Using regular expressions and GREL to clean up data
When cleaning up and preparing data for use, we sometimes need to extract some information from text fields. Occasionally, we can just split the text fields using delimiters. However, when a pattern of data does not allow us to simply split the text, we need to revert to regular expressions.
Getting ready
To follow this recipe, you need to have OpenRefine and virtually any Internet browser installed on your computer.
We assume that you followed the previous recipes and your data is already loaded to OpenRefine and the data types are now representative of what the columns hold. No other prerequisites are required.
How to do it…
First, let's have a look at the pattern that occurs in our city_state_zip column. As the name suggests, we can expect the first element to be the city followed by state and then a 5-digit ZIP code. We could just split the text field using a space character as a delimiter and be done with it. It would work for many records (for example, Sacramento) and they would be parsed properly into city, state, and ZIP. There is one problem with this approach—some locations consist of two or three words (for example, Elk Grove). Hence, we need a slightly different approach to extract such information.
This is where regular expressions play an invaluable role. You can use regular expressions in OpenRefine to transform the data. We will now split city_state_zip into three columns: city, state, and zip. Click on the downward button next to the name of the column and, from the menu, select Edit column and Add column based on this column. A window should appear, as shown in the following screenshot:
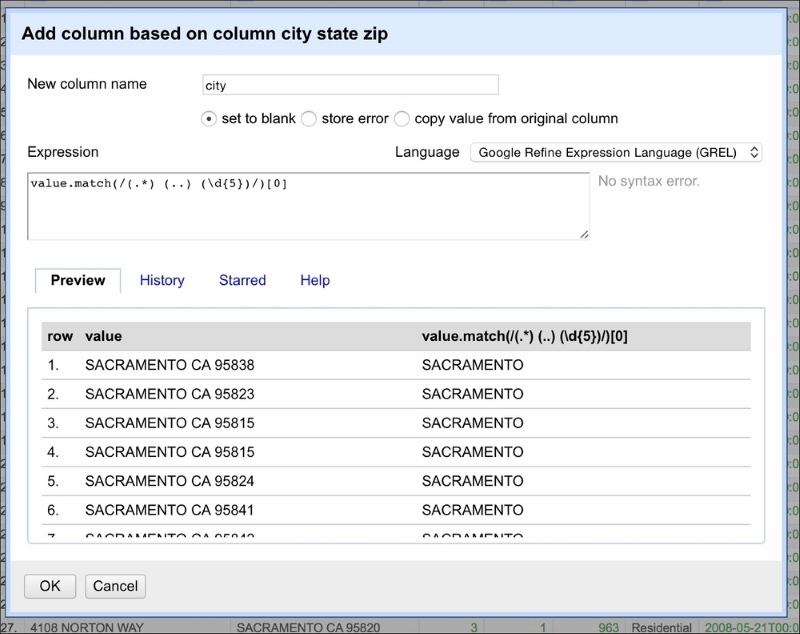
As before, the value represents the value of each cell. The .match(...)
method is applied to the cell's value. It takes a regular expression as its parameter and returns a list of values matched given the expressed pattern. The regular expression is encapsulated between /.../
. Let's break the regular expression down step by step.
We know the pattern of the city_state_zip column: first is the name of the city (can be more than one word), followed by a two-character state acronym, and ending with a 5-digit ZIP code. The regular expression to match such a pattern will be as follows:
(.*) (..) (\d{5})
It is easier to read this expression starting from the end. So, reading from the right, first we extract the ZIP code using (\d{5})
. The \d
indicates any digit (and is equivalent to stating ([0-9]{5})
) and {5}
selects five digits from the back of the string. Next, we have (..)¬
. This expression extracts the two-character acronym of the state identified by two dots (..)
. Note that we used ¬
in place of a space character just for readability purposes. This expression extracts only two characters and a space from the string—no less, no more. The last (reading from the right) is (.*)
that can be understood as: extract all the characters (if any) that will not be matched by the other two expressions.
In entirety, the expression can be translated into English as follows: extract a string (even if empty) until a two-character acronym of the state is encountered (preceded by a space character) followed by a space and five digits indicating the ZIP code.
The .match(...)
method generates a list. In our case, we will get back a list of three elements. To extract city, we select the first element from that list [0]
. To select state and ZIP, we will repeat the same steps but select [1]
and [2]
respectively.
Now that we're done with splitting the city_state_zip column, we can export the project to a file. In the top right corner of the tool, you will find the Export button; select Comma-separated value. This will download the file automatically to your Downloads
folder.
See also
I highly recommend reading the Mastering Python Regular Expressions book by Felix Lopez and Victor Romero available at https://www.packtpub.com/application-development/mastering-python-regular-expressions.