Impact of bad data quality
In November 2018, a Gartner survey found that “Poor data quality costs organizations an average of $11.8M per year.” The same survey also found that “57% of organizations don’t know what bad data quality is costing them.”
Quantification of the impact of bad data
It is usually incredibly difficult to be this precise when thinking about the monetary impact of data quality issues. When looking at these two quotes together, there is a further curiosity. Presumably, the number of $11.8m per year comes from the 43% of organizations that did calculate what bad data quality was costing them. By implication, then, we do not get from this survey what the organizations who are not measuring this suffer in terms of losses from poor data quality. To quote Donald Rumsfeld from 2002, these organizations are operating with “unknown unknowns.”
Those that do not even measure the impact of poor data quality ironically are likely to have the worst data quality issues – they are completely ignoring the topic. It is like in education – the student who constantly worries about their test results and fears failure is usually more successful in the end than their more relaxed counterparts who rarely (if ever) bother the teacher.
The measurement also lacks sophistication. It would be helpful, for example, to understand how this number changes for large organizations and in different geographies. $11.8m is almost irrelevant for a company with tens of billions of dollars in revenue but is a make-or-break figure for more modestly sized organizations.
The other challenge with this number (which will also be discussed in Chapter 2) is that the dollar cost of data quality issues is inherently difficult to accurately and completely measure. For example, it might be possible to identify the personnel cost of the effort expended while contacting suppliers to collect missing email addresses. However, this is just one data quality issue of an unknown number. Do you really have time to identify the effort being expended on all these manual data correction activities in your company today and quantify them? What about the missed revenue from situations where a customer is impacted by poor data quality and decides not to trade with you again? Do you even know that is why they chose to stop trading with you? The reality is that there is rarely time to get holistic answers to these kinds of questions when working to get a data quality initiative off the ground. At best, illustrative examples are provided to show the known impacts of data quality. This is typically not what senior executives expect and this often means data quality initiatives fail before they can even begin.
In truth, no one knows how much bad data quality costs a company – even companies with mature data quality initiatives in place, who are measuring hundreds of data points for their quality struggle to accurately measure quantitative impact. This is often a deal-breaker for senior leaders when trying to get approval for a budget for data quality work. Data quality initiatives often seek substantial budgets and are up against projects with more tangible benefits.
At an investment board meeting in a previous organization, a project in which I was involved was seeking approval for a data quality initiative. In the same meeting, there was a project seeking approval to implement an e-invoicing solution. This was an online portal for suppliers to log onto and submit invoices electronically against purchase orders and track their payments from the company. This project had a clear business case – it was expected to reduce supplier queries about payments by 50% and allow a reduction in the number of full-time employees in that area. The board was challenging and, in the end, approved the e-invoicing project and rejected our initiative.
Six months later (and with irony that was not lost on the team), the e-invoicing project was not able to go live on time because it was identified that the supplier master data quality was too low. The go-live would have caused chaos because basic system functionality required the email and VAT fields for suppliers to be populated with a much higher level of completeness and accuracy than was available.
Both fields were in the scope of the data quality initiative, and our team had raised these concerns previously with the e-invoicing project team. The outcome was that the project had to be delayed by three months and the resources (costly consultants) had to be paid to complete the testing activities again.
What were the learnings from this experience?
Firstly, it is critical to start small. Pick one type of data (for example, customer or product data) where you know there are issues. The type of data you choose should be one where you can give tangible examples of the issues and what they mean to the company – in terms of revenue, costs, or compliance risks. Request a modest budget and show the value of what you have delivered through the issues that you have detected and resolved.
Secondly, make it part of your strategy when trying to obtain approvals to explain to key stakeholders (for example, business sponsors) why it is hard to quantify the benefits of data quality. Remember that they are used to seeing projects with quantitative business cases and they need a mindset shift before considering your data quality initiative. Meet with decision-makers individually before an approval board and make sure they understand this. Not everyone will be supportive, but in taking this approach, hopefully, enough debate is sparked to give you a better chance of approval.
Impacts of bad data in depth
We will now explore each element of our bad data definition in more depth. This section aims to outline in depth how poor data quality can affect organizations to help you look for these impacts in your own organization.
