diarizationThe predictive/prescriptive AI life cycle of a device starts with data collection design. Data is analyzed for factors such as correlation and variance. Then the devices start being manufactured. Other than a small number of sample devices, there is usually no device failures, that produce machine learning models. To compensate for this, most manufacturers use duty cycle thresholds to determine whether a device is in a good state or a bad state. These duty cycle standards may be that that the device is running too hot or an arbitrary value is put on a sensor for an alert. But the data quickly needs more advanced analysis. The sheer volume of data can be daunting for an individual. The analyst needs to look through millions of records to find the proverbial needle in a haystack. Using an analyst-in-the-middle approach using anomaly detection can...




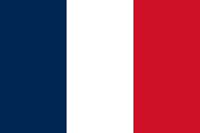


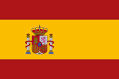



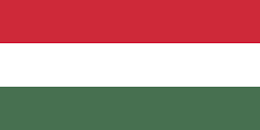

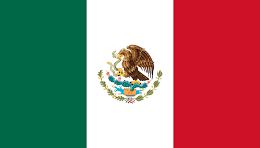


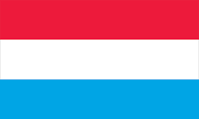



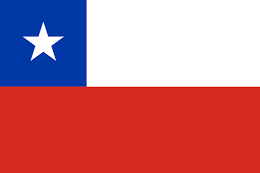


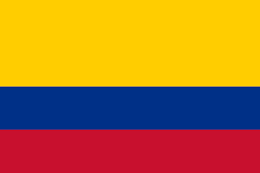
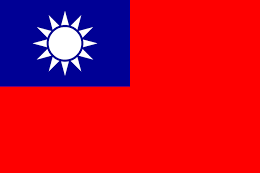


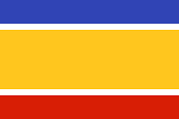
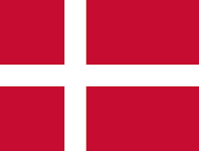
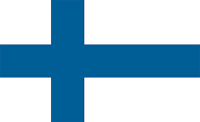



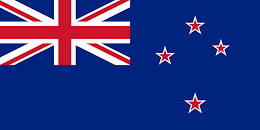





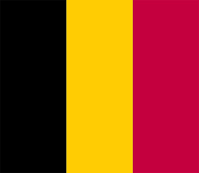

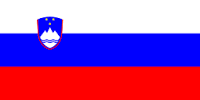




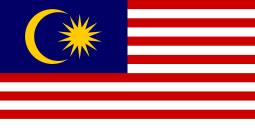


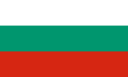


