Optimization of neural networks
Various techniques have been used for optimizing the weights of neural networks:
- Stochastic gradient descent (SGD)
- Momentum
- Nesterov accelerated gradient (NAG)
- Adaptive gradient (Adagrad)
- Adadelta
- RMSprop
- Adaptive moment estimation (Adam)
- Limited memory Broyden-Fletcher-Goldfarb-Shanno (L-BFGS)
In practice, Adam is a good default choice; we will be covering its working methodology in this section. If you cannot afford full batch updates, then try out L-BFGS:
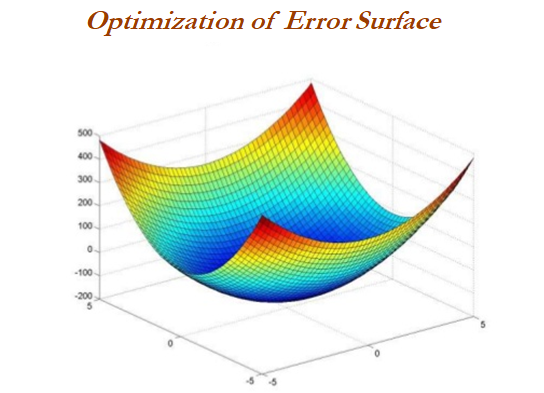
Stochastic gradient descent - SGD
Gradient descent is a way to minimize an objective function J(θ) parameterized by a model's parameter θ ε Rd by updating the parameters in the opposite direction of the gradient of the objective function with regard to the parameters. The learning rate determines the size of the steps taken to reach the minimum:
- Batch gradient descent (all training observations utilized in each iteration)
- SGD (one observation per iteration)
- Mini batch gradient descent (size of about 50 training observations...