In this chapter, we started with an introduction to a typical machine learning problem, online advertising click-through prediction, and the inherent challenges, including categorical features. We then looked at tree-based algorithms that can take in both numerical and categorical features. We then had an in-depth discussion about the decision tree algorithm: the mechanics, different types, how to construct a tree, and two metrics (Gini Impurity and entropy) that measure the effectiveness of a split at a node. After constructing a tree in an example by hand, we implemented the algorithm from scratch. We also learned how to use the decision tree package from scikit-learn and applied it to predict click-through. We continued to improve the performance by adopting the feature-based random forest bagging algorithm and the chapter ended with some ways to tune a random forest...




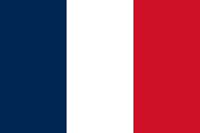


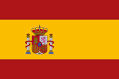




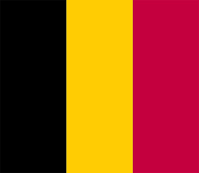
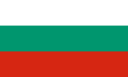
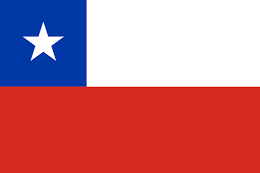
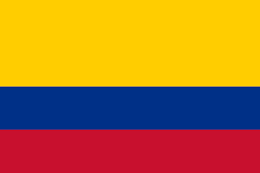
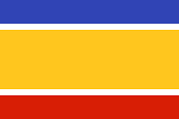

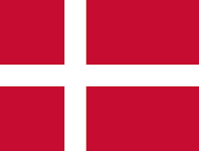



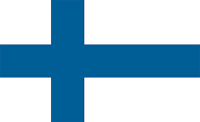

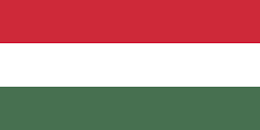






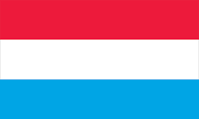
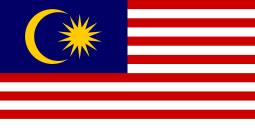

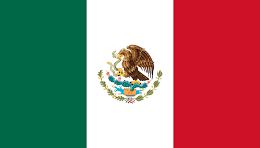

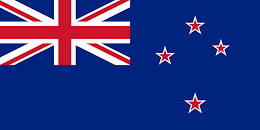







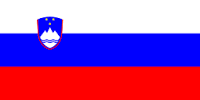




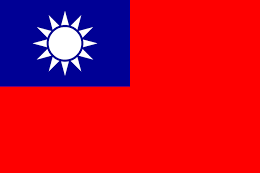


