



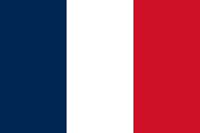


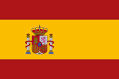



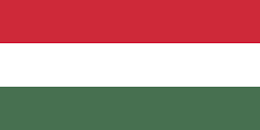

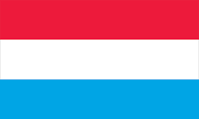





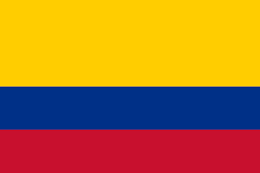
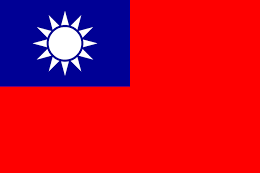
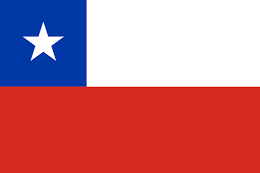



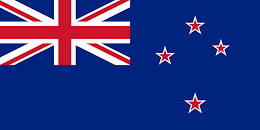
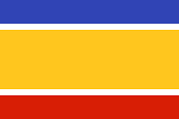
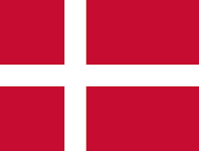
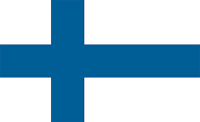







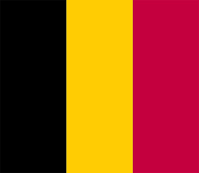

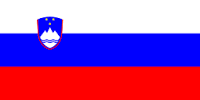





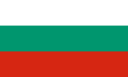


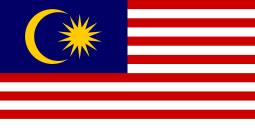



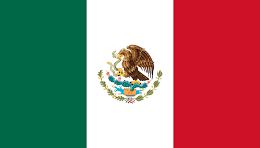

While many of the newer self-service BI offerings are a progressive step forward, they still have some limitations that are not so easily overcome. One of the biggest advantages of self-service business intelligence is the ability for smaller and previously much more limited revenue and smaller sized companies to utilize their data and various other software-as-a-service offerings that previously would have been restricted to enterprise companies needing in house developers and data architects. This alone is an incredible barrier to overcome and helps in lessening the gap and burden that traditional small and medium businesses may have previously faced when hoping to use their collection of data in a real and impactful way.
Self-service BI is the idea that traditional “Business Intelligence” tasks may be automated, and pipelines created in such a way that business insights can be optimized in a way that previously required both enterprise-sized datasets and enterprise-quality engineering to incorporate real and actionable insights. This idea has largely become outdated with the influx of both easily integrate-able third-party services (such as Azure BI tools and IBM Cognos Analytics), and the push towards using a company's collected data at any scale that can be used in supervised or unsupervised learning (with automated model tuning and feature engineering).
One of the limits of self-service business intelligence services is that the abilities of the software are often so broad, that they cannot provide the level of insight that would be helpful or necessary to be applied to increase revenue. While these insights and self-service BI services might be useful for initial, or exploratory visualization purposes, the current implementations cannot provide the expertise and thoroughness that an established data scientist would be able to provide, and cannot divulge into the minutia that a boss may ask of someone with fine tuned statistical knowledge of their models.
Often these insights are limited in ways such as: feature engineering, data warehousing abilities, data pipeline integration, machine learning algorithms available, and a multitude of other aspects that while they are slowly being incorporated into the self-service BI platforms (and as an early Azure ML user, they have become incredibly adept and useful), will always be slightly behind the latest and greatest just by nature of the need to depend on others to implement the newest idea into the platform.
Another limitation of self-service platforms is that you are somewhat locked into these platforms once you create a solution that may be suitable for your needs. Some of the issues with this can be that you are subject to the platform's rising cost, the platform can at any minute change its API format, or the way that data is integrated that would break your system or worse, the self-service BI platform could simply cease to exist if the provider deems that it is no longer something they wish to pursue for a multitude of reasons. While these issues are somewhat avoidable if engineering is done with them in mind (i.e. know your data pipeline and how to translate it to another service or what happens were the third-party service goes down), they are still reasonable issues that could potentially have wide implications for a business depending on how integral they are in the engineering stack. This is probably the most poignant limitation.
All that said, the latest and greatest in BI may be unnecessary for most use cases though, and a general approach that is broad and simple may cover the applicable use cases for most companies, and most big cloud providers of self service BI platforms are unlikely to go down or disappear suddenly without notice. Having hyper-specific pipelines that take months to engineer and integrate optimizations that would have real impacts may far outweigh a simple approach that can be highly adaptable, and creating real insights is one of the best ways a business can start on the path of incorporating machine learning and data science services into their core business.
Graham Annett is an NLP Engineer at Kip (Kipthis.com). He has been interested in deep learning for a bit over a year and has worked with and contributed to Keras (https://github.com/fchollet/keras). He can be found on Github at http://github.com/grahamannett or via http://grahamannett.me