Regression is an inductive learning task that has been widely studied and is widely used in practical applications. Unlike classification processes, where you are trying to predict discrete class labels, regression models predict numeric values.
From a set of data, we can find a model that describes it by the use of the regression algorithms. For example, we can identify a correspondence between input variables and output variables of a given system. One way to do this is to postulate the existence of some kind of mechanism for the parametric generation of data; this, however, does not contain the exact values of the parameters. This process typically makes reference to statistical techniques.
The extraction of general laws from a set of observed data is called induction, as opposed to deduction in which we start from general laws and try to predict the value of a set of variables. Induction is the fundamental mechanism underlying the scientific method in which we want to derive general laws (typically described in mathematical terms) starting from the observation of phenomena. In the following figure, we can see Peirce's triangle, which represents a scheme of relationships between reasoning patterns:
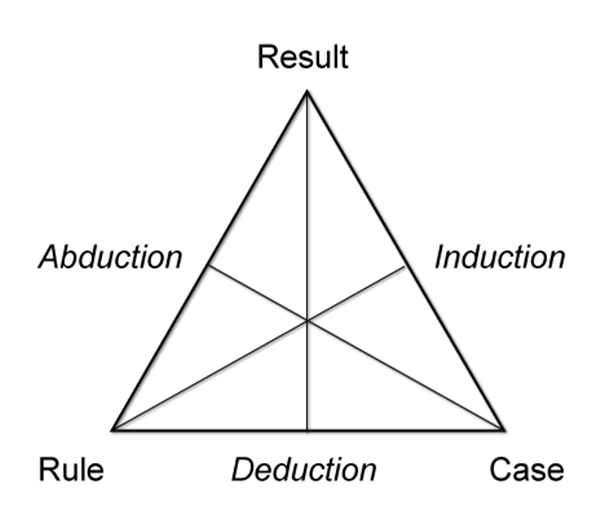
The observation of the phenomena includes the measurement of a set of variables, and therefore the acquisition of data that describes the observed phenomena. Then, the resulting model can be used to make predictions on additional data. The overall process in which, starting from a set of observations, we aim to make predictions on new situations, is called inference.
Therefore, inductive learning starts with observations arising from the surrounding environment that, hopefully, are also valid for not-yet-observed cases.
We have already anticipated the stages of the inference process; now let's analyze them in detail through the workflow setting. When developing an application that uses regression algorithms, we will follow a procedure characterized by the following steps:
- Collect the data: Everything starts with the data—no doubt about it—but one might wonder where so much data comes from. In practice, data is collected through lengthy procedures that may, for example, be derived from measurement campaigns or face-to-face interviews. In all cases, data is collected in a database so that it can then be analyzed to obtain knowledge.
If we do not have specific requirements, and to save time and effort, we can use publicly available data. In this regard, a large collection of data is available at the UCI Machine Learning Repository, at the following link: http://archive.ics.uci.edu/ml.
The following figure shows the regression process workflow:
