Decision tree learning
Decision tree classifiers are attractive models if we care about interpretability. As the name “decision tree” suggests, we can think of this model as breaking down our data by making a decision based on asking a series of questions.
Let’s consider the following example in which we use a decision tree to decide upon an activity on a particular day:
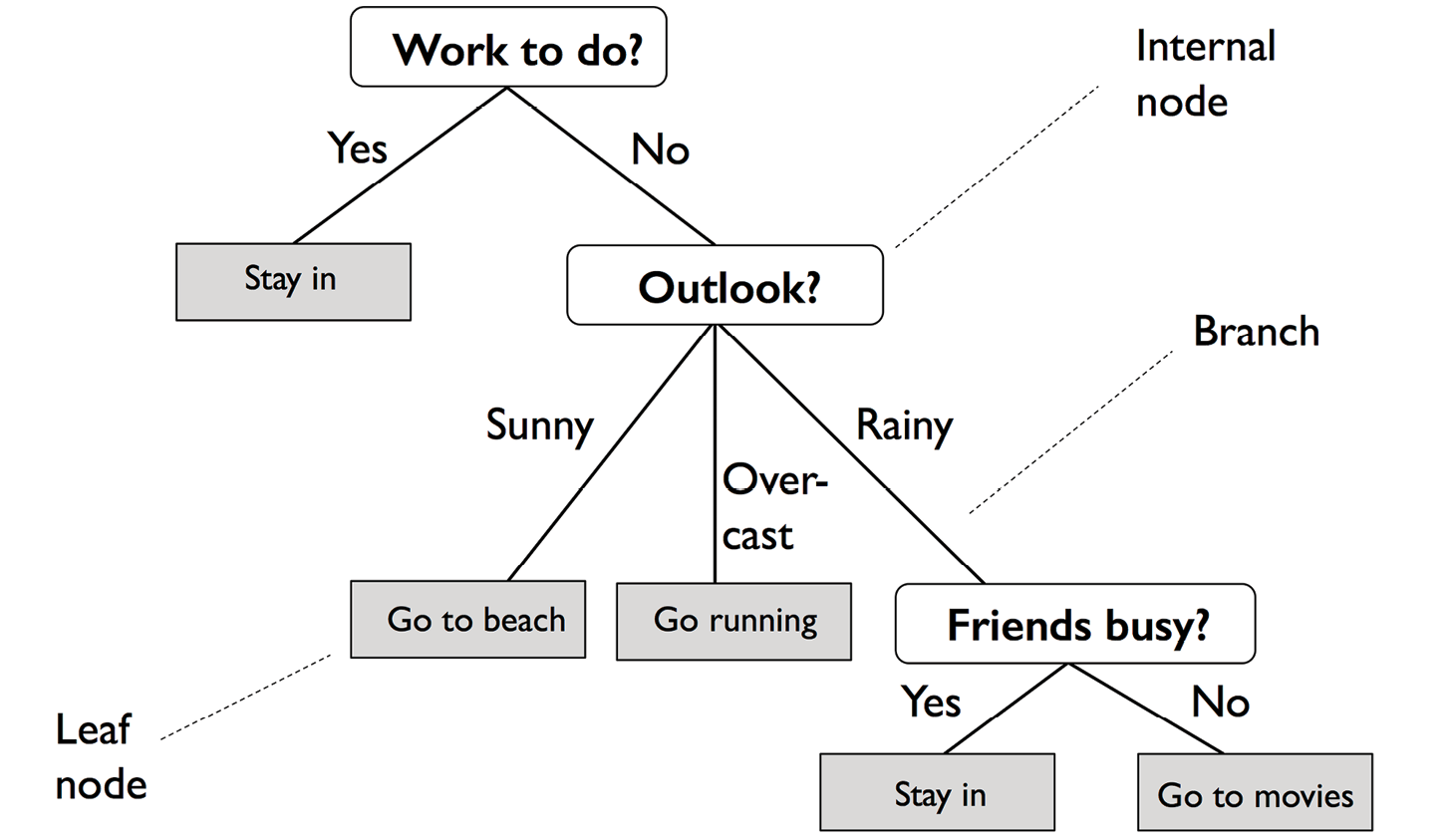
Figure 3.18: An example of a decision tree
Based on the features in our training dataset, the decision tree model learns a series of questions to infer the class labels of the examples. Although Figure 3.18 illustrates the concept of a decision tree based on categorical variables, the same concept applies if our features are real numbers, like in the Iris dataset. For example, we could simply define a cut-off value along the sepal width feature axis and ask a binary question: “Is the sepal width ≥ 2.8 cm?”
Using the decision algorithm, we start...