



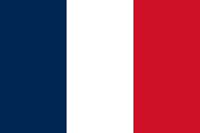


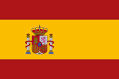



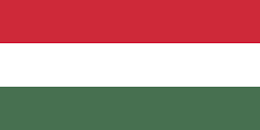

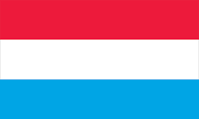





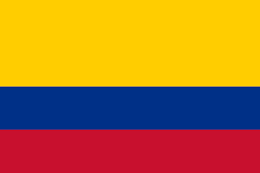
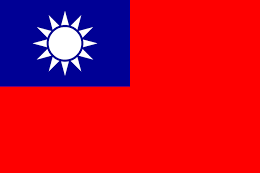
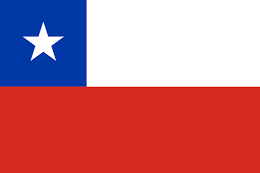



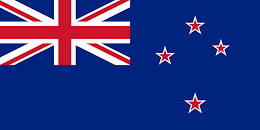
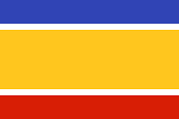
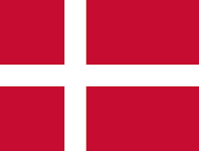
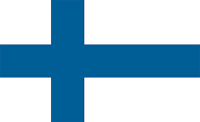







