



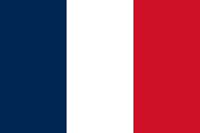


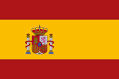



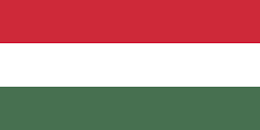

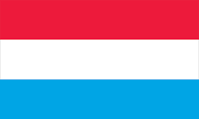





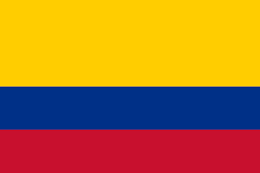
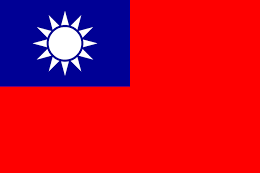
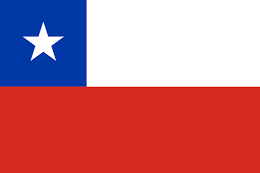



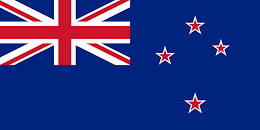
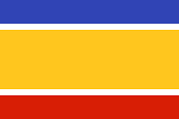
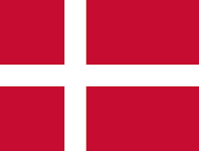
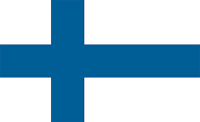







