Managing the model development life cycle
Managing the model life cycle is quite important when working in a team of more than one model developer. It’s quite usual for multiple model developers to try different models within the same project, and having a reviewer decide on the model that ends up going to production is quite important:
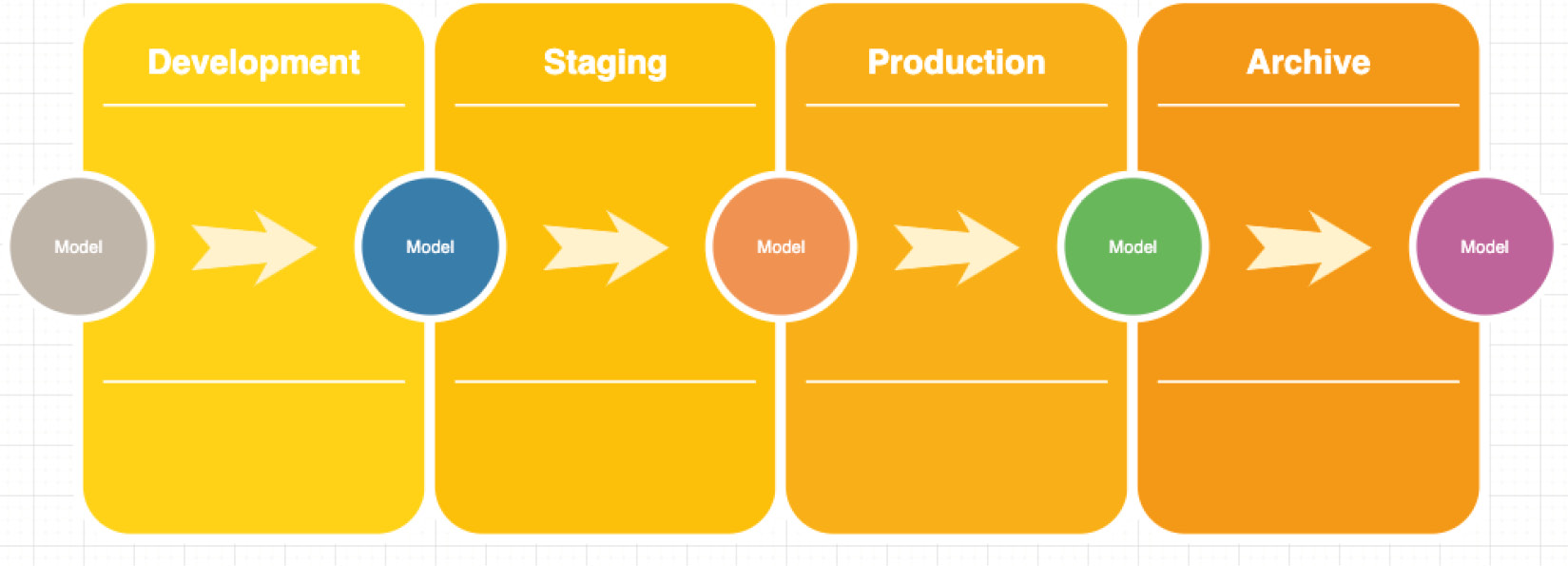
Figure 5.13 – Example of a model development life cycle
A model in its life cycle can undergo the following stages if using a life cycle similar to the one represented in Figure 5.13:
- Development: The state where the model developer is still exploring and trying out different approaches and is still trying to find a reasonable solution to their machine learning problem.
- Staging: The state where the model can be tested automatically with production-type traffic.
- Production: When the model is ready to handle real-life production traffic.
- Archive: When the model no longer serves the business purpose that it was initially developed for, it will be archived and its metadata stored for future reference or compliance.
