Building a CNN autoencoder
Let’s start by going through what a transpose convolution is. Figure 5.3 shows an example transpose convolution operation on a 2x2 sized input with a 2x2 sized convolutional filter, with a stride of 1.
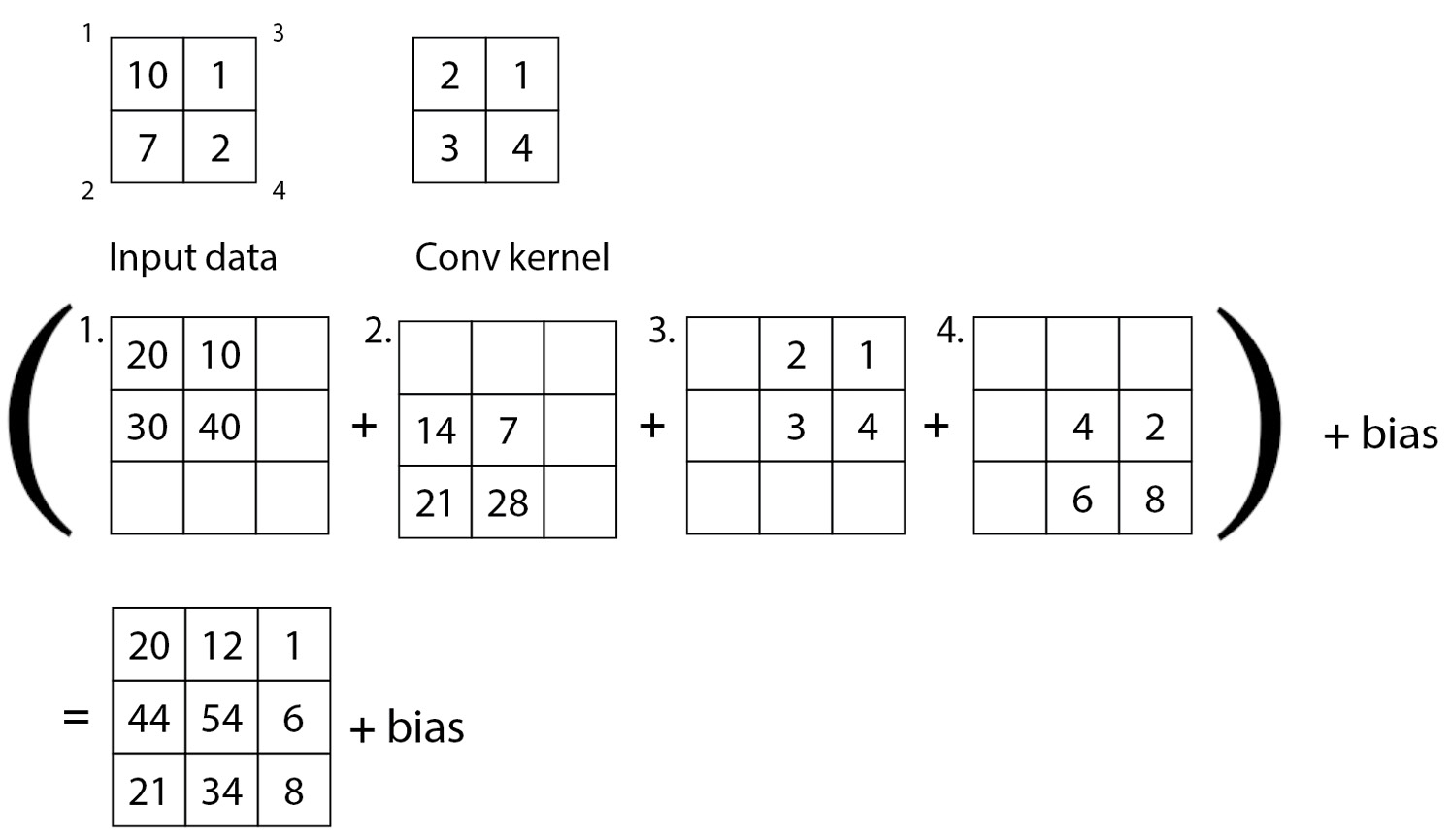
Figure 5.3 – A transposed convolutional filter operation
In Figure 5.3, note that each of the 2x2 input data is marked with a number from 1 to 4. These numbers are used to map the output results, presented as 3x3 outputs. The convolutional kernel applies each of its weights individually to every value in the input data in a sliding window manner, and the outputs from the four convolutional operations are presented in the bottom part of the figure. After the operation is done, each of the outputs will be elementwise added to form the final output and subjected to a bias. This example process depicts how a 2x2 input can be scaled up to a 3x3 data size without relying completely on padding.
Let’s implement...