Ensemble methods are based on a very simple idea: instead of using a single model to make a prediction, we use many models and then use some method to aggregate the predictions. Having different models is like having different points of view, and it has been demonstrated that by aggregating models that offer a different point of view; predictions can be more accurate. These methods further improve generalization over a single model because they reduce the risk of selecting a poorly performing classifier:
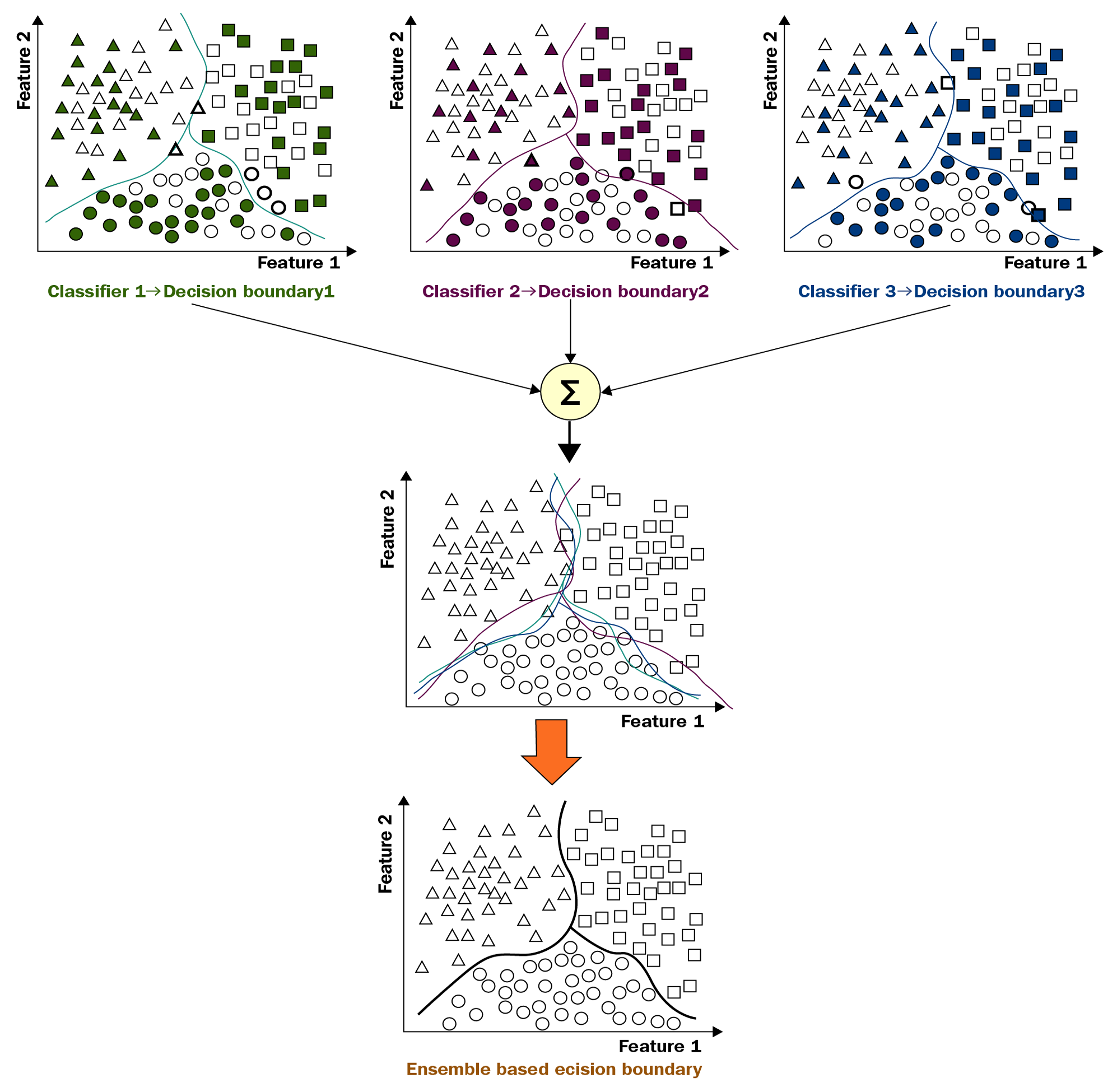
In the preceding diagram, we can see that each object belongs to one of three classes: triangles, circles, and squares. In this simplified example, we have two features to separate or classify the objects into the different classes. As you can see here, we can use three different classifiers and all the three classifiers represent different approaches and have different kinds of decision boundaries.
Ensemble learning combines all those individual predictions into a single one. The predictions made from combining the three boundaries usually have better properties than the ones produced by the individual models. This is the simple idea behind ensemble methods, also called ensemble learning.
The most commonly used ensemble methods are as follows:
- Bootstrap sampling
- Bagging
- Random forests
- Boosting
Before giving a high-level explanation of these methods, we need to discuss a very important statistical technique known as bootstrap sampling.