



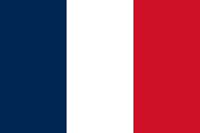


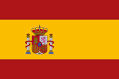



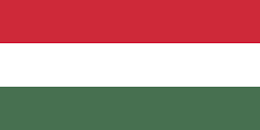

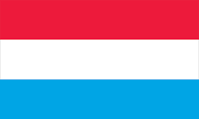





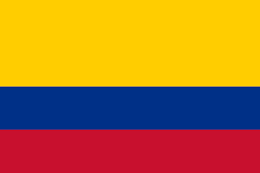
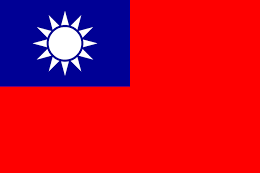
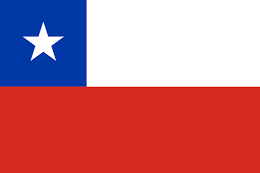



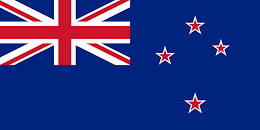
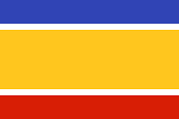
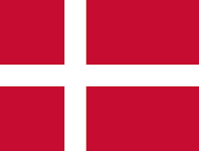
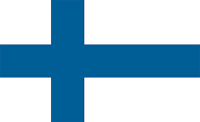







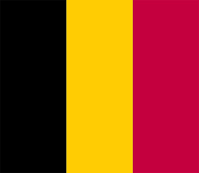

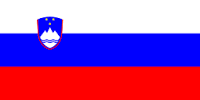





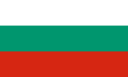


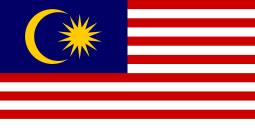



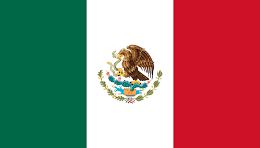

By AI Trends Staff
Data governance in data-driven organizations is a set of practices and guidelines that define where responsibility for data quality lives. The guidelines support the operation’s business model, especially if AI and machine learning applications are at work.
Data governance is an operations issue, existing between strategy and the daily management of operations, suggests a recent account in the MIT Sloan Management Review.
“Data governance should be a bridge that translates a strategic vision acknowledging the importance of data for the organization and codifying it into practices and guidelines that support operations, ensuring that products and services are delivered to customers,” stated author Gregory Vial is an assistant professor of IT at HEC Montréal.
To prevent data governance from being limited to a plan that nobody reads, “governing” data needs to be a verb and not a noun phrase as in “data governance.” Vial writes, “The difference is subtle but ties back to placing governance between strategy and operations — because these activities bridge and evolve in step with both.”
An overall framework for data governance was proposed by Vijay Khatri and Carol V. Brown in a piece in Communications of the ACM published in 2010. The two suggested the strategy is based on five dimensions that represent a combination of structural, operational and relational mechanisms. The five dimensions are:
“Governing data is not easy, but it is well worth the effort,” stated Vial. “Not only does it help an organization keep up with the changing legal and ethical landscape of data production and use; it also helps safeguard a precious strategic asset while supporting digital innovation.”
Master Data Management Seen as a Path to Clean Data Governance
Once the organization commits to data quality, what’s the best way to get there? Naturally entrepreneurs are in position to step forward with suggestions. Some of them are around master data management (MDM), a discipline where business and IT work together to ensure the accuracy and consistency of the enterprise’s master data assets.
Organizations starting down the path with AI and machine learning may be tempted to clean the data that feeds a specific application project, a costly approach in the long run suggests one expert.
“A better, more sustainable way is to continuously cure the data quality issues by using a capable data management technology. This will result in your training data sets becoming rationalized production data with the same master data foundation,” suggests Bill
O’Kane, author of a recent account from tdwi.org on master data management. Formerly an analyst with Gartner, O’Kane is now the VP and MDM strategist at Profisee, a firm offering an MDM solution.
If the data feeding into the AI system is not unique, accurate, consistent and time, the models will not produce reliable results and are likely to lead to unwanted business outcomes. These could include different decisions being made on two customer records thought to represent different people, but in fact describe the same person. Or, recommending a product to a customer that was previously returned or generated a complaint.
Perceptilabs Tries to Get in the Head of the Machine Learning Scientist
Getting inside the head of a machine learning scientist might be helpful in understanding how a highly trained expert builds and trains complex mathematical models. “This is a complex time-consuming process, involving thousands of lines of code,” writes Martin Isaksson, co-founder and CEO of Perceptilabs, in a recent account in VentureBeat. Perceptilabs offers a product to help automation the building of machine learning models, what it calls a “GUI for TensorFlow.”.
“As AI and ML took hold and the experience levels of AI practitioners diversified, efforts to democratize ML materialized into a rich set of open source frameworks like TensorFlow and datasets. Advanced knowledge is still required for many of these offerings, and experts are still relied upon to code end-to-end ML solutions,” Isaksson wrote..
AutoML tools have emerged to help adjust parameters and train machine learning models so that they are deployable. Perceptilabs is adding a visual modeler to the mix.
The company designed its tool as a visual API on top of TensorFlow, which it acknowledges as the most popular ML framework. The approach gives developers access to the low-level TensorFlow API and the ability to pull in other Python modules. It also gives users transparency into how the model is architected and a view into how it performs.
Read the source articles in the MIT Sloan Management Review, Communications of the ACM, tdwi.org and VentureBeat.