As we discussed earlier, background subtraction methods are affected by many factors. Their accuracy depends on how we capture the data and how it's processed. One of the biggest factors that affects these algorithms is the noise level. When we say noise, we are talking about things such as graininess in an image and isolated black/white pixels. These issues tend to affect the quality of our algorithms. This is where morphological image processing comes into play. Morphological image processing is used extensively in a lot of real-time systems to ensure the quality of the output. Morphological image processing refers to processing the shapes of features in the image; for example, you can make a shape thicker or thinner. Morphological operators rely not on how the pixels are ordered in an image, but on their values. This is why they are really...




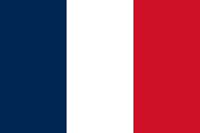


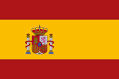



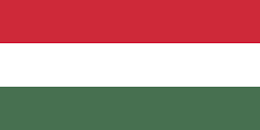

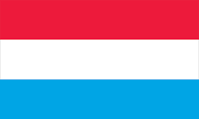





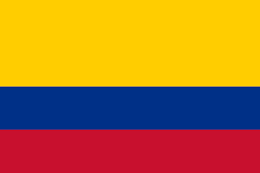
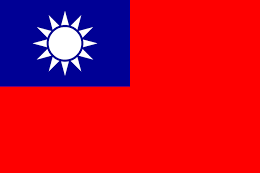
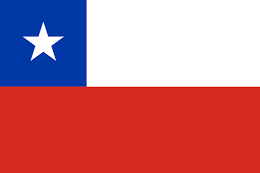



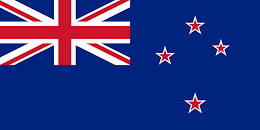
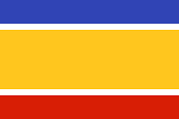
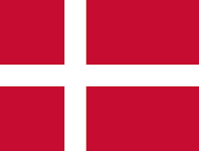
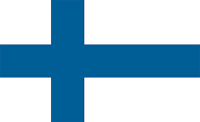







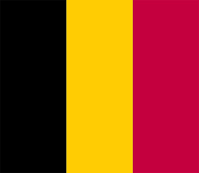

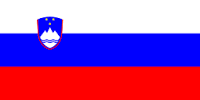





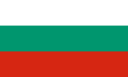


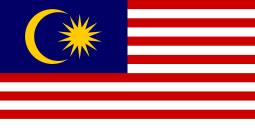



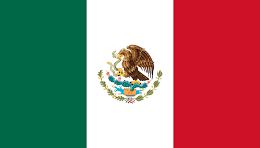
