SVMs are an example of supervised algorithms (as well as the Perceptron), whose task is to identify the hyperplane that best separates classes of data that can be represented in a multidimensional space. It is possible, however, to identify different hyperplanes that correctly separate the data from each other; in this case, the choice falls on the hyperplane that optimizes the prefixed margin, that is, the distance between the hyperplane and the data.
One of the advantages of the SVM is that the identified hyperplane is not limited to the linear model (unlike the Perceptron), as shown in the following screenshot:
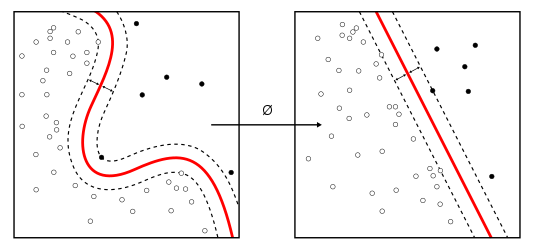
The SVM can be considered as an extension of the Perceptron, however. While in the case of the Perceptron, our goal was to minimize classification errors, in the case of SVM, our goal instead is to maximize the margin, that is, the distance...