There are two phases in the training process of a deep neural network: forward and back propagation. We have seen the forward phase in detail:
- Calculate the weighted sum of the inputs:
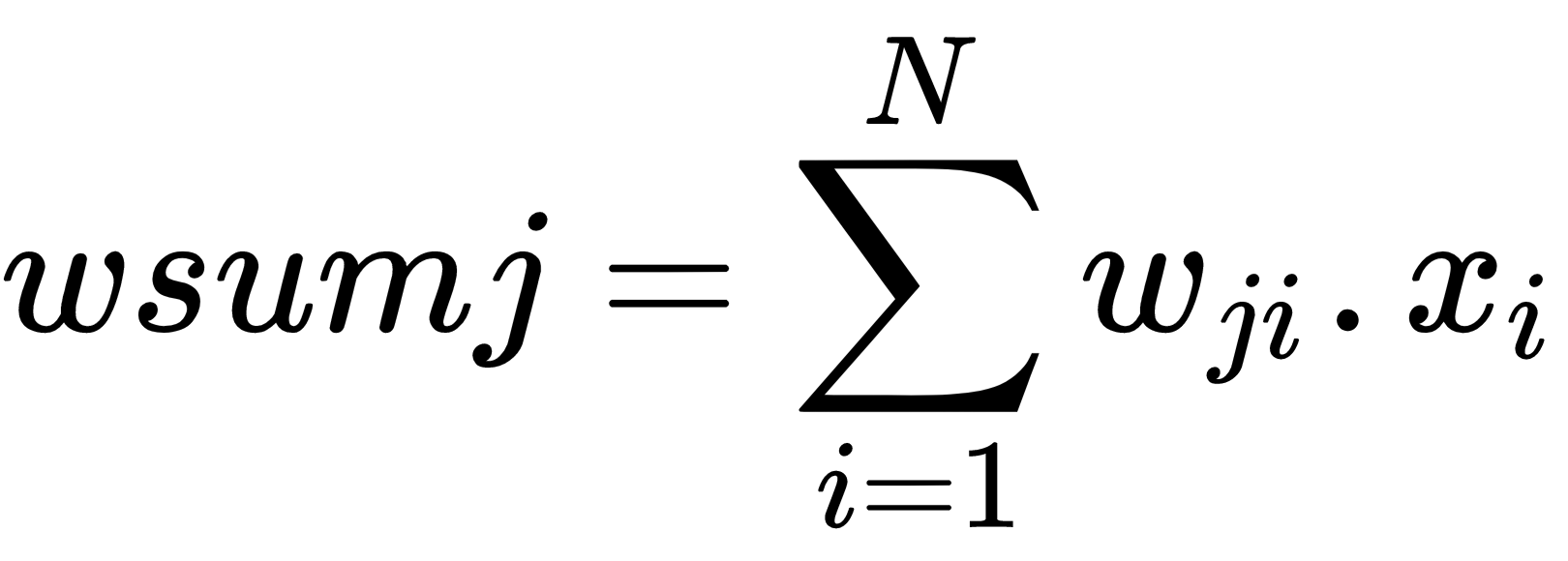
- Apply the activation function to the result:

Find different activation functions in the suggested reading at the end of the chapter. The sigmoid function is the most common and is easier to use, but not the only one.
- Calculate the output by adding all the results from the last layer (N neurons):
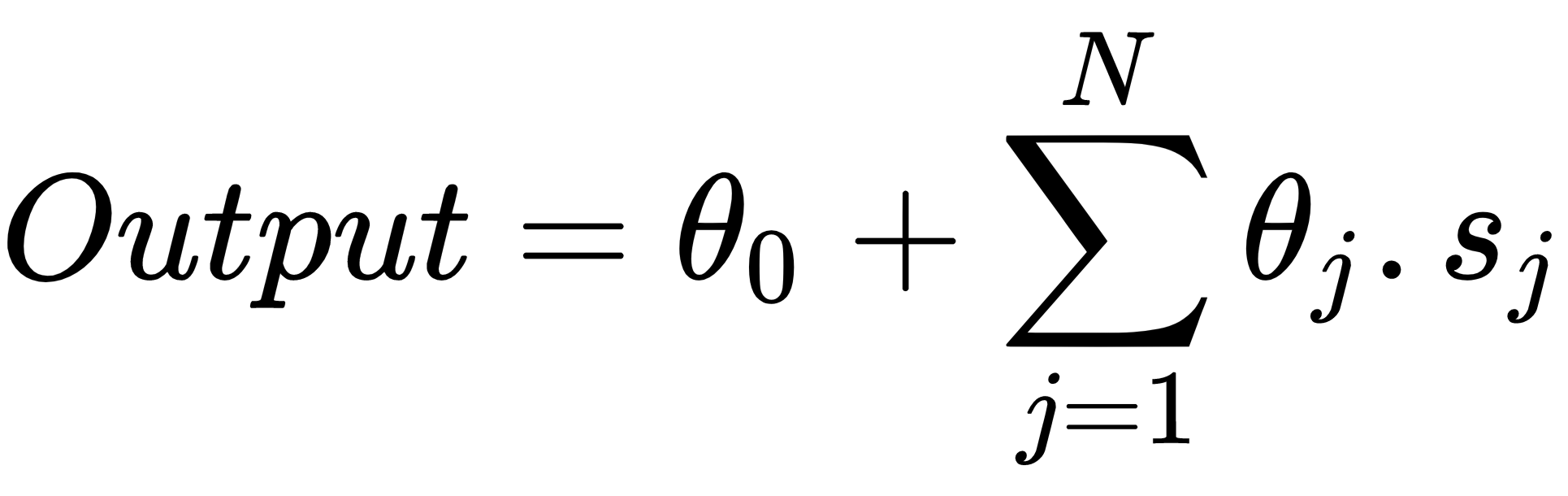
After the forward phase, we calculate the error as the difference between the output and the known target value: Error = (Output-y)2.
All weights are assigned random values at the beginning of the forward phase.
The output, and therefore the error, are functions of the weights wi and θi. This means that we could go backward from the error and see...