



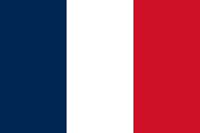


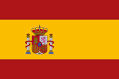



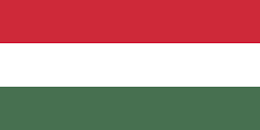

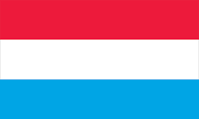





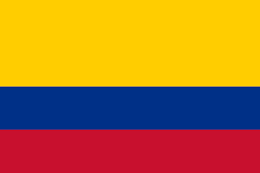
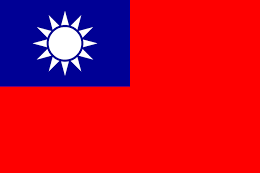
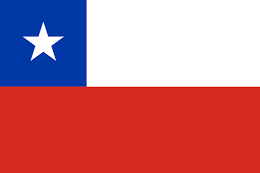



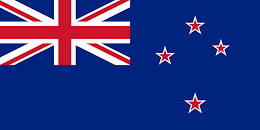
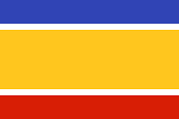
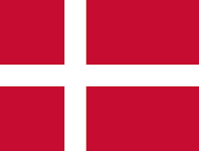
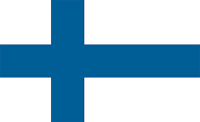







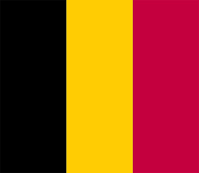

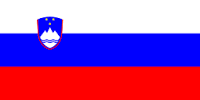





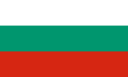


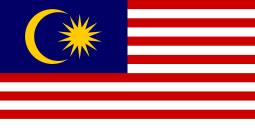



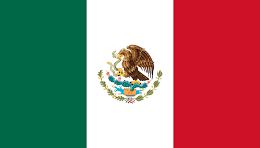

Eliza Strickland, the Senior Associate Editor at IEEE Spectrum, a magazine by the Institute of Electrical and Electronics Engineers, published an article, last week. The article talks about how IBM Watson still has a long way to go before it establishes itself as an efficient AI in the healthcare Industry.
IBM Watson, a question-answering computer system that is capable of answering questions in natural language, made the hits back in February 2011 when it defeated two human champions in the game of Jeopardy! a popular American Quiz Show. This was also the time when IBM researchers explored the possibilities of extending Watson’s capabilities to ‘revolutionize’ health care. IBM decided to apply the outstanding NLP capabilities of Watson to medicine and even promised a commercial product.
The first time IBM showed off Watson’s potential to transform medicine using AI was back in 2014. For the Demo, Watson was fed a bizarre collection of patient symptoms, using which, it produced a list of possible diagnoses. Watson’s memory bank included information on even the rarest of diseases and its processors were totally unbiased in approach, giving it an edge over other AIs for doctors.
“If Watson could bring that instant expertise to hospitals and clinics all around the world, it seemed possible that the AI could reduce diagnosis errors, optimize treatments, and even alleviate doctor shortages—not by replacing doctors but by helping them do their jobs faster and better,” writes Strickland.
However, despite promising on new projects related to AI commercial products, it could not follow up on that promise.
“In the eight years since, IBM has trumpeted many more high-profile efforts to develop AI-powered medical technology—many of which have fizzled and a few of which have failed spectacularly,” writes Strickland. Moreover, the products that have been produced from the IBM Watson Health Division, are more like basic AI assistants that are capable of performing routine tasks, not even close to being an AI doctor.
While IBM was considering Watson’s possibilities in the healthcare industry, the most challenging issue at the time was the fact that the bulk of patient data in Medicine, i.e. unstructured data. This includes doctor’s notes and hospital discharge summaries which accounts for about 80 percent of a typical patient’s record and is an amalgamation of jargon, shorthand, and subjective statements.
Another challenge faced by IBM Watson is its diagnosis of cancer. Mark Kris, a lung cancer specialist at Memorial Sloan Kettering Cancer Center, New York City along with the other preeminent physicians trained an AI system known as Watson for Oncology in 2015. Watson for Oncology would learn by ingesting the vast medical literature on cancer and the health records of real cancer patients and uncover patterns unknown to humans.
The other preeminent physicians at the University of Texas MD Anderson Cancer Center, in Houston, collaborated with IBM to create a tool called Oncology Expert Advisor. Both the products, however, faced severe criticism saying that Watson for Oncology at times provided ‘useless’ and ‘dangerous recommendations’.
“A deeper look at these two projects reveals a fundamental mismatch between the promise of machine learning and the reality of medical care—between “real AI” and the requirements of a functional product for today’s doctors”, writes Strickland.
Although Watson learned quickly about scanning articles on clinical studies, it was difficult to teach Watson to read the articles the way a doctor would. “The information that physicians extract from an article, that they use to change their care, may not be the major point of the study. Watson’s thinking is based on statistics, so all it can do is gather statistics about main outcomes”, adds Mark Kris.
Researchers further found that Watson was also incapable of mining information from patients’ electronic health records. Also, they realized that Watson is incapacitated when it comes to comparing a new patient with another large number of cancer patients to discover hidden patterns. Further, they hoped that Watson would mimic the abilities of expert oncologists, but they were disappointed.
Despite some challenges, IBM Watson has also seen its share of success stories. Strickland cites an example of Watson for Genomics developed in partnership with the University of North Carolina, Yale University, and other renowned institutions. The tool helps genetics lab generate reports for practicing oncologists. Watson ingests lists of patient’s genetic mutations and generates a report describing all the relevant drugs and clinical trials in just a few seconds. Moreover, a paper was published by IBM’s partners at the University of North Carolina on the effectiveness of Watson for Genomics in 2017
Effective or not, IBM Watson still has a long queue of hurdles that it needs to cross before IBM reaches its dream of making Watson the impeccable ‘AI doctor’.
For more information, check out the official IEEE Spectrum article.
IBM CEO, Ginni Rometty, on bringing HR evolution with AI and its predictive attrition AI
IBM sued by former employees on violating age discrimination laws in workplace
IBM announces the launch of Blockchain World Wire, a global blockchain network for cross-border payments