Concept drift and drift detection
As discussed in the introduction of the chapter, the dynamic nature of infinite streams stands in direct opposition to the basic principles of stationary learning; that is, that the distribution of the data or patterns remain constant. Although there can be changes that are swift or abrupt, the discussion here is around slow, gradual changes. These slow, gradual changes are fairly hard to detect and separating the changes from the noise becomes tougher still:
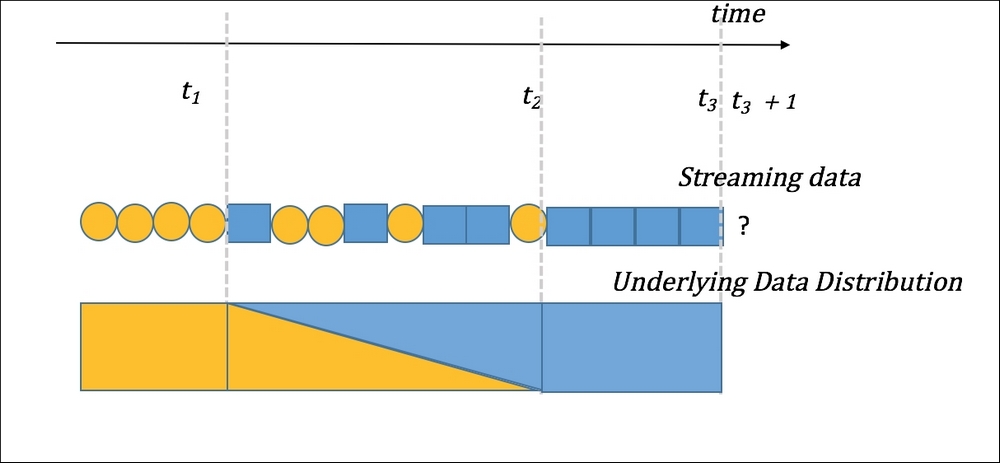
Figure 1 Concept drift illustrated by the gradual change in color from yellow to blue in the bottom panel. Sampled data reflects underlying change in data distribution, which must be detected and a new model learned.
There have been several techniques described in various studies in the last two decades that can be categorized as shown in the following figure:
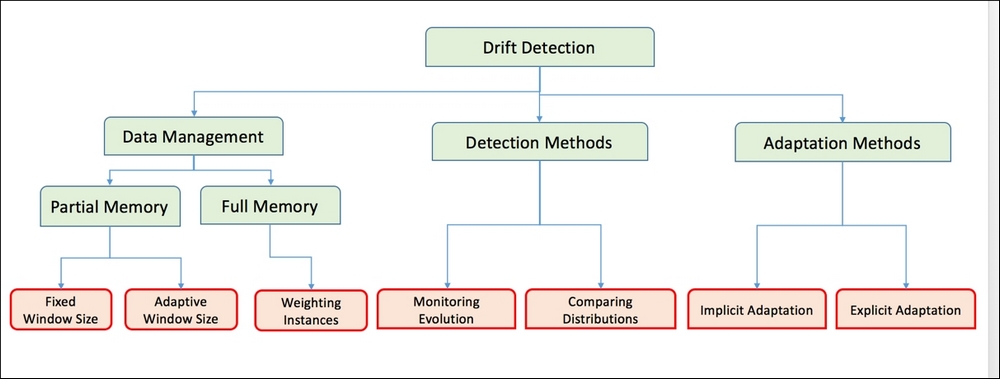
Figure 2 Categories of drift detection techniques
Data management
The main idea is to manage a model in memory that is consistent with the dynamic...