In this section, we'll set up learning and optimization functions, compile the model, fit it to training and testing data, and then actually run the model and see an animation indicating the effects on loss and accuracy.
In the following screenshot, we are compiling our model with loss, optimizer, and metrics:
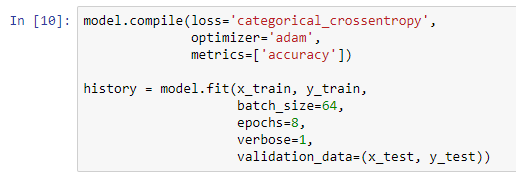
The loss function is a mathematical function that tells optimizer how well it's doing. An optimizer function is a mathematical program that searches the available parameters in order to minimize the loss function. The metrics parameter are outputs from your machine learning model that should be human readable so that you can understand how well your model is running. Now, these loss and optimizer parameters are laden with math. By and large, you can approach this as a cookbook. When you are running a machine learning model with Keras, you...