Using regularized methods for regression
As we discussed in Chapter 3, A Tour of Machine Learning Classifiers Using scikit-learn, regularization is one approach to tackling the problem of overfitting by adding additional information, and thereby shrinking the parameter values of the model to induce a penalty against complexity. The most popular approaches to regularized linear regression are the so-called Ridge Regression, least absolute shrinkage and selection operator (LASSO), and elastic Net.
Ridge Regression is an L2 penalized model where we simply add the squared sum of the weights to our least-squares cost function:
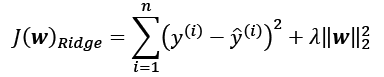
Here:
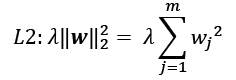
By increasing the value of hyperparameter , we increase the regularization strength and thereby shrink the weights of our model. Please note that we don't regularize the intercept term,
.
An alternative approach that can lead to sparse models is LASSO. Depending on the regularization strength, certain weights can become zero, which also...