




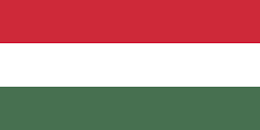

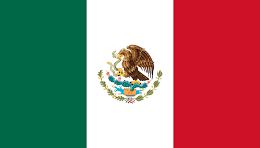


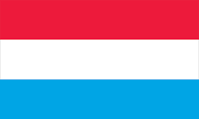



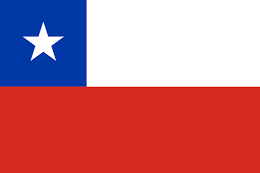



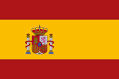


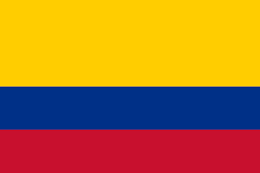
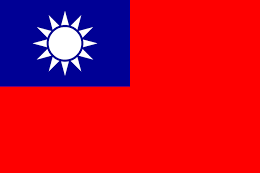


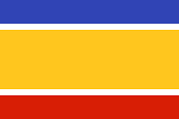
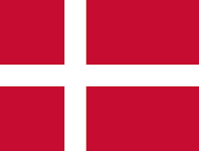
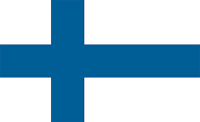



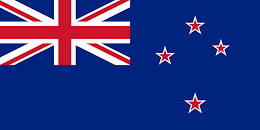


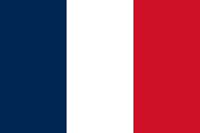



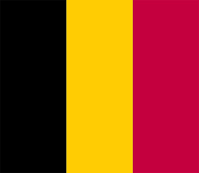

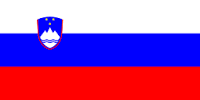




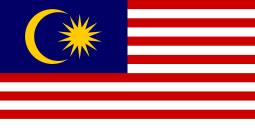


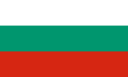




Google and Harvard have teamed up to find out a way to predict locations where the earthquake aftershocks might occur, with the help of deep learning. Currently, it is possible to only predict the timing and size of aftershocks with the help of empirical laws such as, Bäth's Law and Omori's Law. However, forecasting where these events will occur is more challenging.
The researchers at Google, with Brendan Meade, a professor of Earth and Planetary Sciences at Harvard University, and Phoebe DeVries, a post-doctoral fellow working in his lab, are using machine learning to try finding a way to forecast the location where the aftershock occurs. DeVries believes that forecasting aftershock is a well-suited problem for machine learning to solve:
"I'm very excited for the potential for machine learning going forward with these kind of problems -- it's a very important problem to go after. Aftershock forecasting in particular is a challenge that's well-suited to machine learning because there are so many physical phenomena that could influence aftershock behavior and machine learning is extremely good at teasing out those relationships. I think we've really just scratched the surface of what could be done with aftershock forecasting...and that's really exciting."
This model is not absolutely precise, but proved to be significantly more reliable than most of the existing models like, Coulomb failure stress change. In terms of accuracy the deep learning model was able to hit 0.849, on an accuracy scale of 0 to 1. They have also published a paper documenting their findings.
The model is far from ready to deploy in the real-world, but has definitely given a motivation to the researchers to investigate the relevance of deep learning in mitigating earthquake aftershocks.
To know more on how Google and Harvard teamed up to solve the problem of earthquake aftershocks using deep learning check out Google’s blog post.
AutoAugment: Google’s research initiative to improve deep learning performance
Deep Learning in games – Neural Networks set to design virtual worlds
Google strides forward in deep learning: open sources Google Lucid to answer how neural networks make decisions