In overfitting, your model fails to generalize. You will determine an overfitting model when it performs well on the training set but poorly on the test set. This typically indicates that the model is too flexible for the amount of training data, and this flexibility allows it to memorize the data, including noise. Overfitting corresponds to high variance, where small changes in the training data result in big changes to the results.
In underfitting, your model fails to capture essential patterns in the training dataset. Typically, underfitting indicates the model is too simple or has too few explanatory variables. An underfitted model is not flexible enough to model real patterns and corresponds to high bias, which indicates the results show a systematic lack of fit in a certain region.
The following graph illustrates the clear difference between overfitting and underfitting as they correspond to a model with good fit:
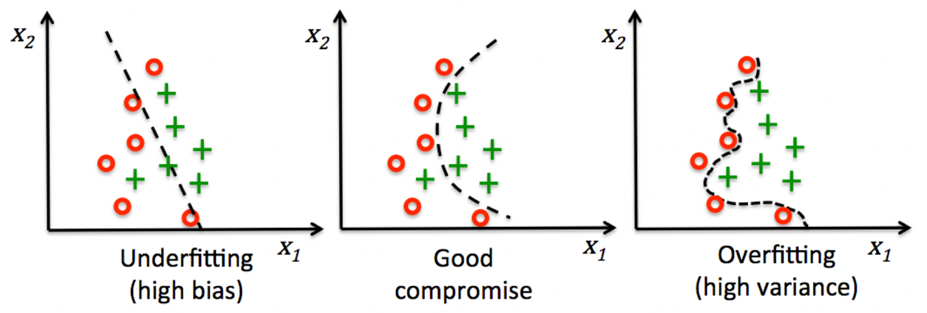