We looked at the DDPG algorithm in the previous chapter. One of the main drawbacks of the DDPG algorithm (as well as the DQN algorithm that we saw earlier) is the use of a replay buffer to obtain independent and identically distributed samples of data for training. Using a replay buffer consumes a lot of memory, which is not desirable for robust RL applications. To overcome this problem, researchers at Google DeepMind came up with an on-policy algorithm called Asynchronous Advantage Actor Critic (A3C). A3C does not use a replay buffer; instead, it uses parallel worker processors, where different instances of the environment are created and the experience samples are collected. Once a finite and fixed number of samples are collected, they are used to compute the policy gradients, which are asynchronously sent to a central processor that updates...




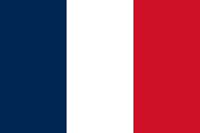


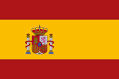



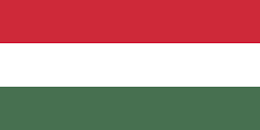

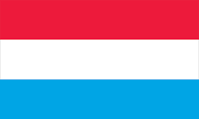





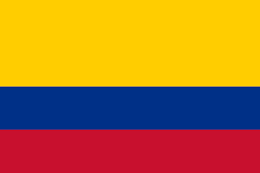
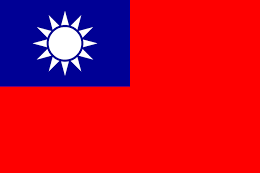
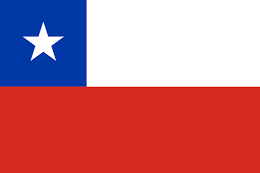



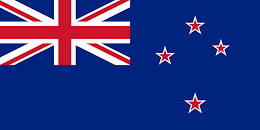
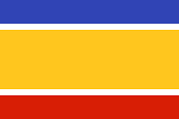
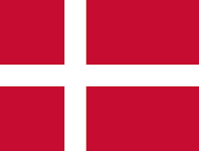
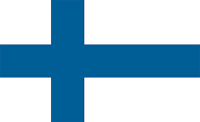







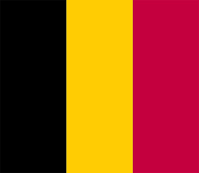

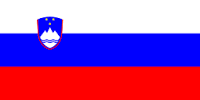





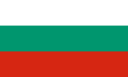


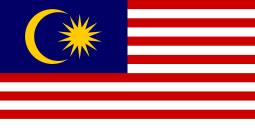



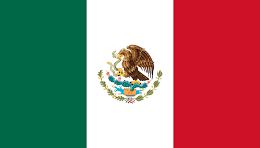