For instance, a reviewer or supervisor, as represented in Figure 5.14, can move a model from the Development state to Staging for further deployment in a test environment and the model can be transitioned into production if approved by reviewers:
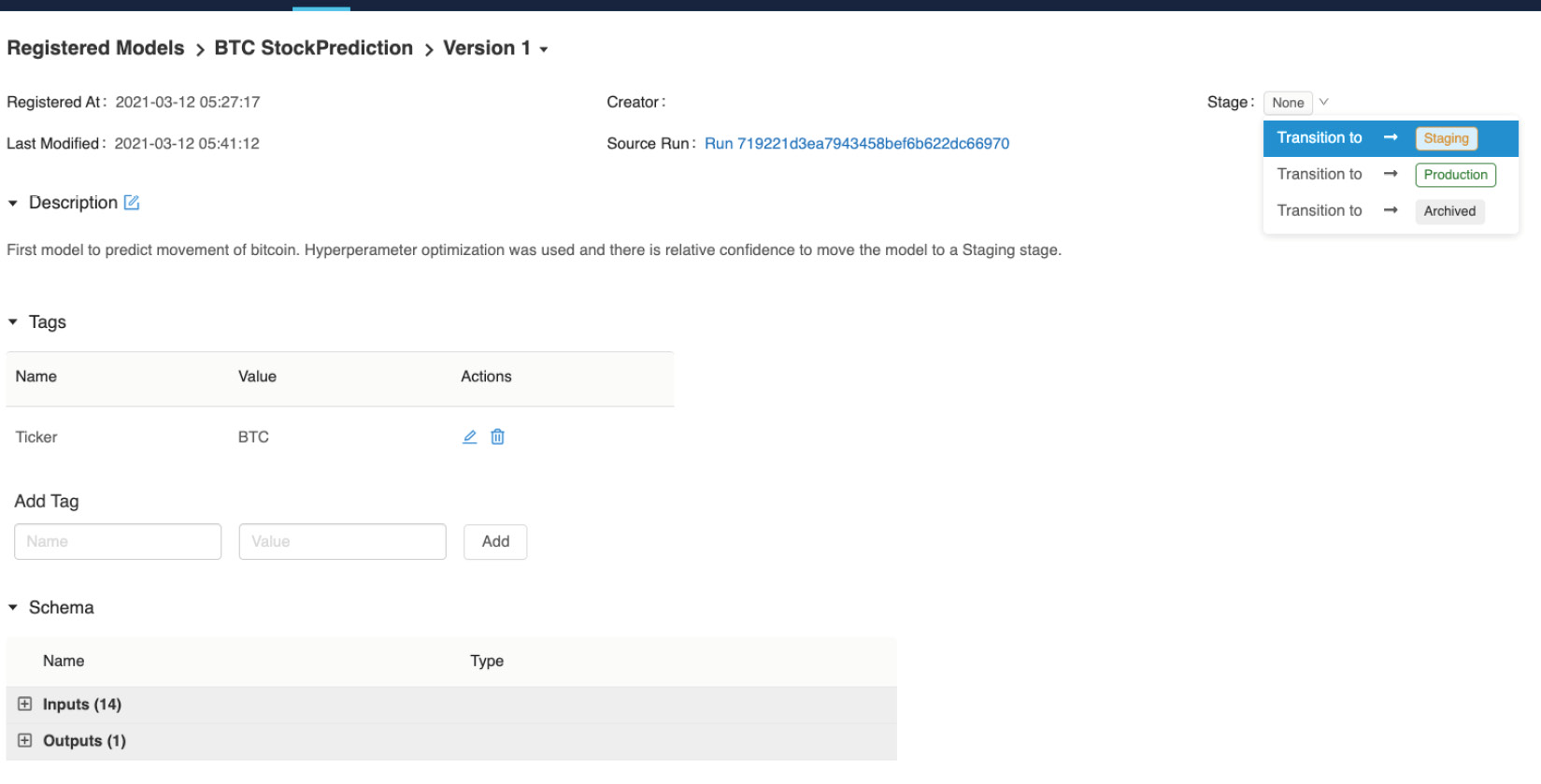
Figure 5.14 – Example of a model development life cycle
When transitioning from a state in MLflow, you have the option to send the model in an existing state to the next state:
