In this chapter, you have learned about various unsupervised learning methods to identify the structures and patterns within the data using k-mean clustering, PCA, SVD and deep auto encoders. Also, the k-means clustering algorithm explained with iris data. Methods were shown on how to choose the optimal k-value based on various performance metrics. Handwritten data from scikit-learn was been utilized to compare the differences between linear methods like PCA and SVD with non-linear techniques and deep auto encoders. The differences between PCA and SVD were given in detail, so that the reader can understand SVD, which can be applied even on rectangular matrices where the number of users and number of products are not necessarily equal. At the end, through visualization, it has been proven that deep auto encoders are better at separating digits than linear unsupervised learning...




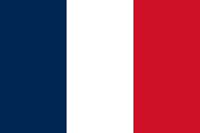


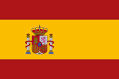



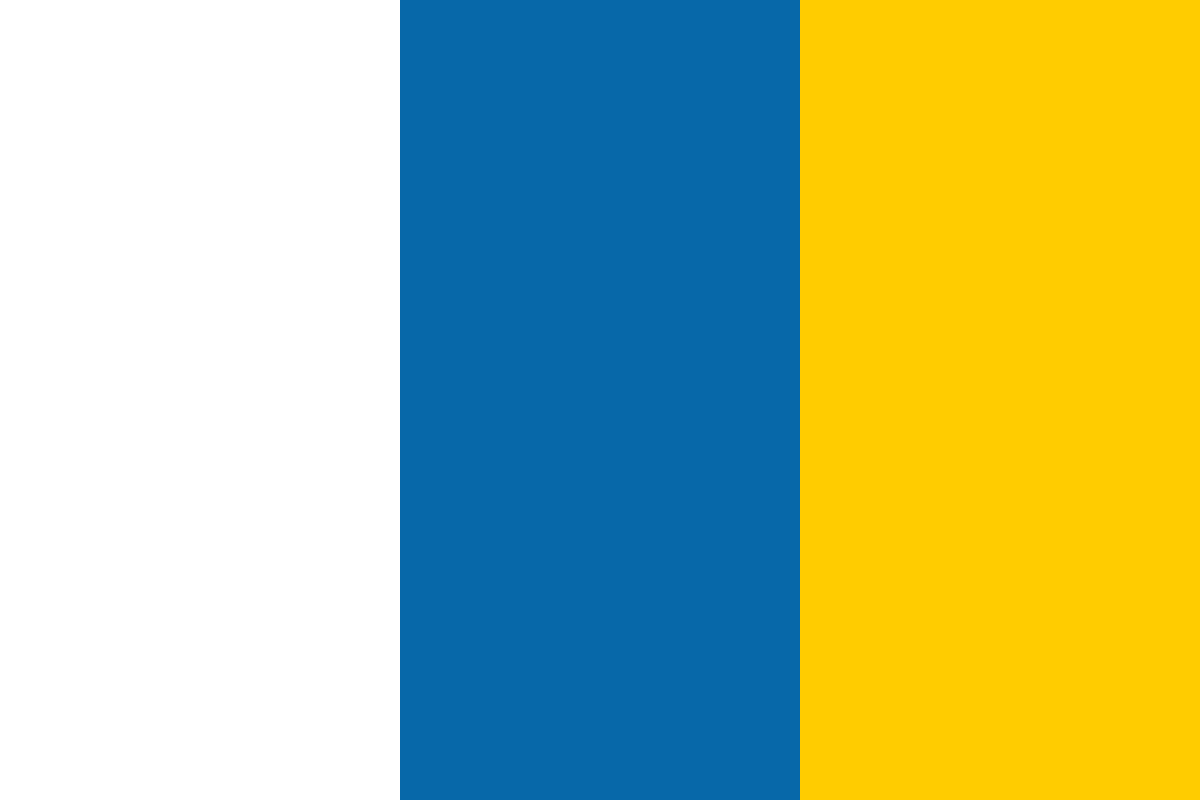
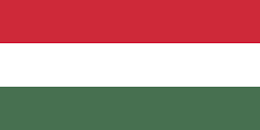

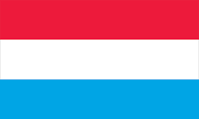





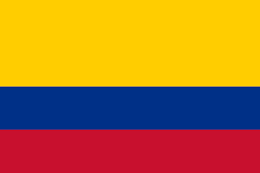
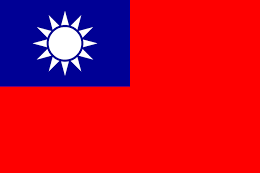
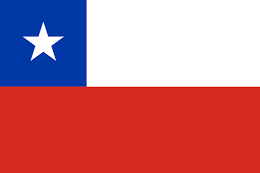



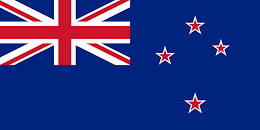
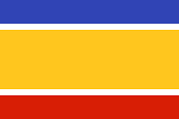
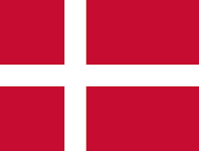
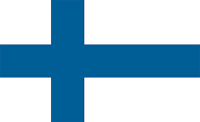







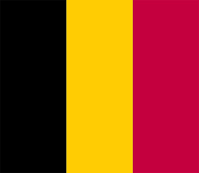

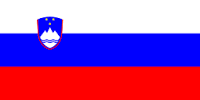





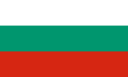


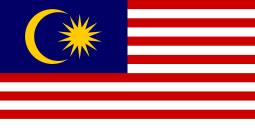



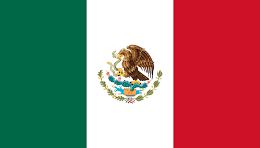
