So, we have seen so far how a single neuron may learn to represent a pattern, as it is trained. Now, let's say we want to leverage the learning mechanism of an additional neuron, in parallel. With two perceptron units in our model, each unit may learn to represent a different pattern in our data. Hence, if we wanted to scale the previous perceptron just a little bit by adding another neuron, we may get a structure with two fully connected layers of neurons, as shown in the following diagram:
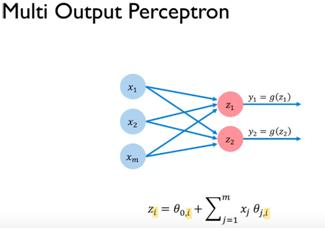
Note here that the feature weights, as well as the additional fictional input we will have per neuron to represent our bias, have both disappeared. To simplify our representation, we have instead denoted both the scalar dot product, and our bias term, as a single symbol.
We choose to represent this mathematical function as the letter z. The value of z is then...