1.1. Introduction
1.1.1. The rise of NoSQL systems and languages
Managing, querying and making sense of data have become major aspects of our society. In the past 40 years, advances in technology have allowed computer systems to store vast amounts of data. For the better part of this period, relational database management systems (RDBMS) have reigned supreme, almost unchallenged, in their role of sole keepers of our data sets. RDBMS owe their success to several key factors. First, they stand on very solid theoretical foundations, namely the relational algebra introduced by Edgar F. Codd [COD 70], which gave a clear framework to express, in rigorous terms, the limit of systems, their soundness and even their efficiency. Second, RDBMS used one of the most natural representations to model data: tables. Indeed, tables of various sorts have been used since antiquity to represent scales, account ledgers, and so on. Third, a domain-specific language, SQL, was introduced almost immediately to relieve the database user from the burden of low-level programming. Its syntax was designed to be close to natural language, already highlighting an important aspect of data manipulation: people who can best make sense of data are not necessarily computer experts, and vice versa. Finally, in sharp contrast to the high level of data presentation and programming interface, RDBMS have always thrived to offer the best possible performances for a given piece of hardware, while at the same time ensuring consistency of the stored data at all times.
At the turn of the year 2000 with the advances in high speed and mobile networks, and the increase in storage and computing capacity, the amount of data produced by humans became massive, and new usages were discovered that were impractical previously. This increase in both data volumes and computing power gave rise to two distinct but related concepts: “Cloud Computing” and “Big Data”. Broadly speaking, the Cloud Computing paradigm consists of having data processing performed remotely in data centers (which collectively form the so-called cloud) and having end-user devices serve as terminals for information display and input. Data is accessed on demand and continuously updated. The umbrella term “Big Data” characterizes data sets with the so-called three “V”s [LAN 01]: Volume, Variety and Velocity. More precisely, “Big Data” data sets must be large (at least several terabytes), heterogeneous (containing both structured and unstructured textual data, as well as media files), and produced and processed at high speed. The concepts of both Cloud Computing and Big Data intermingle. The sheer size of the data sets requires some form of distribution (at least at the architecture if not at the logical level), preventing it from being stored close to the end-user. Having data stored remotely in a distributed fashion means the only realistic way of extracting information from it is to execute computation close to the data (i.e. remotely) to only retrieve the fraction that is relevant to the end-user. Finally, the ubiquity of literally billions of connected end-points that continuously capture various inputs feed the ever growing data sets.
In this setting, RDBMS, which were the be-all and end-all of data management, could not cope with these new usages. In particular, the so-called ACID (Atomicity, Consistency, Isolation and Durability) properties enjoyed by RDBMS transactions since their inception (IBM Information Management System already supported ACID transactions in 1973) proved too great a burden in the context of massively distributed and frequently updated data sets, and therefore more and more data started to be stored outside of RDBMS, in massively distributed systems. In order to scale, these systems traded the ACID properties for performance. A milestone in this area was the MapReduce paradigm introduced by Google engineers in 2004 [DEA 04]. This programming model consists of decomposing a high-level data operation into two phases, namely the map phase where the data is transformed locally on each node of the distributed system where it resides, and the reduce phase where the outputs of the map phase are exchanged and migrated between nodes according to a partition key – all groups with the same key being migrated to the same (set of) nodes – and where an aggregation of the group is performed. Interestingly, such low-level operations where known both from the functional programming language community (usually under the name map and fold) and from the database community where the map phase can be used to implement selection and projection, and the reduce phase roughly corresponds to aggregation, grouping and ordering.
At the same time as the Big Data systems became prevalent, the so-called CAP theorem was conjectured [BRE 00] and proved [GIL 02]. In a nutshell, this formal result states that no distributed data store can ensure, at the same time, optimal Consistency, Availability and Partition tolerance. In the context of distributed data stores, consistency is the guarantee that a read operation will return the result of the most recent global write to the system (or an error). Availability is the property that every request receives a response that is not an error (however, the answer can be outdated). Finally, partition tolerance is the ability for the system to remain responsive when part of its components are isolated (due to network failures, for instance). In the context of the CAP theorem, the ACID properties enjoyed by RDBMS consist of favoring consistency over availability. With the rise of Big Data and associated applications, new systems emerged that favored availability over consistency. Such systems follow the BASE principles (Basically Available, Soft state and Eventual consistency). The basic tenets of the approach is that operations on the systems (queries as well as updates) must be as fast as possible and therefore no global synchronization between nodes of the system should occur at the time of operation. This, in turn, implies that after an operation, the system may be in an inconsistent state (where several nodes have different views of the global data set). The system is only required to eventually correct this inconsistency (the resolution method is part of the system design and varies from system to system). The wide design space in that central aspect of implementation gave rise to a large number of systems, each having its own programming interface. Such systems are often referred to with the umbrella term Not only SQL (NoSQL). While, generally speaking, NoSQL can also characterize XML databases and Graph databases, these define their own field of research. We therefore focus our study on various kinds of lower-level data stores.
1.1.2. Overview of NoSQL concepts
Before discussing the current trends in research on NoSQL languages and systems, it is important to highlight some of the technical concepts of such systems. In fact, it is their departure from well understood relational traits that fostered new research and development in this area. The aspects which we focus on are mainly centered around computational paradigms and data models.
