algorithms.
- Evaluation: This is perhaps one of the most crucial stages. Building models is an iterative process. In this stage, we evaluate the results that are obtained from multiple iterations of various models and techniques, and then we select the best possible method or analysis, which gives us the insights that we need based on our business requirements. Often, this stage involves reiterating through the previous two steps to reach a final agreement based on the results.
- Deployment: This is the final stage, where decision systems that are based on analysis are deployed so that end users can start consuming the results and utilizing them. This deployed system can be as complex as a real-time prediction system or as simple as an ad-hoc report.
The following figure shows the complete flow with the various stages in the CRISP-DM model:
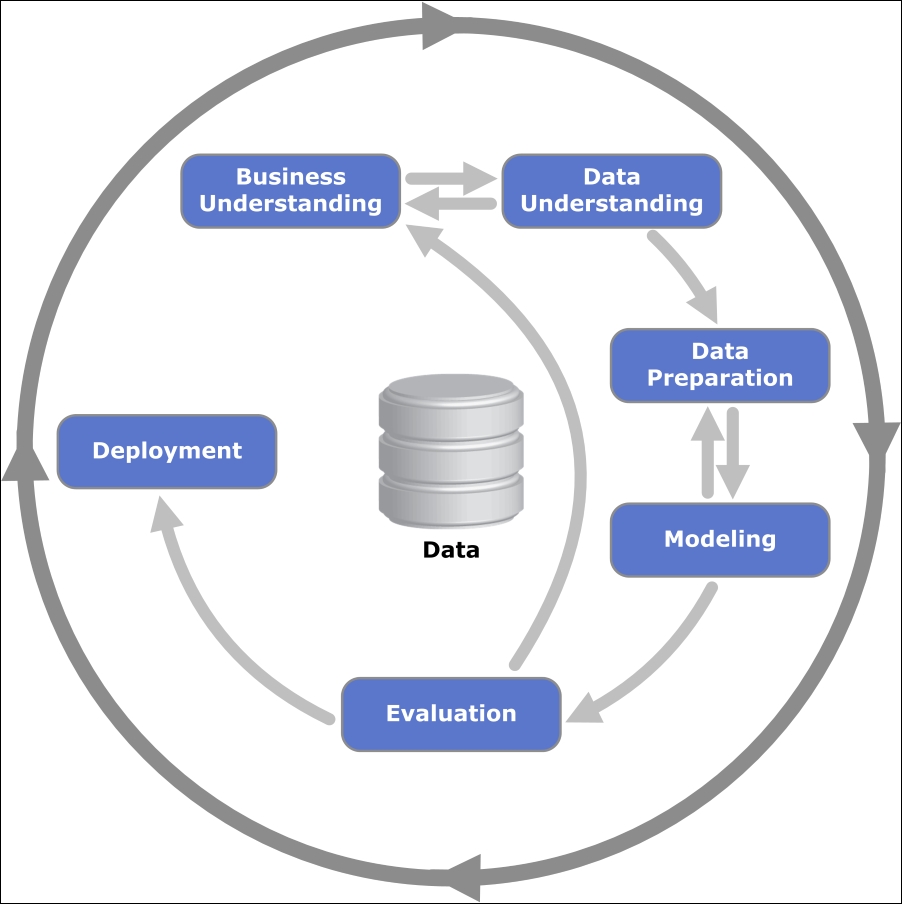
The CRISP-DM model. Source: www.wikipedia.org
In principle, the CRISP-DM model is very clear and concise with straightforward requirements at each step. As a result, this workflow has been widely adopted across the industry and has become an industry standard.