3.1 Sharing information, sharing priors
Hierarchical models are also known as multilevel models, mixed-effects models, random-effects models, or nested models. They are particularly useful when dealing with data that can be described as grouped or having different levels, such as data nested within geographic regions (for example, cities belonging to a province and provinces belonging to a country), or with a hierarchical structure (such as students nested within schools, or patients nested within hospitals) or repeated measurements on the same individuals.
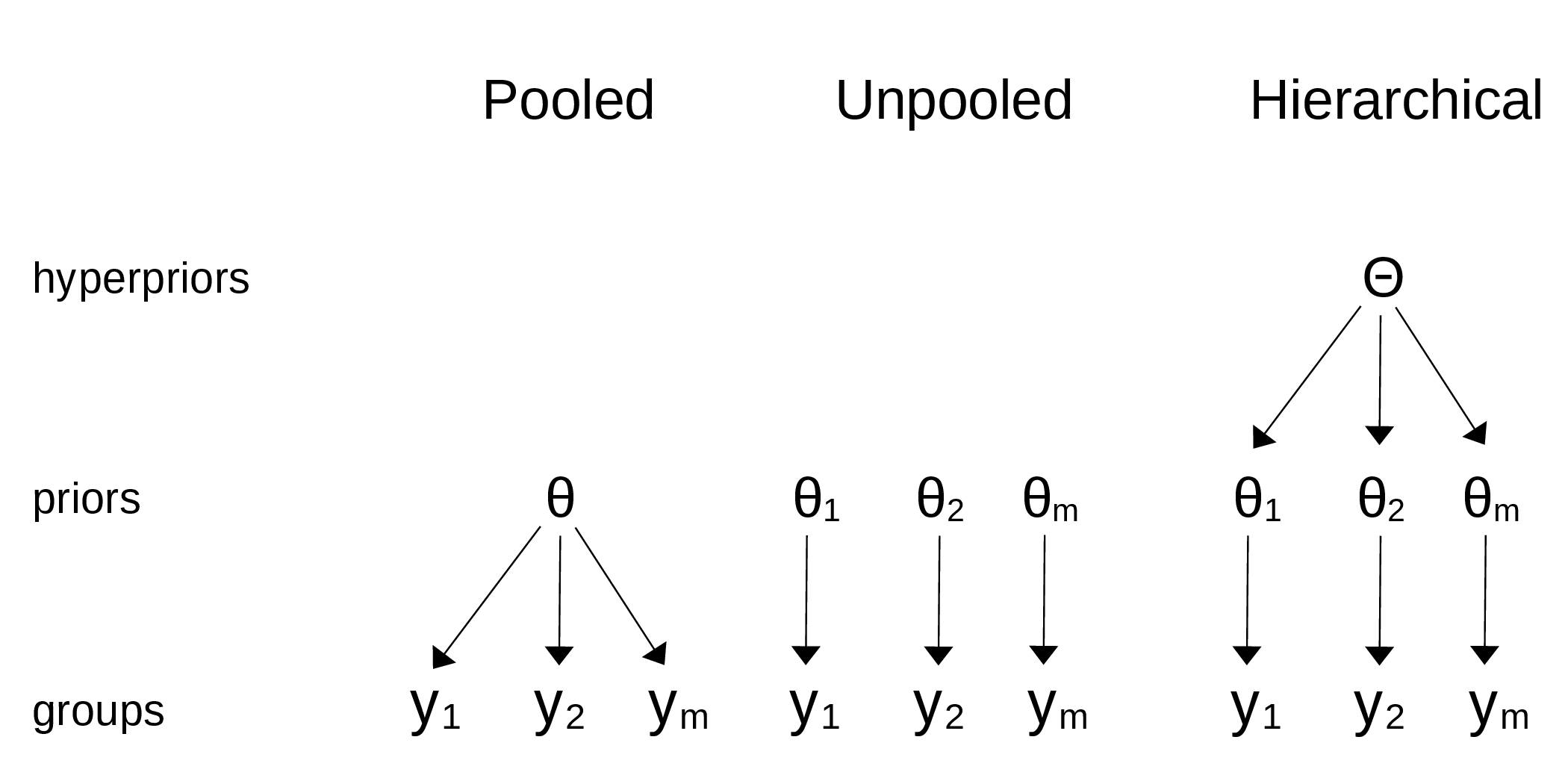
Figure 3.1: The differences between a pooled model, an unpooled model, and a hierarchical model
Hierarchical models are a natural way to share information between groups. In a hierarchical model, the parameters of the prior distributions are themselves given a prior distribution. These higher-level priors are often called hyperpriors; ”hyper” means ”over” in Greek. Having hyperpriors allows the model...