Now, we will see in more detail what we need to do in an MTL task.
There are different ways to implement MTL. Two methods that are commonly used are as follows:
- Hard parameter sharing: This is the most common way to implement MTL, and it consists of sharing some of the hidden layers across all tasks, while other layers are kept specific for each single task:
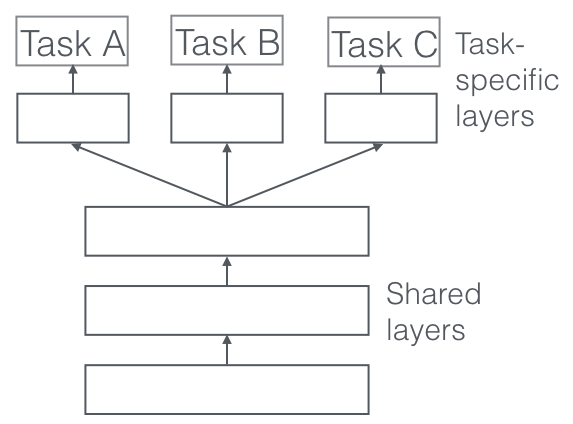
The main advantage of this method is that it's difficult to overfit. Overfitting is particularly a problem for NNs, but in this case, the more tasks, the lower the danger of overfitting. This is quite clear, because overfitting is creating a solution that is too specific for the dataset we provide, while in this case, by design, we have a more generic task and a variegated dataset.
- Soft parameter sharing: With soft parameter sharing, we have one model, but each task will have its own parameters. In...