SVM or Support Vector Machine
This is another popular algorithm that is used in many real life applications like text categorization, image classification, sentiment analysis and handwritten digit recognition. Support vector machine algorithm can be used both for classification as well as for regression. Spark has the implementation for linear SVM which is a binary classifier. If the datapoints are plotted on a chart the SVM algorithm creates a hyperplane between the datapoints. The algorithm finds the closest points with different labels within the dataset and it plots the hyperplane between those points. The location of the hyperplane is such that it is at maximum distance from these closest points, this way the hyperplane would nicely bifurcate the data. To figure out this maximum distance for the location of the hyperplane the SVM algorithm uses a kernel
function (mathematical function).
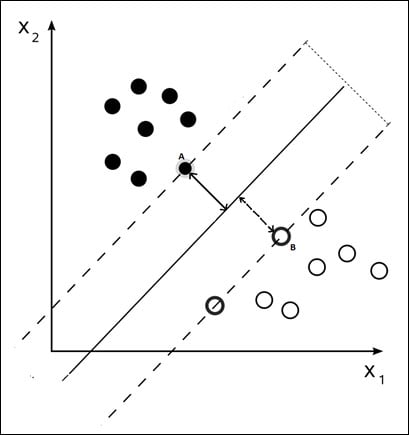
As you can see in the image we have two different type of datapoints one clustered on the X2 axis...