Distributed computing
For the remainder of this book, we will adopt the following working definition of distributed computing:
Distributed computing is the simultaneous use of more than one computer to solve a problem.
Typically, as in the case of parallel computing, this definition is oftentimes further restricted. The restriction usually is the requirement that these computers appear to their users as a single machine, therefore hiding the distributed nature of the application. In this book, we will be happy with the more general definition.
Distributing computation across multiple computers is again a pretty obvious strategy when using systems that are able to speak to each other over the (local or otherwise) network. In many respects, in fact, this is just a generalization of the concepts of parallel computing that we saw in the previous section.
Reasons to build distributed systems abound. Oftentimes, the reason is the ability to tackle a problem so big that no individual computer could handle it at all, or at least, not in a reasonable amount of time. An interesting example from a field that is probably familiar to most of us is the rendering of 3D animation movies, such as those from Pixar and DreamWorks.
Given the sheer number of frames to render for a full-length feature (30 frames per second on a two-hour movie is a lot!), movie studios need to spread the full-rendering job to large numbers of computers (computer farms as they are called).
Other times, the very nature of the application being developed requires a distributed system. This is the case, for instance, for instant messaging and video conferencing applications. For these pieces of software, performance is not the main driver. It is just that the problem that the application solves is itself distributed.
In the following figure, we see a very common web application architecture (another example of a distributed application), where multiple users connect to the website over the network. At the same time, the application itself communicates with systems (such as a database server) running on different machines in its LAN:
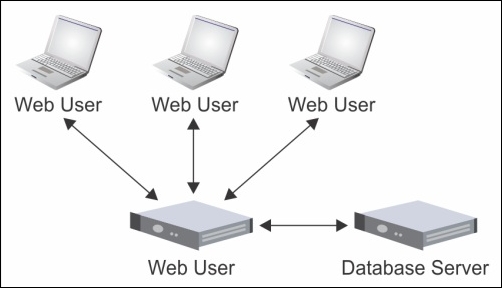
Another interesting example of distributed systems, which might be a bit counterintuitive, is the CPU-GPU combination. These days, graphics cards are very sophisticated computers in their own right. They are highly parallel and offer compelling performance for a large number of compute-intensive problems, not just for displaying images on screen. Tools and libraries exist to allow programmers to make use of GPUs for general-purpose computing (for example CUDA from NVIDIA, OpenCL, and OpenAcc among others).
However, the system composed by the CPU and GPU is really an example of a distributed system, where the network is replaced by the PCI bus. Any application exploiting both the CPU and the GPU needs to take care of data movement between the two subsystems just like a more traditional application running across the network!
It is worth noting that, in general, adapting the existing code to run across computers on a network (or on the GPU) is far from a simple exercise. In these cases, I find it quite helpful to go through the intermediate step of using multiple processes on a single computer first (refer to the discussion in the previous section). Python, as we will see in Chapter 3, Parallelism in Python, has powerful facilities for doing just that (refer to the concurrent.futures
module).
Once I evolve my application so that it uses multiple processes to perform operations in parallel, I start thinking about how to turn these processes into separate applications altogether, which are no longer part of my monolithic core.
Special attention must be given to the data—where to store it and how to access it. In simple cases, a shared filesystem (for example, NFS on Unix systems) is enough; other times, a database and/or a message bus is needed. We will see some concrete examples from Chapter 4, Distributed Applications – with Celery, onwards. It is important to remember that, more often than not, data, rather than CPU, is the real bottleneck.