Fine-tuning the clustering
Deciding the optimum value of K is one of the tough parts while performing a k-means clustering. There are a few methods that can be used to do this.
The elbow method
We earlier discussed that a good cluster is defined by the compactness between the observations of that cluster. The compactness is quantified by something called intra-cluster distance. The intra-cluster distance for a cluster is essentially the sum of pair-wise distances between all possible pairs of points in that cluster.
If we denote intra-cluster distance by W, then for a cluster k intra-cluster, the distance can be denoted by:
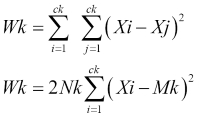
Generally, the normalized intra-cluster distance is used, which is given by:
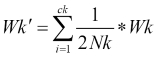
Here Xi and Xj are points in the cluster, Mk is the centroid of the cluster, Nk is the number of points in the centroid, and K is the number of clusters.
Wk' is actually a measure of the variance between the points in the same cluster. Since it is normalized, its value would range from 0 to...