



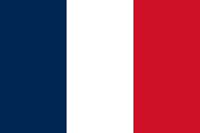


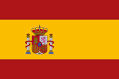



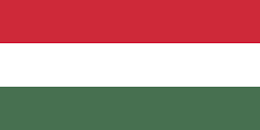

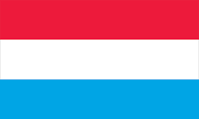





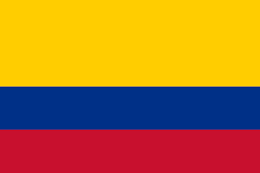
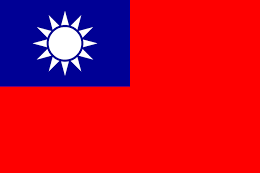
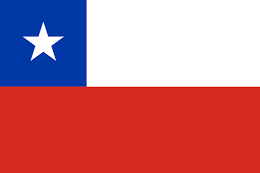



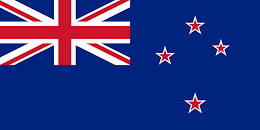
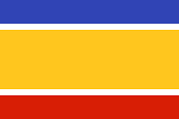
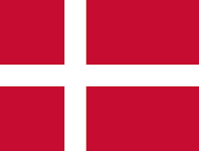
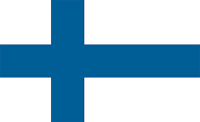







