In this chapter, we took an in-depth look at DP and the Bellman equation. The Bellman equation with DP has influenced RL significantly by introducing the concept of future rewards and optimization. We covered the contribution of Bellman in this chapter by first taking a deep look at DP and how to solve a problem dynamically. Then, we advanced to understanding the Bellman optimality equation and how it can be used to account for future rewards as well as determine expected state and action values using iterative methods. In particular, we focused on the implementation in Python of policy iteration and improvement. Then, from there, we looked at value iteration. Finally, we concluded this chapter by setting up an agent test against the FrozenLake environment using a policy generated by both policy and value iteration. For this chapter, we looked at a specific class of problems...




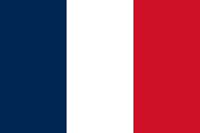


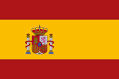



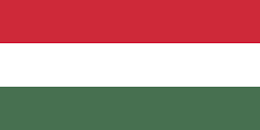

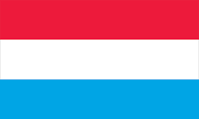





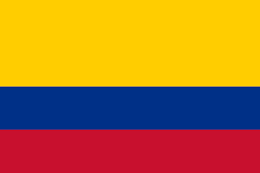
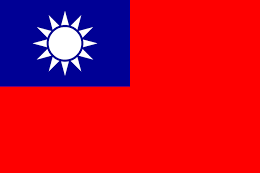
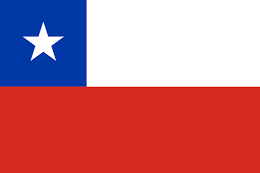



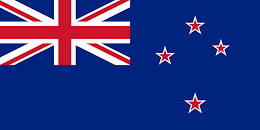
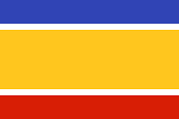
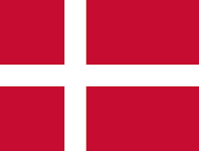
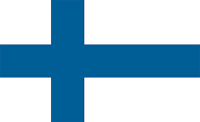







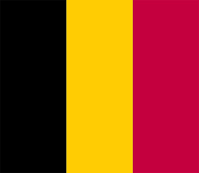

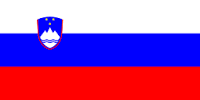





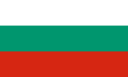


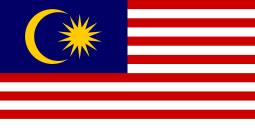



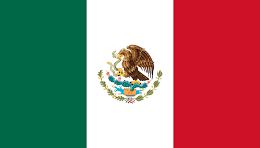