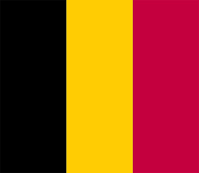

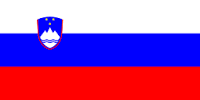





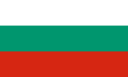


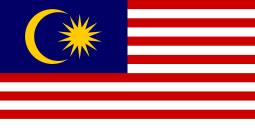



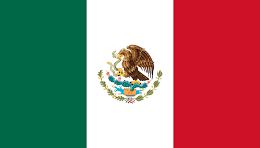

Navigating the world of data and AI systems can be overwhelming.
Their complexity often makes it difficult to visualize how data engineering, research (data science and machine learning), and production roles (AI engineering, ML engineering, MLOps) work together to form an end-to-end system.
But is there a more intuitive and accessible way to comprehend the entire end-to-end data and AI ecosystem?
Absolutely—through the FTI architecture.
Let’s quickly dig into the FTI architecture and apply it to a production LLM & RAG use case.
Figure 1: The mess of bringing structure between the common elements of an ML system.
The FTI architecture proposes a clear and straightforward mind map that any team or person can follow to compute the features, train the model, and deploy an inference pipeline to make predictions.
The pattern suggests that any ML system can be boiled down to these 3 pipelines: feature, training, and inference.
This is powerful, as we can clearly define the scope and interface of each pipeline. Ultimately, we have just 3 instead of 20 moving pieces, as suggested in Figure 1, which is much easier to work with and define.
Figure 2 shows the feature, training, and inference pipelines. We will zoom in on each one to understand its scope and interface.
Before going into the details, it is essential to understand that each pipeline is a separate component that can run on different processes or hardware. Thus, each pipeline can be written using a different technology, by a different team, or scaled differently.
The feature pipeline takes raw data as input, processes it, and outputs the features and labels required by the model for training or inference.
Instead of directly passing them to the model, the features and labels are stored inside a feature store. Its responsibility is to store, version, track, and share the features.
By saving the features into a feature store, we always have a state of our features. Thus, we can easily send the features to the training and inference pipelines.
The training pipeline takes the features and labels from the features stored as input and outputs a trained model(s).
The models are stored in a model registry. Its role is similar to that of feature stores, but the model is the first-class citizen this time. Thus, the model registry will store, version, track, and share the model with the inference pipeline.
The inference pipeline takes as input the features and labels from the feature store and the trained model from the model registry. With these two, predictions can be easily made in either batch or real-time mode.
As this is a versatile pattern, it is up to you to decide what you do with your predictions. If it’s a batch system, they will probably be stored in a DB. If it’s a real-time system, the predictions will be served to the client who requested them.
The most important thing you must remember about the FTI pipelines is their interface. It doesn’t matter how complex your ML system gets — these interfaces will remain the same.
The final thing you must understand about the FTI pattern is that the system doesn’t have to contain only 3 pipelines. In most cases, it will include more.
For example, the feature pipeline can be composed of a service that computes the features and one that validates the data. Also, the training pipeline can comprise the training and evaluation components.
The FTI architecture is tool-agnostic, but to better understand how it works, let’s present a concrete use case and tech stack.
Use case: Fine-tune an LLM on your social media data (LinkedIn, Medium, GitHub) and expose it as a real-time RAG application. Let’s call it your LLM Twin.
As we build an end-to-end system, we split it into 4 pipelines:
As the FTI architecture defines a straightforward interface, we can easily connect the data collection pipeline to the ML components through a data warehouse, which, in our case, is a MongoDB NoSQL DB.
The feature pipeline (the second ML-oriented data pipeline) can easily extract standardized data from the data warehouse and preprocess it for fine-tuning and RAG.
The communication between the two is done solely through the data warehouse. Thus, the feature pipeline isn’t aware of the data collection pipeline and how it collected the raw data.
Figure 3: LLM Twin high-level architecture
The feature pipeline does two things:
chunks, embeds and loads the data to a Qdrant vector DB for RAG;
generates an instruct dataset and loads it into a versioned ZenML artifact.
The training pipeline ingests a specific version of the instruct dataset, fine-tunes an open-source LLM from HuggingFace, such as Llama 3.1, and pushes it to a HuggingFace model registry.
During the research phase, we use a Comet ML experiment tracker to compare multiple fine-tuning experiments and push only the best one to the model registry.
During production, we can automate the training job and use our LLM evaluation strategy or canary tests to check if the new LLM is fit for production.
As the input dataset and output model registry are decoupled, we can quickly launch our training jobs using ML platforms like AWS SageMaker.
ZenML orchestrates the data collection, feature, and training pipelines. Thus, we can easily schedule them or run them on demand or
The end-to-end RAG application is implemented in the inference pipeline side, which accesses fresh documents from the Qdrant vector DB and the latest model from the HuggingFace model registry.
Here, we can implement advanced RAG techniques such as query expansion, self-query and rerank to improve the accuracy of our retrieval step for better context during the generation step.
The fine-tuned LLM will be deployed to AWS SageMaker as an inference endpoint. Meanwhile, the rest of the RAG application is hosted as a FastAPI server, exposing the end-to-end logic as REST API endpoints.
The last step is to collect the input prompts and generated answers with a prompt monitoring tool such as Opik to evaluate the production LLM for things such as hallucinations, moderation or domain-specific problems such as writing tone and style.
The FTI architecture is a powerful mindmap that helps you connect the dots in the complex data and AI world, as illustrated in the LLM Twin use case.
Unlock the full potential of Large Language Models with the "LLM Engineer's Handbook" by Paul Iusztin and Maxime Labonne. Dive deeper into real-world applications, like the FTI architecture, and learn how to seamlessly connect data engineering, ML pipelines, and AI production. With practical insights and step-by-step guidance, this handbook is an essential resource for anyone looking to master end-to-end AI systems. Don’t just read about AI—start building it. Get your copy today and transform how you approach LLM engineering!
Paul Iusztin is a senior ML and MLOps engineer at Metaphysic, a leading GenAI platform, serving as one of their core engineers in taking their deep learning products to production. Along with Metaphysic, with over seven years of experience, he built GenAI, Computer Vision and MLOps solutions for CoreAI, Everseen, and Continental. Paul's determined passion and mission are to build data-intensive AI/ML products that serve the world and educate others about the process. As the Founder of Decoding ML, a channel for battle-tested content on learning how to design, code, and deploy production-grade ML, Paul has significantly enriched the engineering and MLOps community. His weekly content on ML engineering and his open-source courses focusing on end-to-end ML life cycles, such as Hands-on LLMs and LLM Twin, testify to his valuable contributions.