Process and efficiency impacts
Many organizations introduce SLAs for key processes – for example, 24 hours to create a new account for a new employee. These SLAs are critical because other processes are designed with an expectation that the SLA is met. For example, a hiring manager might be told that an employee can be onboarded within two weeks from the initial request. If one of the sub-processes (for example, new account creation) is delayed, this can lead to an employee arriving on site and being unable to be effective. Poor data quality can often cause SLAs to be missed. For example, if a new employee record is incorrectly assigned to an old organizational unit, the relevant approvals may not be triggered by the hiring manager and other leaders. This is surprisingly common – when re-organizations take place, legacy organizational units are often left in place.
Note
Every organization I have worked with asks for a response to a similar statement in their employee survey: “Processes at the organization allow me to be effective at work.” This statement always received the most negative response in the survey. When studying the text comments in response to this statement, I found that a significant percentage (around 30%) related to issues with data quality.
Here are further typical impacts on the organization when bad data causes SLAs to be missed:
Typical Impacts |
Example(s) |
The impacts are diverse. They can include the following:
|
A contract is signed with a supplier to start providing a service. The supplier has been used for many contracts in the past and there are multiple versions of this supplier in the system already. Procurement has to work out which version of the supplier record to associate the contract to, and this takes 2 weeks, against an SLA of 48 hours. The supplier is not able to provide resources on time as there is no purchase order, and resources are assigned to another project. It takes a further 4 weeks for appropriately skilled staff from the supplier to become available, leading to a 6 week delay in a critical project. |
Table 1.1 - Impacts and examples of missing SLAs
When bad data quality causes issues with processes, another impact can be on the budget for running that process. The organization of teams running processes is based on a certain level of expectation for process efficiency. Often, leaders and Human Resources professionals do not check the level of data quality before establishing teams. There is an assumption that data is of high enough quality to be used in the process and there is no resourcing allowance for remedial work. When data quality is not fit for purpose, then the team may not be correctly sized, resulting in the following impacts:
Typical Impacts |
Example(s) |
|
The accounts payable team for one business unit discover that invoices are routinely coded to another business unit by mistake. Invoices must be manually re-coded to the correct business unit before month-end processes can start. The month-end deadline is not adjusted; therefore, the team effort level is higher. |
Table 1.2 – Impacts and examples of incorrectly sized teas
When processes are unexpectedly impacted by data quality issues, it may not be possible to rapidly augment the team. In these situations, the focus of the team running the process is split. They must manage data quality issues on top of their usual tasks:
Typical Impacts |
Example(s) |
If a team cannot be augmented, the following can occur:
|
The accounts payable team can process payments for key suppliers. Key suppliers include those who provide raw materials for manufacturing. However, utility suppliers are not included in the priority list and are not paid on time, leading to facility utility outages. Manufacturing is halted while the issue is resolved. |
Table 1.3 – Impacts and examples of poor data quality on teams that cannot be augmented
Tables 1.1, 1.2, and 1.3 provide many of the typical impacts of data quality in the area of processes and efficiency. Many of those who are impacted by these will also be impacted again when they start to use reporting and analytics.
Reporting and analytics impacts
The main purpose of reports is to provide summarized data in a way that quickly conveys relevant information to a user and allows them to make a decision or help them with their day-to-day activities. Summarizing data can often mean that end users of reports are not best placed to detect data quality issues. The more senior the stakeholder, the more difficult it is for them to detect gaps in the data because they are looking at the highest level of summarized data.
For example, the following simple column chart shows the count of road traffic collisions in the UK in 2010 (source: https://www.kaggle.com/datasets/salmankhaliq22/road-traffic-collision-dataset).
