Garbage in, garbage out (or GIGO) is an adage of computer science which is even more important when dealing with machine learning and possibly even more so when dealing with textual data. Garbage in, garbage out means that if we have poorly formatted data, it is likely we will have poor results.
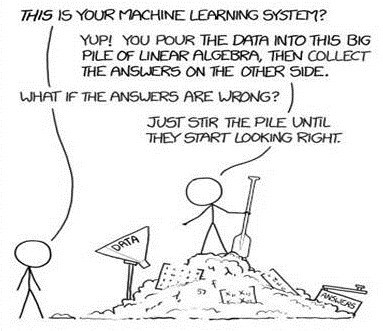
While more data usually leads to a better prediction, it isn't always the same case with text analysis, where more data can result in nonsense results or results which we don't always want. An intuitive example: the part of speech, articles, such as the words a, or the tend to appear a lot in text, but not adding any information to the text, and is usually limited to grammar or structure.
Words such as these which don't provide useful information are called stop words, and these words are often removed from the text before applying text analysis techniques on them. Similarly, sometimes we remove words with very high frequency in the body of text, and words which only appear once or twice – it is highly likely these words will not be useful to our analysis. That being said, this depends heavily on the kind of task being performed - if, for example, we would want to replicate human writing styles, stop words are important because humans many such words when writing. An example of how stop words can also include useful information is in this article, Pastiche detection based on stopword rankings. Exposing impersonators of a Romanian writer [20], is a study identified a certain author using frequency of stop words.
Let's consider another example where we might be dealing with useless data – if searching for influential words or topics in the text, would it make sense to have both the words reading and read in the results? Here, shortening the word reading to read would not lead to any loss of information. But on a similar note, it would make sense to have the words information and inform exist separately in the same body of text, because they could mean different things based on the context. We would then need techniques to shorten words appropriately. Lemmatizing and stemming are two methods we use to tackle this problem and remain two of the core concepts in natural language processing. We will be exploring these two techniques in more detail in Chapter 3, spaCy's Language models.
Even after basic text-processing, our data is still a collection of words. Since machines do not inherently understand the concepts tied to words, we can instead use numbers that represent individual words. The next important step in text analysis is converting words into numbers, whether it is bag-of-words (BOW), or term frequency-inverse document frequency (TF-IDF), which are different ways to count the number of words in each document or sentence. There are also more advanced techniques to represent words such as Word2Vec and GloVe.
We will go into these details and techniques in more detail in the chapter on preprocessing techniques – it is especially important to understand the motivation behind these techniques, and that a computer's output is only as good as the input you feed it.