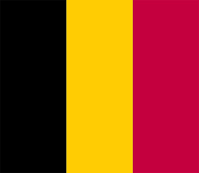

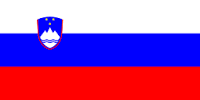





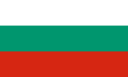


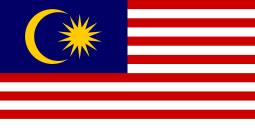



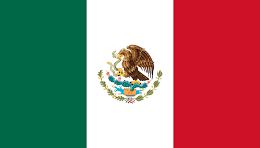

Caffe2 is a lightweight, modular, scalable deep learning framework developed by Facebook. It is a successor of Caffe, a project started at the University of California, Berkeley. It is primarily built for production use cases and mobile development and offers developers greater flexibility for building high-performance products.
Caffe2 aims to provide an easy way to experiment with deep learning and leverage community contributions of new models and algorithms. It is cross-platform and integrates with Visual Studio, Android Studio, and Xcode for mobile development.
Its core C++ libraries provide speed and portability, while its Python and C++ APIs make it easy for you to prototype, train, and deploy your models. It utilizes GPUs when they are available. It is fine-tuned to take full advantage of the NVIDIA GPU deep learning platform. To deliver high performance, Caffe2 uses some of the deep learning SDK libraries by NVIDIA such as cuDNN, cuBLAS, and NCCL.
Facebook is using Caffe2 to help their developers and researchers train large machine learning models and deliver AI on mobile devices. Using Caffe2, they significantly improved the efficiency and quality of machine translation systems. As a result, all machine translation models at Facebook have been transitioned from phrase-based systems to neural models for all languages.
OpenCV short for Open Source Computer Vision Library is a collection of programming functions for real-time computer vision and machine learning. It has C++, Python, and Java interfaces and supports Windows, Linux, Mac OS, iOS and Android. It also supports the deep learning frameworks TensorFlow and PyTorch. Written natively in C/C++, the library can take advantage of multi-core processing.
OpenCV aims to provide a common infrastructure for computer vision applications and to accelerate the use of machine perception in the commercial products. The library consists of more than 2500 optimized algorithms including both classic and state-of-the-art computer vision algorithms.
These algorithms can be used for the following:
Plickers is an assessment tool, that lets you poll your class for free, without the need for student devices. It uses OpenCV as its graphics and video SDK. You just have to give each student a card called a paper clicker, and use your iPhone/iPad to scan them to do instant checks-for-understanding, exit tickets, and impromptu polls.
TensorFlow is an open source software library for building machine learning models. Its flexible architecture allows easy model deployment across a variety of platforms ranging from desktops to mobile and edge devices. Currently, TensorFlow provides two solutions for deploying machine learning models on mobile devices: TensorFlow Mobile and TensorFlow Lite.
TensorFlow Lite is an improved version of TensorFlow Mobile, offering better performance and smaller app size. Additionally, it has very few dependencies as compared to TensorFlow Mobile, so it can be built and hosted on simpler, more constrained device scenarios. TensorFlow Lite also supports hardware acceleration with the Android Neural Networks API.
But the catch here is that TensorFlow Lite is currently in developer preview and only has coverage to a limited set of operators. So, to develop production-ready mobile TensorFlow apps, it is recommended to use TensorFlow Mobile.
Also, TensorFlow Mobile supports customization to add new operators not supported by TensorFlow Mobile by default, which is a requirement for most of the models of different AI apps. Although TensorFlow Lite is in developer preview, its future releases “will greatly simplify the developer experience of targeting a model for small devices”. It is also likely to replace TensorFlow Mobile, or at least overcome its current limitations.
The Alibaba tech team is using TensorFlow Lite to implement and optimize speaker recognition on the client side. This addresses many of the common issues of the server-side model, such as poor network connectivity, extended latency, and poor user experience.
Google uses TensorFlow for advanced machine learning models including Google Translate and RankBrain.
Core ML is a machine learning framework which can be used to integrate machine learning model in your iOS apps. It supports Vision for image analysis, Natural Language for natural language processing, and GameplayKit for evaluating learned decision trees.
Core ML is built on top of the following low-level APIs, providing a simple higher level abstraction to these:
To train and deploy custom models you can also use the Create ML framework. It is a machine learning framework in Swift, which can be used to train models using native Apple technologies like Swift, Xcode, and Other Apple frameworks.
Lumina is a camera designed in Swift for easily integrating Core ML models - as well as image streaming, QR/Barcode detection, and many other features.
ML Kit is a cross-platform suite of machine learning tools for its Firebase mobile development platform. It comprises of Google's ML technologies, such as the Google Cloud Vision API, TensorFlow Lite, and the Android Neural Networks API together in a single SDK enabling you to apply ML techniques to your apps easily.
You can leverage its ready-to-use APIs for common mobile use cases such as recognizing text, detecting faces, identifying landmarks, scanning barcodes, and labeling images. If these APIs don't cover your machine learning problem, you can use your own existing TensorFlow Lite models. You just have to upload your model on Firebase and ML Kit will take care of the hosting and serving.
These APIs can run on-device or in the cloud. Its on-device APIs process your data quickly and work even when there’s no network connection. Its cloud-based APIs leverage the power of Google Cloud Platform's machine learning technology to give you an even higher level of accuracy.
A popular calorie counter app, Lose It! uses Google ML Kit Text Recognition API to quickly capture nutrition information to ensure it’s easy to record and extremely accurate.
PicsArt uses ML Kit custom model APIs to provide TensorFlow–powered 1000+ effects to enable millions of users to create amazing images with their mobile phones.
Dialogflow is a Natural Language Understanding (NLU) platform that makes it easy for developers to design and integrate conversational user interfaces into mobile apps, web applications, devices, and bots. You can integrate it on Alexa, Cortana, Facebook Messenger, and other platforms your users are on.
With Dialogflow you can build interfaces, such as chatbots and conversational IVR that enable natural and rich interactions between your users and your business. It provides this human-like interaction with the help of agents. Agents can understand the vast and varied nuances of human language and translate that to standard and structured meaning that your apps and services can understand.
It comes in two types: Dialogflow Standard Edition and Dialogflow Enterprise Edition. Dialogflow Enterprise Edition users have access to Google Cloud Support and a service level agreement (SLA) for production deployments.
Domino’s simplified the process of ordering pizza using Dialogflow’s conversational technology. Domino's leveraged large customer service knowledge and Dialogflow's NLU capabilities to build both simple customer interactions and increasingly complex ordering scenarios.
Cognitive Services is a collection of APIs, SDKs, and services to enable developers easily add cognitive features to their applications such as emotion and video detection, facial, speech, and vision recognition, among others.
You need not be an expert in data science to make your systems more intelligent and engaging. The pre-built services come with high-quality RESTful intelligent APIs for the following:
To safeguard against fraud, Uber uses the Face API, part of Microsoft Cognitive Services, to help ensure the driver using the app matches the account on file.
Cardinal Blue developed an app called PicCollage, a popular mobile app that allows users to combine photos, videos, captions, stickers, and special effects to create unique collages.
These were some of the tools that will help you integrate intelligence into your apps. These libraries make it easier to add capabilities like speech recognition, natural language processing, computer vision, and many others, giving users the wow moment of accomplishing something that wasn’t quite possible before.
Along with choosing the right AI tool, you must also consider other factors that can affect your app performance. These factors include the accuracy of your machine learning model, which can be affected by bias and variance, using correct datasets for training, seamless user interaction, and resource-optimization, among others.
While building any intelligent app it is also important to keep in mind that the AI in your app is solving a problem and it doesn’t exist because it is cool. Thinking from the user’s perspective will allow you to assess the importance of a particular problem. A great AI app will not just help users do something faster, but enable them to do something they couldn’t do before.
With the growing popularity and the need to speed up the development of intelligent apps, many companies ranging from huge tech giants to startups are providing AI solutions. In the future we will definitely see more developer tools coming into the market, making AI in apps a norm.
6 most commonly used Java Machine learning libraries
5 ways artificial intelligence is upgrading software engineering
Machine Learning as a Service (MLaaS): How Google Cloud Platform, Microsoft Azure, and AWS are democratizing Artificial Intelligence