1.1.2.1. Distributed computations with MapReduce
As explained previously, the MapReduce paradigm consists of decomposing a generic, high-level computation into a sequence of lower-level, distributed, map and reduce operations. Assuming some data elements are distributed over several nodes, the map operation is applied to each element individually, locally on the node where the element resides. When applied to such an element e, the map function may decide to either discard it (by not returning any result) or transform it into a new element e′, to which is associated a grouping key, k, thus returning the pair (k, e′). More generally, given some input, the map operation may output any number of key-value pairs. At the end of the map phase, output pairs are exchanged between nodes so that pairs with the same key are grouped on the same node of the distributed cluster. This phase is commonly referred to as the shuffle phase. Finally, the reduce function is called once for each distinct key value, and takes as input a pair of a key and all the outputs of the map function that were associated with that key. The reduce function can then either discard its input or perform an operation on the set of values to compute a partial result of the transformation (e.g. by aggregating the elements in its input). The result of the reduce function is a pair (k′, r) of an output key k′ and a result r. The results are then returned to the user, sorted according to the k′ key. The user may choose to feed such a result to a new pair of map/reduce functions to perform further computations. The whole MapReduce process is shown in Figure 1.1.
This basic processing can be optimized if the operation computed by the reduce phase is associative and commutative. Indeed, in such a case, it is possible to start the reduce operations on subsets of values present locally on nodes after the map phase, before running the shuffle phase. Such an operation is usually called a combine operation. In some cases, it can drastically improve performance since it reduces the amount of data moved around during the shuffle phase. This optimization works particularly well in practice since the reduce operations are often aggregates which enjoy the commutativity and associativity properties (e.g. sum and average).
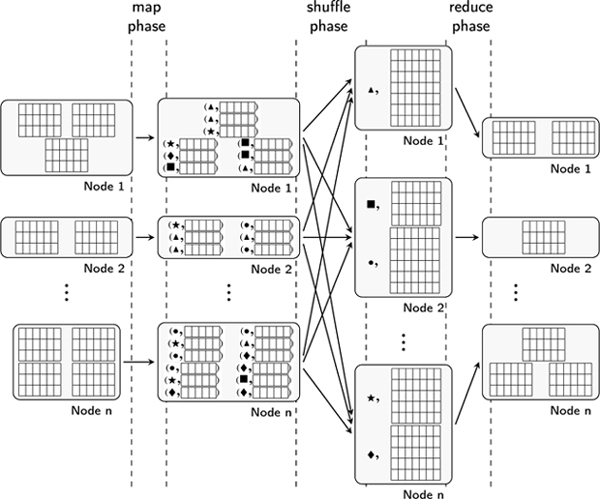
Figure 1.1. MapReduce
The most commonly used MapReduce implementation is certainly the Apache Hadoop framework [WHI 15]. This framework provides a Java API to the programmer, allowing us to express map and reduce transformations as Java methods. The framework heavily relies on the Hadoop Distributed File System (HDFS) as an abstraction for data exchange. The map and reduce transformations just read their input and write their output to the file system, which handle the lower-level aspects of distributing chunks of the files to the components of the clusters, and handle failures of nodes and replication of data.
1.1.2.2. NoSQL databases
A common trait of the most popular NoSQL databases in use is their nature as key-value stores. A key-value store is a database where collections are inherently dictionaries in which each entry is associated with a key that is unique to the collection. While it seems similar to a relational database where tables may have primary keys, key-value stores differ in a fundamental way from relational tables in that they do not rely on – nor enforce – a fixed schema for a given collection. Another striking aspect of all these databases is the relatively small set of operations that is supported natively. Updates are usually performed one element at a time (using the key to denote the element to be added, modified or deleted). Data processing operations generally consist of filtering, aggregation and grouping and exposing a MapReduce-like interface. Interestingly, most NoSQL databases do not support join operations, rather they rely on data denormalization (or materialized joins) to achieve similar results, at the cost of more storage usage and more maintenance effort. Finally, some databases expose a high-level, user-friendly query language (sometimes using an SQL compatible syntax) where queries are translated into combinations of lower-level operations.
1.1.3. Current trends of French research in NoSQL languages
NoSQL database research covers several domains of computer science, from system programming, networking and distributed algorithms, to databases and programming languages. We focus our study on the language aspect of NoSQL systems, and highlight two main trends in French research that pertains to NoSQL languages and systems.
The first trend aims to add support for well-known, relational operations to NoSQL databases. In particular, we survey the extensive body of work that has been done to add support for join operations between collections stored in NoSQL databases. We first describe how join operations are implemented in NoSQL systems (and in particular how joins can be decomposed into sequences of MapReduce operations).
The second trend of research is aimed at unifying NoSQL systems, in particular their query languages. Indeed, current applications routinely interact with several data stores (both relational and NoSQL), using high-level programming languages (PHP, Java, Ruby or JavaScript for Web applications, Python and R for data analytics, etc.). We will survey some of the most advanced work in the area, particularly the definition of common, intermediate query language that can map to various data stores.