V's of big data
Typically, the problem that comes in the bracket of big data is defined by terms that are often called as V's of big data. There are typically three V's, which are Volume, Velocity, and variety, as shown in the following image:
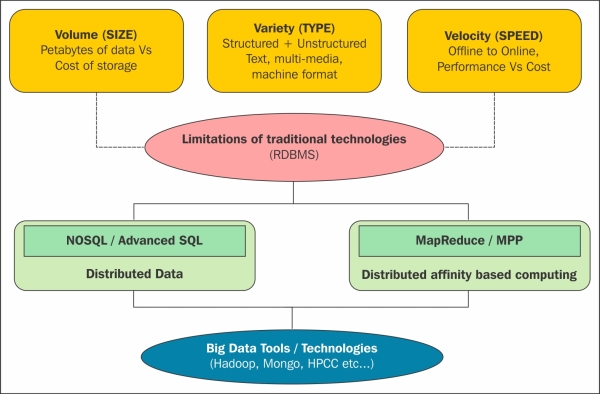
Volume
According to the fifth annual survey by International Data Corporation (IDC), 1.8 zettabytes (1.8 trillion gigabytes) of information were created and replicated in 2011 alone, which is up from 800 GB in 2009, and the number is expected to more than double every two years surpassing 35 zettabytes by 2020. Big data systems are designed to store these amounts of data and even beyond that too with a fault tolerant architecture, and as it is distributed and replicated across multiple nodes, the underlying nodes can be average computing systems, which too need not be high performing systems, which reduces the cost drastically.
The cost per terabyte storage in big data is very less than in other systems, and this has made organizations interested to a greater extent, and even if the data grows multiple times, it is easily scalable, and nodes can be added without much maintenance effort.
Velocity
Processing and analyzing the amount of data that we discussed earlier is one of the key interest areas where big data is gaining popularity and has grown enormously. Not all data to be processed has to be larger in volume initially, but as we process and execute some complex algorithms, the data can grow massively. For processing most of the algorithms, we would require intermediate or temporary data, which can be in GB or TB for big data, so while processing, we would require some significant amount of data, and processing also has to be faster. Big data systems can process huge complex algorithms on huge data much quickly, as it leverages parallel processing across distributed environment, which executes multiple processes in parallel at the same time, and the job can be completed much faster.
For example, Yahoo created a world record in 2009 using Apache Hadoop for sorting a petabyte in 16.25 hours and a terabyte in 62 seconds. MapR have achieved terabyte data sorting in 55 seconds, which speaks volume for the processing power, especially in analytics where we need to use a lot of intermediate data to perform heavy time and memory intensive algorithms much faster.
Variety
Another big challenge for the traditional systems is to handle different variety of semi-structured data or unstructured data such as e-mails, audio and video analysis, image analysis, social media, gene, geospatial, 3D data, and so on. Big data can not only help store, but also utilize and process such data using algorithms much more quickly and also efficiently. Semi-structured and unstructured data processing is complex, and big data can use the data with minimal or no preprocessing like other systems and can save a lot of effort and help minimize loss of data.