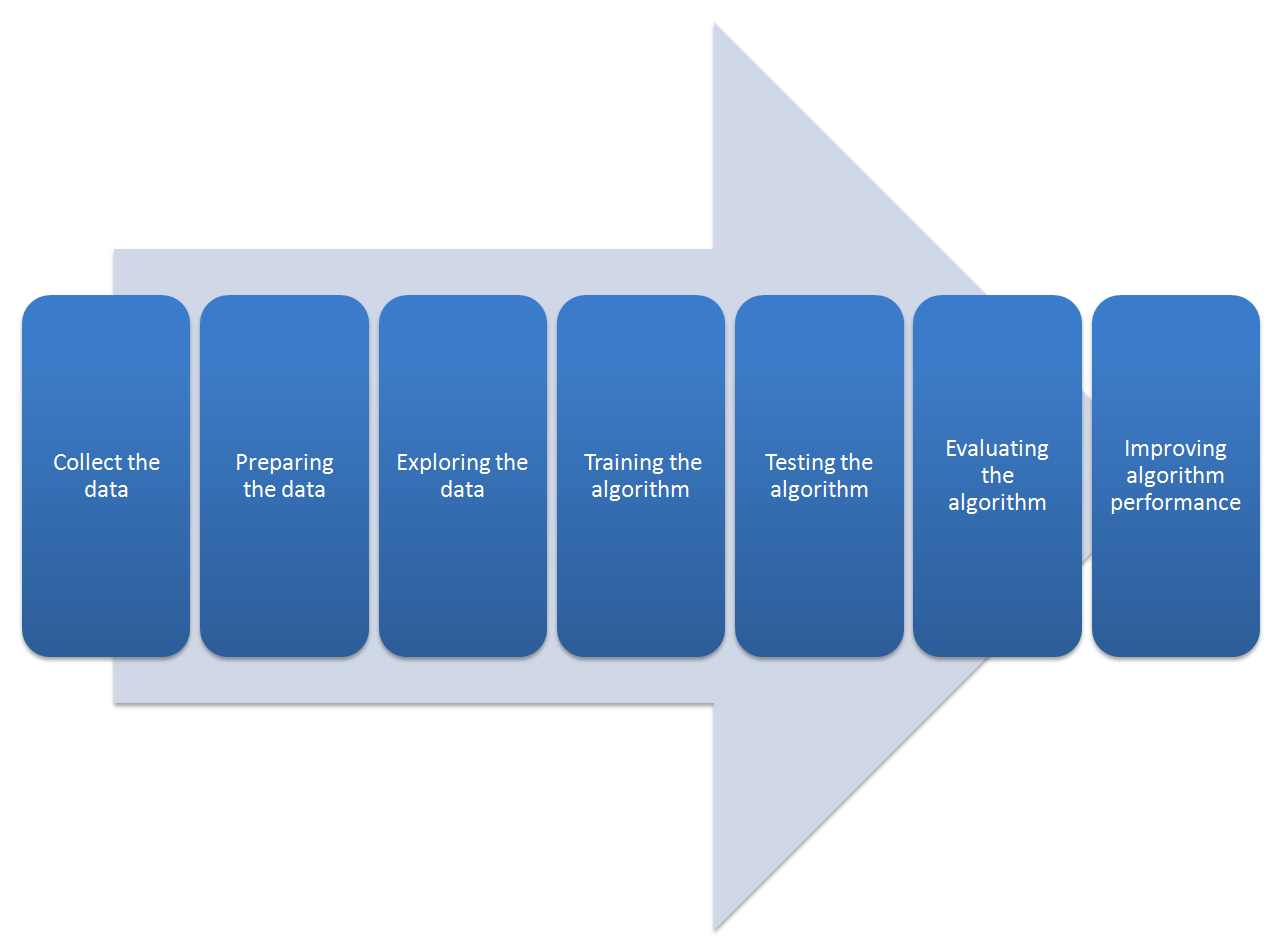
- Preparing the data: We have collected the data; now we have to prepare it for the next step. Once you have this data, you must make sure it is in a format usable by the algorithm you want to use. To do this, you may need to do some formatting. Recall that some algorithms need data in an integer format, whereas some require it in the form of strings, and finally others need it to be in a special format. We will get to this later, but the specific formatting is usually simple compared to data collection.
- Exploring the data: At this point, we can look at the data to verify that it is actually working and we do not have a bunch of empty values. In this step, through the use of plots, we can recognize any patterns or check whether there are some data points that are vastly different from the rest of the set. Plotting data in one, two, or three dimensions can also help.
- Training the algorithm: At this stage, it starts to get serious. The regression algorithm begins to work with the definition of the model and the next training step. The model starts to extract knowledge from the large amounts of data that we have available.
- Testing the algorithm: In this step, we use the information learned in the previous step to see whether the model actually works. The evaluation of an algorithm is for seeing how well the model approximates the real system. In the case of regression techniques, we have some known values that we can use to evaluate the algorithm. So, if we are not satisfied, we can return to the previous steps, change some things, and retry the test.
- Evaluating the algorithm: We have reached the point where we can apply what has been done so far. We can assess the approximation ability of the model by applying it to real data. The model, preventively trained and tested, is then valued in this phase.
- Improving algorithm performance: Finally, we can focus on finishing the work. We have verified that the model works, we have evaluated the performance, and now we are ready to analyze it completely to identify possible room for improvement.
The generalization ability of the regression model is crucial for all other machine learning algorithms as well. Regression algorithms must not only detect the relationships between the target function and attribute values ​​in the training set, but also generalize them so that they may be used to predict new data.
It should be emphasized that the learning process must be able to capture the underlying regimes from the training set and not the specific details. Once the learning process is completed through training, the effectiveness of the model is tested further on a dataset named testset.