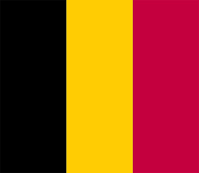

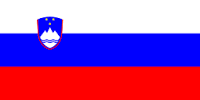





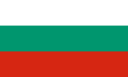


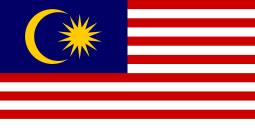



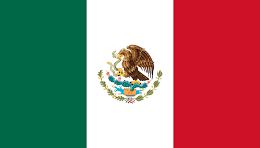

There has been a huge shift in the way that businesses build technology in recent years driven by a move towards cloud and microservices. Public cloud services like AWS, Microsoft Azure, and Google Cloud Platform are transforming the way companies of all sizes understand and use software. Not only do public cloud services reduce the resourcing costs associated with on site server resources, they also make it easier to leverage cutting edge technological innovations like machine learning and artificial intelligence. Cloud is giving rise to what’s known as ‘Machine Learning as a Service’ - a trend that could prove to be transformative for organizations of all types and sizes.
According to a report published on Research and Markets, Machine Learning as a Service is set to face a compound annual growth rate (CAGR) of 49% between 2017 and 2023. The main drivers of this growth include the increased application of advanced analytics in manufacturing, the high volume of structured and unstructured data, and the integration of machine learning with big data.
Of course, with machine learning a relatively new area for many businesses, demand for MLaaS is ultimately self-fulfilling - if it’s there and people can see the benefits it can bring, demand is only going to continue.
But it’s important not to get fazed by the hype. Plenty of money will be spent on cloud based machine learning products that won’t help anyone but the tech giants who run the public clouds. With that in mind, let’s dive deeper into Machine Learning as a Service and what the biggest cloud vendors offer.
Machine learning as a Service (MLaaS) is an array of services that provides machine learning tools to users. Businesses and developers can incorporate a machine learning model into their application without having to work on its implementation. These services range from data visualization, facial recognition, natural language processing, chatbots, predictive analytics and deep learning, among others.
Typically, for a given machine learning task, a user has to perform various steps. These steps include data preprocessing, feature identification, implementing the machine learning model, and training the model. MLaaS services simplify this process by only exposing a subset of the steps to the user while automatically managing the remaining steps. Some services can also provide 1-click mode, where the users does not have to perform any of the steps mentioned earlier.
Large companies can afford to hire expert machine learning engineers and data scientists, but they still have to build and manage their own custom machine learning model. This is time-intensive and complicated process. By leveraging MLaaS services these companies can use pre-trained machine learning models via APIs that perform specific tasks and save time.
Big companies can invest in their own machine learning solutions because they have the resources. For small and mid-sized businesses (SMBs), however, this simply isn’t the case. Fortunately, MLaaS changes all that and makes machine learning accessible to organizations with resource limitations.
By using MLaaS, businesses can leverage machine learning without the huge investment in infrastructure or talent. Whether it’s for smarter and more intelligent customer-facing apps, or improved operational intelligence and automation, this could bring huge gains for a reasonable amount of spending.
Machine learning can contribute to any kind of app development provided you have data to train your app. However, adding AI features to your app is not easy. As a developer, you’ve to worry about a lot of other factors besides regular app development checklist, in order to make your app intelligent. Some of them are:
The development tools provided by MLaaS can simplify these tasks allowing you to easily embed machine learning in your applications. Developers can build quickly and efficiently with MLaaS offerings, because they have access to pre-built algorithms and models that would take them extensive resources to build otherwise.
MLaaS can also support data scientists and analysts. While most data scientists should have the necessary skills to build and train machine learning models from scratch, it can nevertheless still be a time consuming task. MLaaS can, as already mentioned, simplify the machine learning engineering process, which means data scientists can focus on optimizations that require more thought and expertise.
Amazon Web Services (AWS), Azure, and Google, all have MLaaS products in their cloud offerings. Let’s take a look at them. Google Cloud AI at a glance
Google’s Cloud AI provides modern machine learning services. It consists of pre-trained models and a service to generate your own tailored models. The services provided are fast, scalable, and easy to use.
The following are the services that Google provides at an unprecedented scale and speed to your applications:
It is a suite of machine learning products, with the help of which developers with limited machine learning expertise can train high-quality models specific to their business needs. It provides you a simple GUI to train, evaluate, improve, and deploy models based on your own data.
Read also: AmoebaNets: Google’s new evolutionary AutoML
Google Cloud Machine Learning Engine is a service that offers training and prediction services to enable developers and data scientists to build superior machine learning models and deploy in production. You don’t have to worry about infrastructure and can instead focus on the model development and deployment. It offers two types of predictions:
Read also: Google announces Cloud TPUs on the Cloud Machine Learning Engine (ML Engine)
It is a cloud data warehouse for data analytics. It uses SQL and provides Java Database Connectivity (JDBC) and Open Database Connectivity (ODBC) drivers to make integration fast and easy. It provides benefits like auto scaling and high-performance streaming to load data. You can create amazing reports and dashboards using your favorite BI tool, like Tableau, MicroStrategy, Looker etc.
Read also: Getting started with Google Data Studio: An intuitive tool for visualizing BigQuery Data
Dialogflow is an end-to-end, build-once deploy-everywhere development suite for creating conversational interfaces for websites, mobile applications, popular messaging platforms, and IoT devices. Dialogflow Enterprise Edition users have access to Google Cloud Support and a service level agreement (SLA) for production deployments.
Read also: Google launches the Enterprise edition of Dialogflow, its chatbot API
Google Cloud Speech-to-Text allows you to convert speech to text by applying neural network models. 120 languages are supported by the API, which will help you extend your user base. It can process both real-time streaming and prerecorded audio.
Read also: Google announce the largest overhaul of their Cloud Speech-to-Text
The Azure platform consists of various AI tools and services that can help you build smart applications. It provides Cognitive Services and Conversational AI with Bot tools, which facilitate building custom models with Azure Machine Learning for any scenario. You can run AI workloads anywhere at scale using its enterprise-grade AI infrastructure
The following are services provided by Azure AI to help you achieve maximum productivity and reliability:
You need not be an expert in data science to make your systems more intelligent and engaging. The pre-built services come with high-quality RESTful intelligent APIs for the following:
Azure Machine Learning is a fully managed cloud service which helps you to easily prepare data, build, and train your own models:
Read also: Microsoft supercharges its Azure AI platform with new features
Machine learning services provided by AWS help developers to easily add intelligence to any application with pre-trained services. For training and inferencing, it offers a broad array of compute options with powerful GPU-based instances, compute and memory optimized instances, and even FPGAs. You will get to choose from a set of services for data analysis including data warehousing, business intelligence, batch processing, stream processing, and data workflow orchestration.
The following are the services provided by AWS:
Read also: AWS makes Amazon Rekognition, its image recognition AI, available for Asia-Pacific developers
Read also: Amazon Sagemaker makes machine learning on the cloud easy.
Machine learning and artificial intelligence can be expensive - skills and resources can cost a lot. For that reason, MLaaS is going to be a hugely influential development within cloud.
Yes, the range of services on offer are impressive from AWS, Azure and GCP, but it’s really the ease and convenience that is most remarkable. With these services it’s easy to set up and run machine learning algorithms that enhance business processes and operations, customer interactions and overall business strategy. You don’t need a PhD, and you don’t need to code algorithms from scratch. The MLaaS market will likely continue to grow as more companies realise the potential machine learning has on their business - however, whether anyone can deliver a better set of services than the established cloud providers remains to be seen.
Predictive Analytics with AWS: A quick look at Amazon ML
Microsoft supercharges its Azure AI platform with new features
AmoebaNets: Google’s new evolutionary AutoML