Implementation of StackedGAN in Keras
The detailed network model of StackedGAN can be seen in the following figure. For conciseness, only two encoder-GANs per stack are shown. The figure may initially appear complex, but it is just a repetition of an encoder-GAN. Meaning that if we understood how to train one encoder-GAN, the rest uses the same concept. In the following section, we assume that the StackedGAN is designed for the MNIST digit generation:
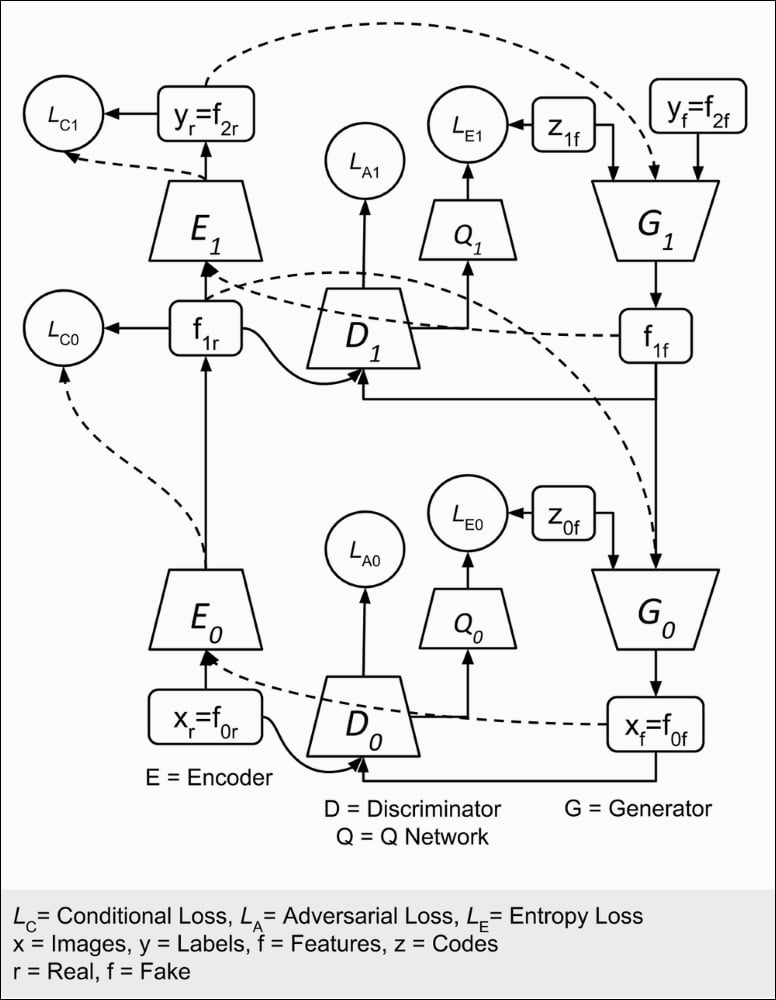
Figure 6.2.2: A StackedGAN is made of a stack of an encoder and GAN. The encoder is pre-trained to perform classification. Generator1, G1, learns to synthesize f1f features conditioned on the fake label, y f, and latent code, z1f. Generator0, G0, produces fake images using both the fake features, f1f and latent code, z0f.
StackedGAN starts with an Encoder. It could be a trained classifier that predicts the correct labels. The intermediate features vector, f1r, is made available for GAN training. For MNIST, we can use a CNN-based classifier similar...