Some more terminology
This is a good time to define some more vocabulary. By this point, you're probably excitedly looking up a lot of data science material and seeing words and phrases I haven't used yet. Here are some common terms that you are likely to encounter.
- Machine learning: This refers to giving computers the ability to learn from data without explicit "rules" being given by a programmer. We have seen the concept of machine learning earlier in this chapter as the union of someone who has both coding and math skills. Here, we are attempting to formalize this definition. Machine learning combines the power of computers with intelligent learning algorithms in order to automate the discovery of relationships in data and create powerful data models. Speaking of data models, in this book, we will concern ourselves with the following two basic types of data model:
- Probabilistic model: This refers to using probability to find a relationship between elements that includes a degree of randomness.
- Statistical model: This refers to taking advantage of statistical theorems to formalize relationships between data elements in a (usually) simple mathematical formula.
Note
While both the statistical and probabilistic models can be run on computers and might be considered machine learning in that regard, we will keep these definitions separate, since machine learning algorithms generally attempt to learn relationships in different ways. We will take a look at the statistical and probabilistic models in later chapters.
- Exploratory data analysis (EDA): This refers to preparing data in order to standardize results and gain quick insights. EDA is concerned with data visualization and preparation. This is where we turn unorganized data into organized data and clean up missing/incorrect data points. During EDA, we will create many types of plots and use these plots to identify key features and relationships to exploit in our data models.
- Data mining: This is the process of finding relationships between elements of data. Data mining is the part of data science where we try to find relationships between variables (think the spawn-recruit model).
I have tried pretty hard not to use the term big data up until now. This is because I think this term is misused, a lot. Big data is data that is too large to be processed by a single machine (if your laptop crashed, it might be suffering from a case of big data).
The following diagram shows the relationship between these data science concepts:
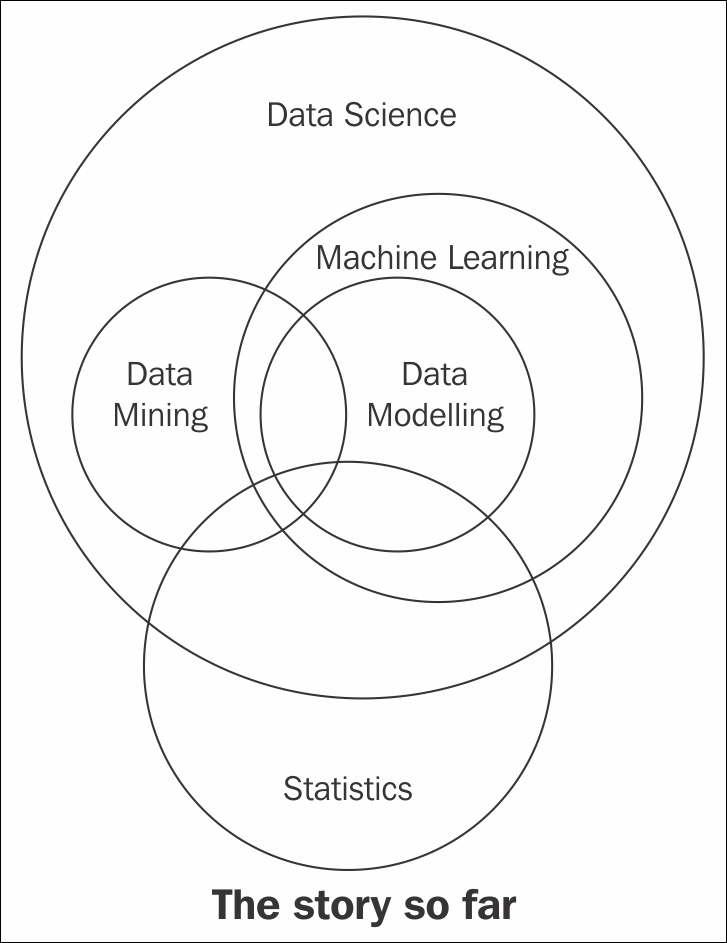
The state of data science (so far)
The preceding diagram is incomplete and is meant for visualization purposes only.