



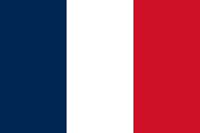


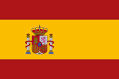



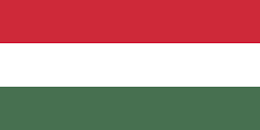

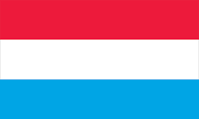





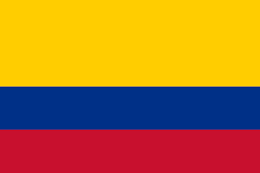
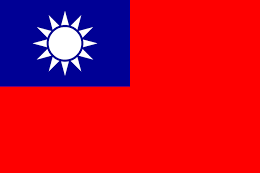
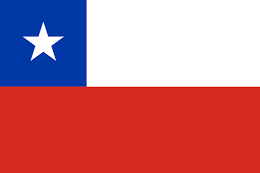



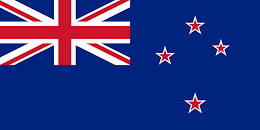
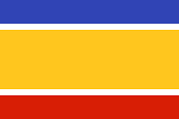
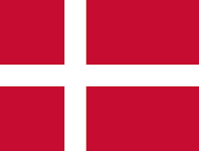
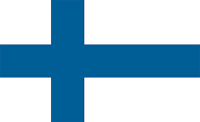







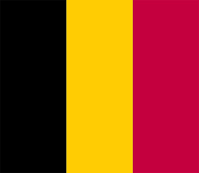

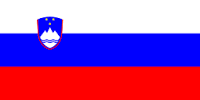





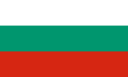


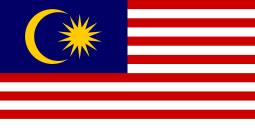



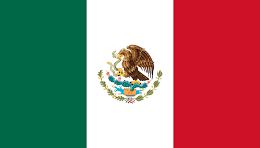

In 2012, a year which feels a lot like the very early years of the era of data, Wired published this article on Narrative Science, an organization based in Chicago that uses Machine Learning algorithms to write news articles. Its founder and CEO, Kris Hammond, is a man whose enthusiasm for algorithmic possibilities is unparalleled. When asked whether an algorithm would win a Pulitzer in the next 20 years he goes further, claiming that it could happen in the next 5 years.
Hammond’s excitement at what his organization is doing is not unwarranted. But his optimism certainly is. Unless 2017 is a particularly poor year for journalism and literary nonfiction, a Pulitzer for one of Narrative Science’s algorithms looks unlikely to say the least.
But there are a couple of problems with Hammond’s enthusiasm. He fails to recognise the limitations of algorithms, the fact that the job of even the most intricate and complex Deep Learning algorithm is very specific is quite literally determined by the people who create it. “We are humanising the machine” he’s quoted as saying in a Guardian interview from June 2015. “Based on general ideas of what is important and a close understanding of who the audience is, we are giving it the tools to know how to tell us stories”. It’s important to notice here how he talks - it’s all about what ‘we’re’ doing. The algorithms that are central to Narrative Science’s mission are things that are created by people, by data scientists. It’s easy to read what’s going on as a simple case of the machines taking over. True, perhaps there is cause for concern among writers when he suggests that in 25 years 90% of news stories will be created by algorithms, but in actual fact there’s just a simple shift in where labour is focused.
We need to rethink how we view and talk about data science, Machine Learning and algorithms. We see, for example, algorithms as impersonal, blandly futuristic things. Although they might be crucial to our personalized online experiences, they are regarded as the hypermodern equivalent of the inauthentic handshake of a door to door salesman. Similarly, at the other end, the process of creating them are viewed as a feat of engineering, maths and statistics nerds tackling the complex interplay of statistics and machinery. Instead, we should think of algorithms as something creative, things that organize and present the world in a specific way, like a well-designed building.
If an algorithm did indeed win a Pulitzer, wouldn’t it really be the team behind it that deserves it?
When Hammond talks, for example, about “general ideas of what is important and a close understanding who the audience is”, he is referring very much to a creative process. Sure, it’s the algorithm that learns this, but it nevertheless requires the insight of a scientist, an analyst to consider these factors, and to consider how their algorithm will interact with the irritating complexity and unpredictability of reality. Machine Learning projects, then, are as much about designing algorithms as they are programming them. There’s a certain architecture, a politics that informs them. It’s all about prioritization and organization, and those two things aren’t just obvious; they’re certainly not things which can be identified and quantified. They are instead things that inform the way we quantify, the way we label. The very real fingerprints of human imagination, and indeed fallibility are in algorithms we experience every single day.
Perhaps we’ve all fallen for Hammond’s enthusiasm. It’s easy to see the algorithms as the key to the future, and forget that really they’re just things that are made by people. Indeed, it might well be that they’re so successful that we forget they’ve been made by anyone - it’s usually only when algorithms don’t work that the human aspect emerges. The data-team have done their job when no one realises they are there.
An obvious example: You can see it when Spotify recommends some bizarre songs that you would never even consider listening to. The problem here isn’t simply a technical one, it’s about how different tracks or artists are tagged and grouped, how they are made to fit within a particular dataset that is the problem. It’s an issue of context - to build a great Machine Learning system you need to be alive to the stories and ideas that permeate within the world in which your algorithm operates - if you, as the data scientist lack this awareness, so will your Machine Learning project.
But there have been more problematic and disturbing incidents such as when Flickr auto tags people of color in pictures as apes, due to the way a visual recognition algorithm has been trained. In this case, the issue is with a lack of sensitivity about the way in which an algorithm may work - the things it might run up against when it’s faced with the messiness of the real-world, with its conflicts, its identities, ideas and stories. The story of Solid Gold Bomb too, is a reminder of the unintended consequences of algorithms. It’s a reminder of the fact that we can be lazy with algorithms; instead of being designed with thought and care they become a surrogate for it - what’s more is that they always give us a get out clause; we can blame the machine if something goes wrong.
If this all sounds like I’m simply down on algorithms, that I’m a technological pessimist, you’re wrong. What I’m trying to say is that it’s humans that are really in control. If an algorithm won a Pulitzer, what would that imply – it would mean the machines have won. It would mean we’re no longer the ones doing the thinking, solving problems, finding new ones.
As the economy becomes reliant on technological innovation, it’s easy to remove ourselves, to underplay the creative thinking that drives what we do. That’s what Hammond’s doing, in his frenzied excitement about his company - he’s forgetting that it’s him and his team that are finding their way through today’s stories. It might be easier to see creativity at work when we cast our eyes towards game development and web design, but data scientists are designers and creators too. We’re often so keen to stress the technical aspects of these sort of roles that we forget this important aspect of the data scientist skillset.