Sequence-to-sequence networks for natural language processing
Rule-based systems are being replaced by end-to-end neural networks because of their increase in performance.
An end-to-end neural network means the network directly infers all possible rules by example, without knowing the underlying rules, such as syntax and conjugation; the words (or the characters) are directly fed into the network as input. The same is true for the output format, which can be directly the word indexes themselves. The architecture of the network takes care of learning the rules with its coefficients.
The architecture of choice for such end-to-end encoding-decoding networks applied to Natural Language Processing (NLP), is the sequence-to-sequence network, displayed in the following figure:
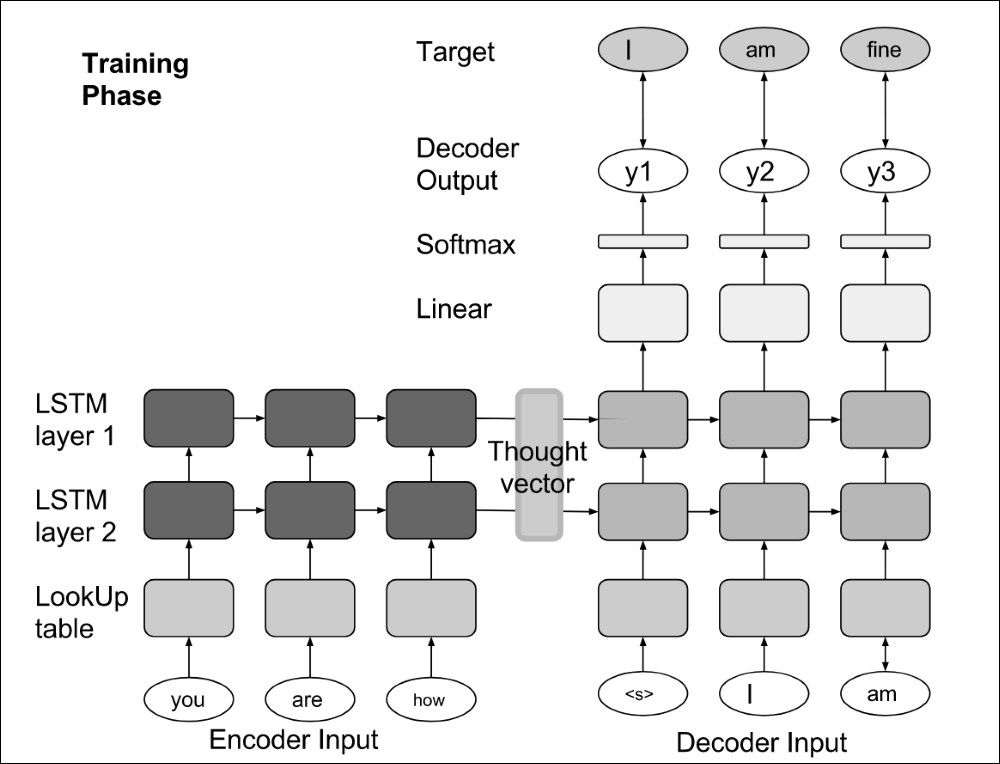
Word indexes are converted into their continuous multi-dimensional values in the embedded space with a lookup table. This conversion, presented in Chapter 3, Encoding Word into Vector is a crucial step to encode the discrete...