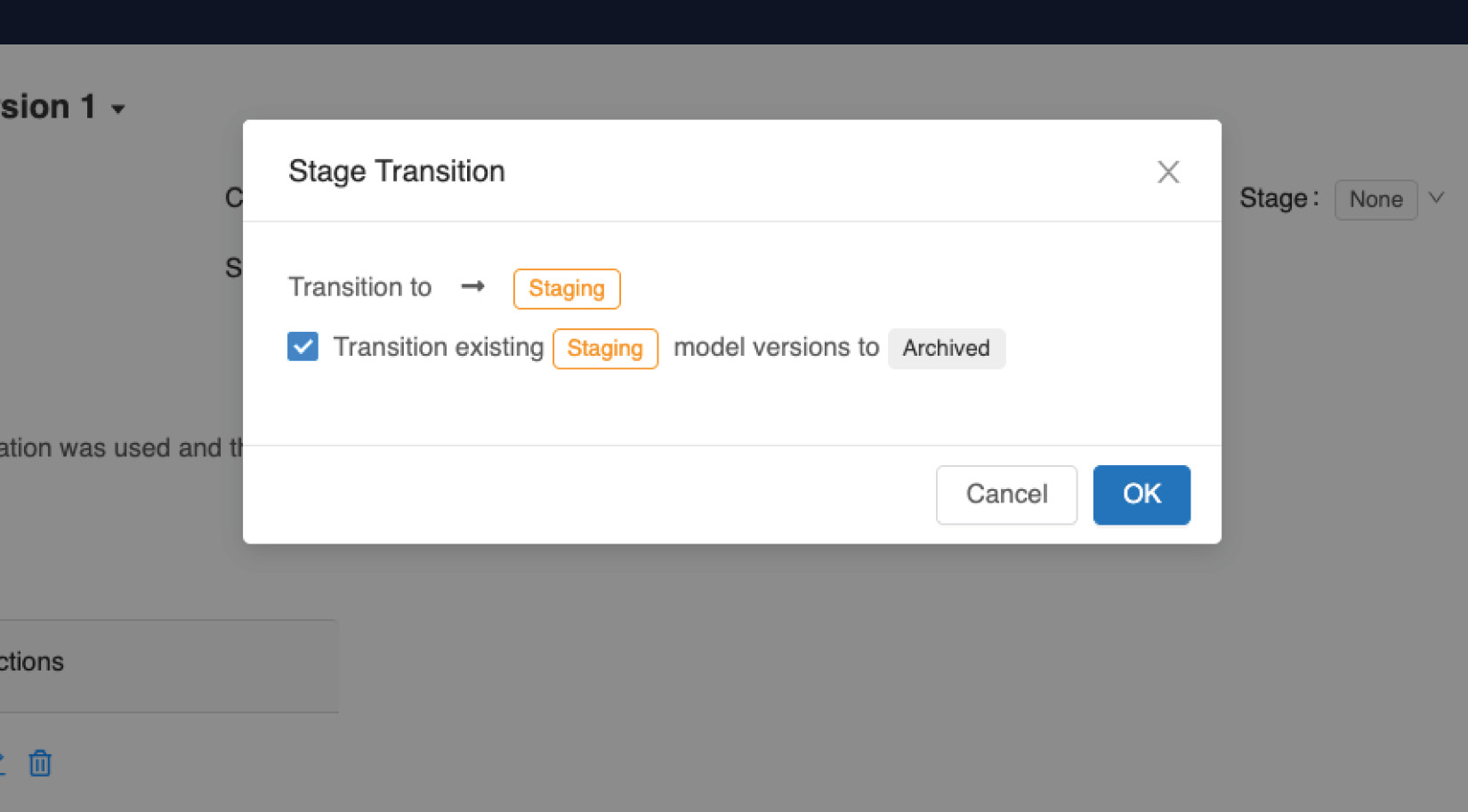
Figure 5.15 – Stage Transition in MLflow
The transitions from the Staging to Production stages in a mature environment are meant to be done automatically, as we will demonstrate in the upcoming chapters of the book.
With this section, we have concluded the description of the features related to models in MLflow.