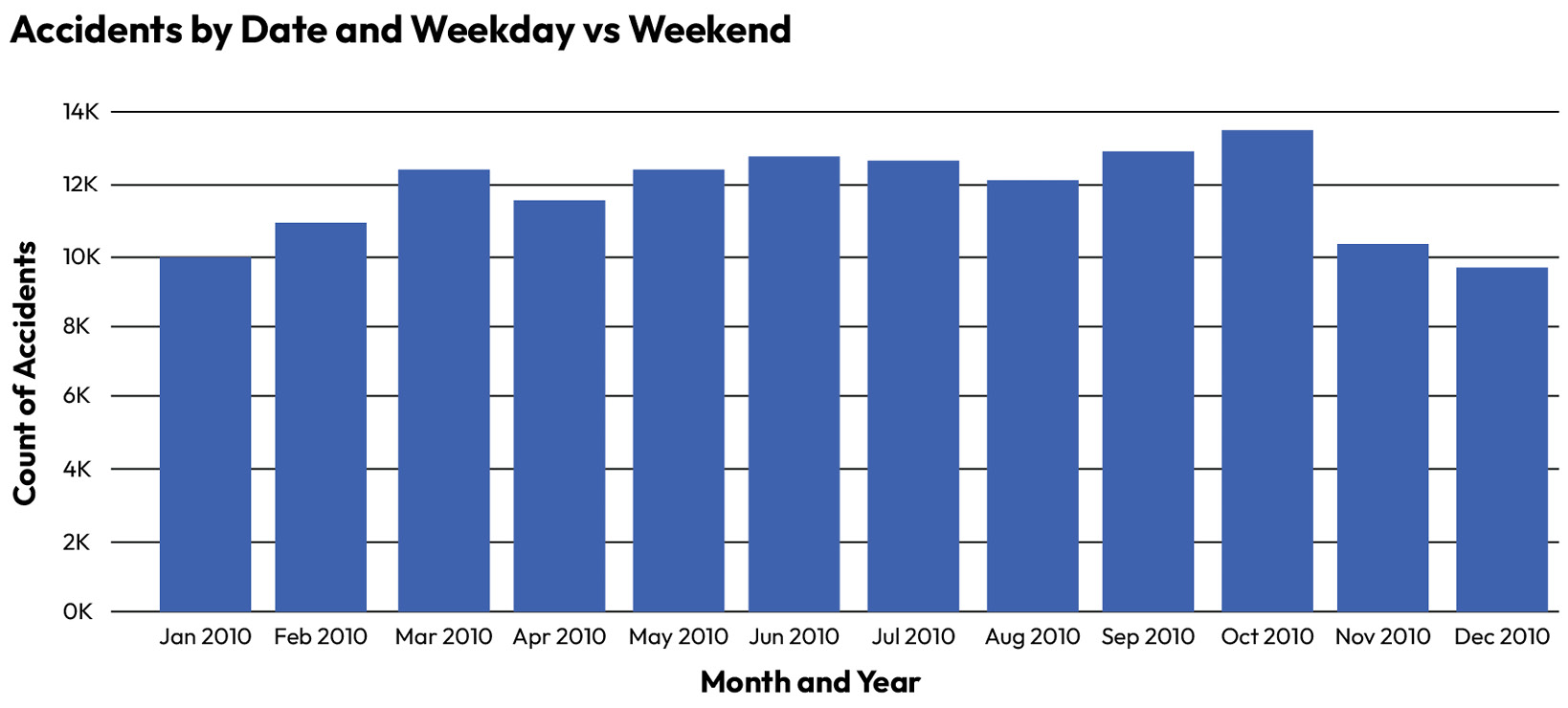
Figure 1.1 – Road traffic collision chart with missing data in November 2010
November 2010 looks like one of the best months in terms of collisions. Only December is better. However, a full week of data has been removed from November 2010 – but there is no way that the end user of this report could know that. Here is the correct chart:
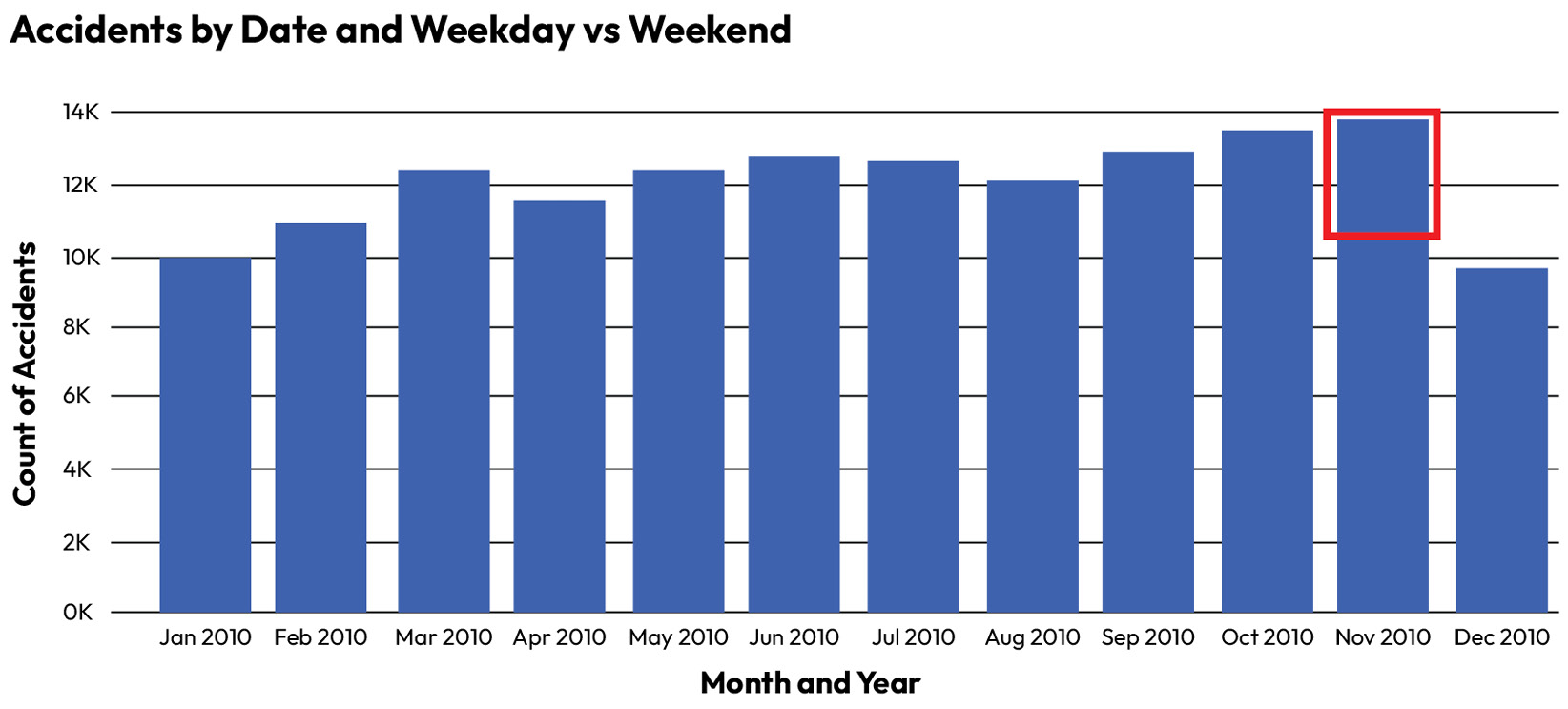
Figure 1.2 – Corrected road traffic collision chart
Here, we can see that November is actually the worst month of the year. There could be other major data quality issues in this dataset that an end user would find hard to detect – a whole region of the United Kingdom could be missing, for example. Some collisions could be misclassified into the wrong region.
All of these issues could drive incorrect decision-making. For example, the Department of Transport in the UK could decide to ban certain types of roadworks on major roads in October every year with a catch-up activity in November. In reality, this could drive a major increase in collisions in a month that is already the worst in the year.
In addition to the process and reporting impacts I've described so far, bad data can mean that an organization struggles to remain compliant with local laws and regulations. Let's explore the impacts and risks that can arise from issues with compliance.
Compliance impacts
Data quality issues can impact compliance for any organization – even those outside of regulated industries. Most companies have a financial audit every year and those with data quality issues will find that process challenging. The modern approach of external auditors is to assess internal systems, processes, and controls and, wherever possible, rely on those controls. The auditor tests that controls were in operation instead of checking the underlying records.
Historically, auditors would perform what they called a substantive audit where they would try to observe documents to support a high enough percentage of a particular number in the accounts. For example, if accounts receivable (amounts owed to the company by other companies) was £1m, the auditor would look for invoices to the total of around £600k and check that they had been properly accounted for (that is, they were unpaid at the period end). This would give them confidence about the whole balance of £1m.
In modern auditing, where controls are found to not be operating effectively, the auditor will exit from the controls-based approach and return to the substantive audit. This increases the audit fee substantially because of the time involved; it also consumes time from your internal resources. In the worst cases, auditors may actually qualify their audit opinion where there is an inability to obtain sufficient appropriate audit evidence. This qualified opinion appears in the company’s financial statements and is a huge red flag to investors.
However, companies in regulated industries have another set of challenges to face.
