Applying schemas to data ingestion
The application of schemas is common practice when ingesting data, and PySpark natively supports applying them to DataFrames. To define and apply schemas to our DataFrames, we need to understand some concepts of Spark.
This recipe introduces the basic concept of working with schemas using PySpark and its best practices so that we can later apply them to structured and unstructured data.
Getting ready
Make sure PySpark is installed and working on your machine for this recipe. You can run the following code on your command line to check this requirement:
$ pyspark --version
You should see the following output:
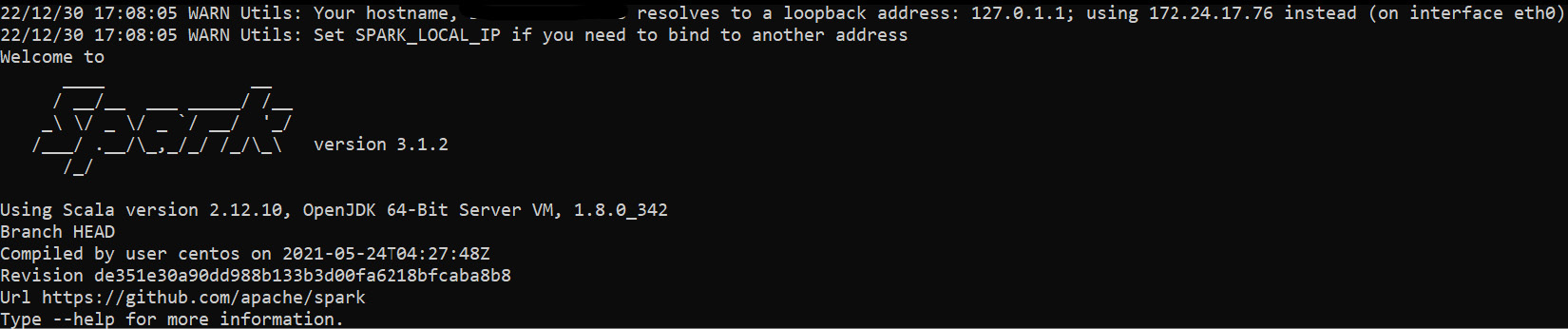
Figure 6.1 – PySpark version console output
If don’t have PySpark installed on your local machine, please refer to the Installing PySpark recipe in Chapter 1.
I will use Jupyter Notebook to execute the code to make it more interactive. You can use this link and follow the instructions...