Training the baseline model
In this section, we will perform actual training using the following ML algorithms. This step is time-consuming as it needs more computation power. We use 75% of the training dataset for actual training and 25% of the dataset for testing in order to measure the training accuracy.
You can find the code snippet in the following figure:
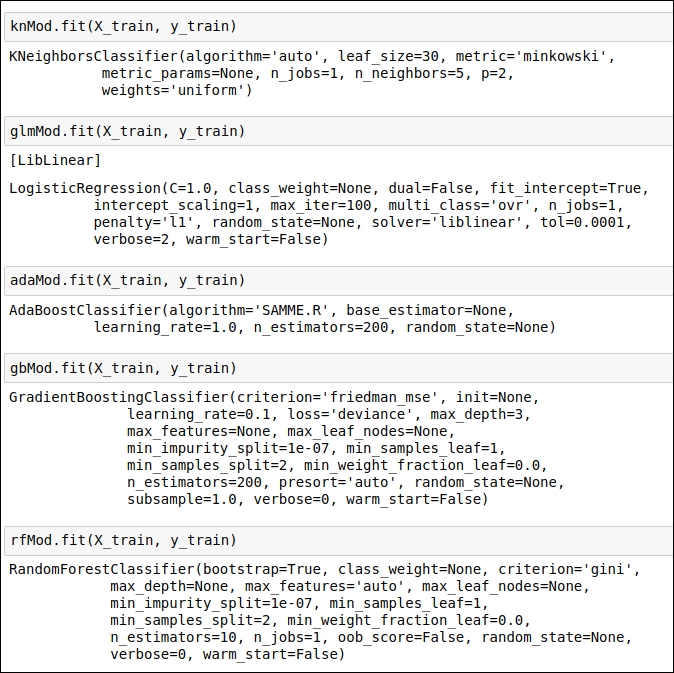
Figure 1.52: Code snippet for performing training
In the preceding code snippet, you can see that we performed the actual training operation using the fit()
function from the scikit-learn library. This function uses the given parameter and trains the model by taking the input of the target data attribute and other feature columns.
Once you are done with this step, you'll see that our different ML algorithms generate different trained models. Now it's time to check how good our trained model is when it comes to prediction. There are certain techniques that we can use on 25% of the dataset. In the next section, we will understand these techniques.