In Financial Services, the regulators request submissions of data in a particular taxonomy so that they can compare different financial institutions. The goal (particularly following the Lehmann Brothers collapse and resulting global financial crisis) is to ensure that institutions are being prudent enough in their lending to avoid future financial disruption. When the data is received by the regulator, it must meet stringent quality checks and submissions are frequently returned with required changes. Regulators will strengthen their oversight of an organization if they see poor practices in place. Strengthened oversight can even lead to institutions being required to retain more capital on their balance sheets (that is, reduce the amount they are lending and making a profit with!) if they lack confidence in management. Banking regulators have even introduced specific regulations for their industry about data governance. In Europe, the Basel Committee for Banking Supervision wrote a standard (BCBS 239) with the subject “Principles for effective risk data aggregation and risk reporting.” It includes principles such as “Governance, accuracy and integrity, completeness, timeliness,” and many more. See https://en.wikipedia.org/wiki/BCBS_239.
In pharmaceutical companies, medicinal products and devices are highly regulated by bodies such as the FDA in the United States and the MHRA in the United Kingdom. These regulators examine many aspects of a pharmaceutical company business – manufacturing, commercial, R&D, quality assurance, and quality control to name a few. Regulators expect to be able to inspect a site of the company with little to no warning and a data review would be a key part of this.
For example, deviations are a critical part of the pharmaceutical company data model. These are issues that are raised with any part of the company’s operations that can contribute to patient outcomes. They can be raised when something goes wrong in manufacturing, in a clinical trial, or even when an IT project does not go to plan. Regulators will inspect deviations, and if data quality is poor, the regulator may choose to apply their statutory powers to remedy the situation. The most serious issues can result in sites being shut down until improvements can be made. This has financial and reputational consequences for organizations, but the ultimate goal of regulation is to keep human beings safe. Data quality in pharmaceutical companies can be a matter of life and death!
The level of scrutiny and the risk of managing data poorly is so high for companies in these industries that investment in data governance in general tends to be higher. However, it should be noted that data initiatives in these organizations tend to move slowly because of the level of documentation and compliance required for implementation work.
More and more organizations are going beyond using data just for processes, reporting, and compliance in modern economies. We’ve already covered how these areas are impacted by bad data. If an organization is aiming to create or enhance streams of revenue by including data in their products or by making data itself the product, bad data can be disastrous.
Data differentiation impacts
There has been a major growth in businesses that use data to drive a revenue stream. An example of this is where data is a product in its own right (or part of a product), such as a database of doctor's offices (GP practices) in the UK, that is kept up to date by the owning company and sold to pharmaceutical companies to help with their sales pipelines and contact details.
Data is also often used by organizations as part of a differentiated customer experience. For example, online retailers use algorithms based partly on purchase history to present relevant recommendations to customers. If this purchase history were incomplete, the recommendations would lose relevance and fewer people would be enticed into their next purchase.
In these cases where the data itself is the product or part of the product, data quality is under the greatest scrutiny. It is no longer just your organization that is impacted by the quality issues – your customer is directly impacted now as well, leading to complaints, loss of revenue, and reputational damage. If you sell a range of data products, the low quality of one product might affect the sales of all data products!
Finally, and probably most seriously, there is the risk that where business partners (customers, suppliers, or employees) are exposed to poor data from your organization, the issue enters the public domain. With the prevalence of social media, a relatively isolated data quality issue posted by an influential person can harm the reputation of your company and give the impression that you are hard to do business with. At one organization the commercial team was talking to multiple customers about pricing for the year – which varied across different customers. The data quality of the source system was poor and was exported and combined with spreadsheet data to make it complete. This export was broken down into different spreadsheets to be shared with each customer. Unfortunately, one of the master data analysts made a mistake and sent the whole export to one of the customers – revealing other customers’ prices to that customer. This was a significant data breach and led to the employee being dismissed and the customer relationship breaking down as they saw that other customers were paying less for the same products, and they lost confidence in the organization’s ability to manage their data. This did not reach social media channels but became widely known in the industry and I saw it quoted as an example of poor practice in another company’s data training. It would just take a similar mistake to occur on data about individuals and there could be a GDPR breach with accompanying financial penalties and unwanted press attention. Data quality issues lead to workarounds with data, and workarounds lead to mistakes. Mistakes like these can destroy a business.
With all the negative impacts we have described, it can sometimes be hard to understand how organizations reach a point of having bad data in the first place. It is important to understand how this has occurred in your organization so that meaningful change can be made to avoid future re-occurrences.