Naive Bayes is one of the simplest and fastest ML algorithms. This too belongs to the class of supervised learning algorithms. It's based on the Bayes probability theorem. One important assumption that we make in the case of the Naive Bayes classifier is that all of the features of the input vector are independent and identically distributed (iid). The goal is to learn a conditional probability model for each class Ck in the training dataset:

Under the iid assumption, and using the Bayes theorem, this can be expressed in terms of the joint probability distribution p(Ck, X):

We pick the class that maximizes this term Maximum A Posteriori (MAP):
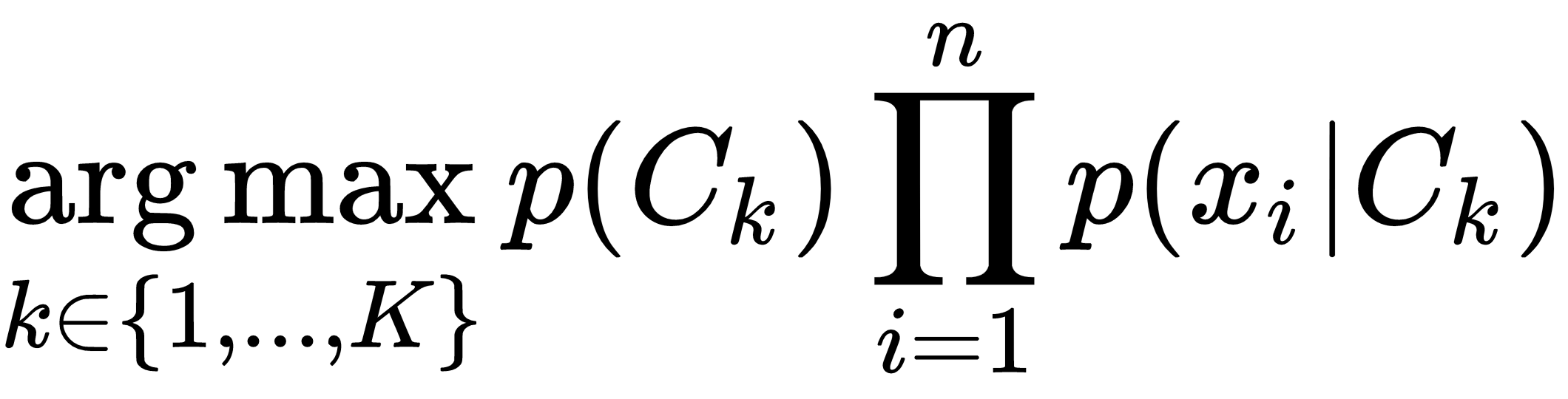
There can be different Naive Bayes algorithms, depending upon the distribution of p(xi|Ck). The common choices are Gaussian in the case of real-valued data, Bernoulli for binary data, and MultiNomial when the data contains...