Since our first example of DQN, we have been using experience replay internally to more efficiently train an agent. ER involves nothing more than storing the agent's experiences in the form of a <state,action,reward,next state> tuple that fills a buffer. The agent then randomly walks or samples through this buffer of experiences in training. This has the benefit of keeping the agent more generalized and avoiding localized patterns. The following is an updated diagram of what our learning flow looks like when we add experience replay:
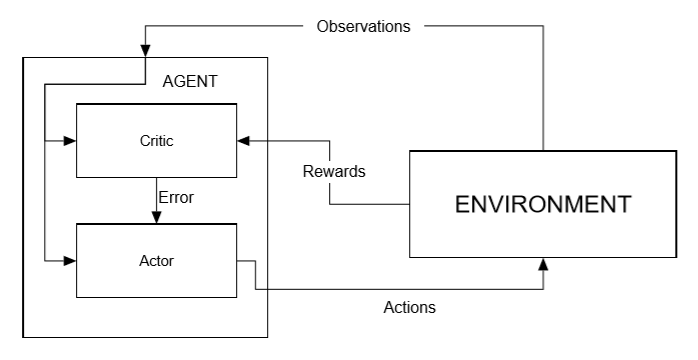
Diagram of RL with experience replay added
In the preceding diagram, you can see how the agent stores experiences in a buffer memory that it then randomly samples from at each step. As the buffer fills, older experiences are discarded. This may seem quite counter-intuitive, since our goal is to find the best or optimal...