Understanding Data for Sentiment Analysis
Sentiment analysis is a type of text classification. Sentiment analysis models are usually trained using supervised datasets. Supervised datasets are a kind of dataset that are labeled with the target variable – usually a column that specifies the sentiment value in the text. This is the value we want to predict in the unseen text.
Exercise 64: Loading Data for Sentiment Analysis
In this exercise, we will load data that could be used to train a sentiment analysis model. For this exercise, we will be using three datasets, namely Amazon, Yelp, and IMDB. Follow these steps to implement this exercise:
- Open a Jupyter notebook.
- Insert a new cell and add the following code to import the necessary libraries:
import pandas as pd pd.set_option('display.max_colwidth', 200)
This imports the
pandas
library. It also sets the display width to200
characters so that more of the review text is displayed on the screen. - Now we need to specify where the sentiment data is located. We will be loading three different datasets from
Yelp
,IMDB
, andAmazon
. Insert a new cell and add the following code to implement this:DATA_DIR = '../data/sentiment_labelled_sentences/' IMDB_DATA_FILE = DATA_DIR + 'imdb_labelled.txt' YELP_DATA_FILE = DATA_DIR + 'yelp_labelled.txt' AMAZON_DATA_FILE = DATA_DIR + 'amazon_cells_labelled.txt' COLUMN_NAMES = ['Review', Sentiment']
Each of the data files has two columns: one for the review text and a numeric column for the sentiment.
Note
All three datasets can be found at this link: https://bit.ly/2VufVIb.
- To load the IMDb reviews, insert a new cell and add the following code:
imdb_reviews = pd.read_table(IMDB_DATA_FILE, names=COLUMN_NAMES)
In this code, the
read_table()
method loads the file into a DataFrame. - Display the top
10
records in the DataFrame. Add the following code in the new cell:imdb_reviews.head(10)
The code generates the following output:
Figure 8.6: The first 10 records in the IMDb movie review file
- In the preceding figure, you can see that the negative reviews have sentiment scores of
0
and positive reviews have sentiment scores of1
. - To check the total records of the IMDB review file, we make use of the
value_counts()
function. Add the following code in a new cell to implement this:imdb_reviews.Sentiment.value_counts()
The expected output with total reviews should be as follows:
Figure 8.7: Total positive and negative reviews in the IMDB review file
In the preceding figure, you can see that the data file contains a total of 748 reviews, out of which 362 are negative and 386 are positive.
- We can format the data by adding the following code in a new cell:
imdb_counts = imdb_reviews.Sentiment.value_counts().to_frame() imdb_counts.index = pd.Series(['Positive', 'Negative']) imdb_counts
The code generates the following output:
Figure 8.8: Counts of positive and negative sentiments in the IMDB review file
- To load the Amazon reviews, insert a new cell and add the following code:
amazon_reviews = pd.read_table(AMAZON_DATA_FILE, name=COLUMN_NAMES) amazon.reviews.head(10)
The code generates the following output:
Figure 8.9: Total positive and negative reviews in the Amazon review file
- To load the Yelp reviews, insert a new cell and add the following code:
yelp_reviews = pd.read_table(YELP_DATA_FILE, names=COLUMN_NAMES) yelp_reviews.head(10)
The code generates the following output:
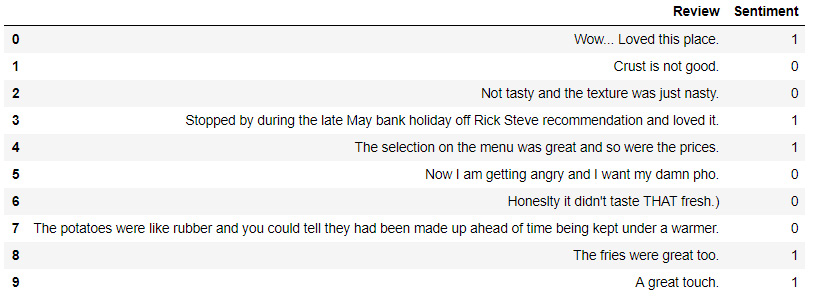
Figure 8.10: Total positive and negative reviews in the YELP review file
We have learned how to load data that could be used to train a sentiment analysis model. The review files mentioned in this exercise are an example of data that could be used to train sentiment models. Each file contains review text plus a sentiment label for each. This is the minimum requirement of a supervised machine learning project: to build a model that is capable of predicting sentiments. However, the review text cannot be used as is. It needs to be preprocessed so that we can extract feature vectors out of it and eventually provide this as an input to the model.
Now that we have learned about loading the data, in the next section, we focus on training sentiment models.