As we have already mentioned, Apache Spark is a distributed, in-memory, parallel processing system, which needs an associated storage system. So, when you build a big data cluster, you will probably use a distributed storage system such as Hadoop, as well as tools to move data such as Sqoop, Flume, and Kafka.
We wanted to introduce the idea of edge nodes in a big data cluster. These nodes in the cluster will be client-facing, on which reside the client-facing components such as Hadoop NameNode or perhaps the Spark master. Majority of the big data cluster might be behind a firewall. The edge nodes would then reduce the complexity caused by the firewall as they would be the only points of contact accessible from outside. The following figure shows a simplified big data cluster:
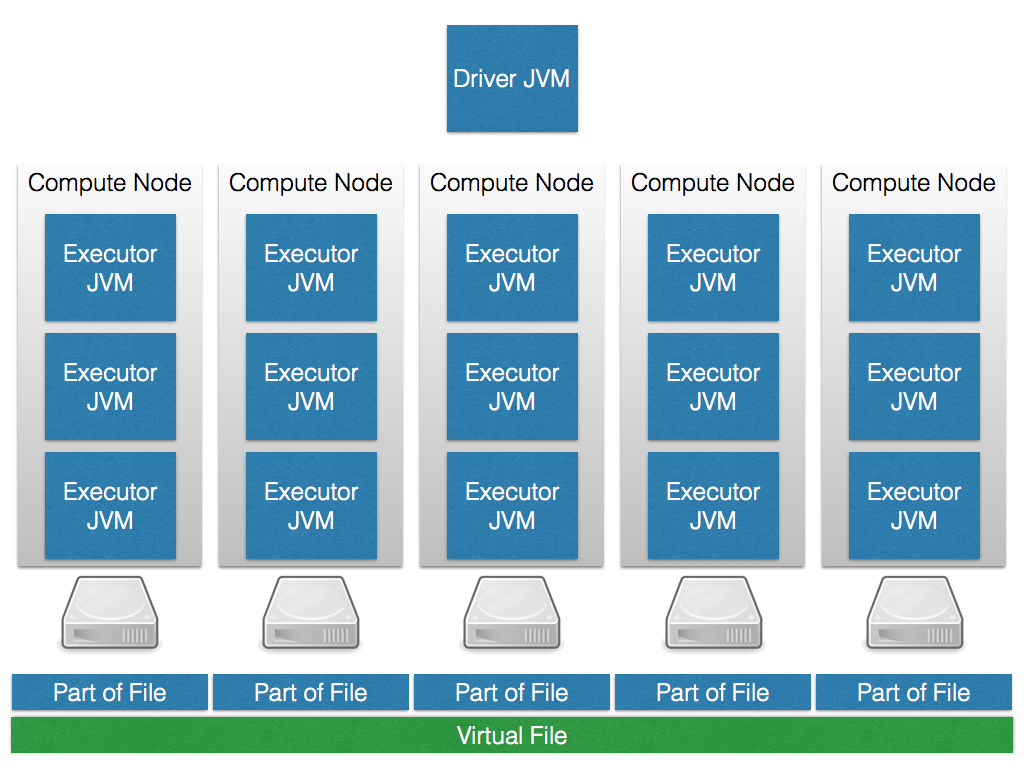
It shows five simplified cluster nodes with executor JVMs, one per CPU core, and the Spark Driver JVM sitting outside the cluster. In addition, you see the disk directly attached to the nodes. This is called the JBOD (just a bunch of disks) approach. Very large files are partitioned over the disks and a virtual filesystem such as HDFS makes these chunks available as one large virtual file. This is, of course, stylized and simplified, but you get the idea.
The following simplified component model shows the driver JVM sitting outside the cluster. It talks to the Cluster Manager in order to obtain permission to schedule tasks on the worker nodes because the Cluster Manager keeps track of resource allocation of all processes running on the cluster.
As we will see later, there is a variety of different cluster managers, some of them also capable of managing other Hadoop workloads or even non-Hadoop applications in parallel to the Spark Executors. Note that the Executor and Driver have bidirectional communication all the time, so network-wise, they should also be sitting close together:
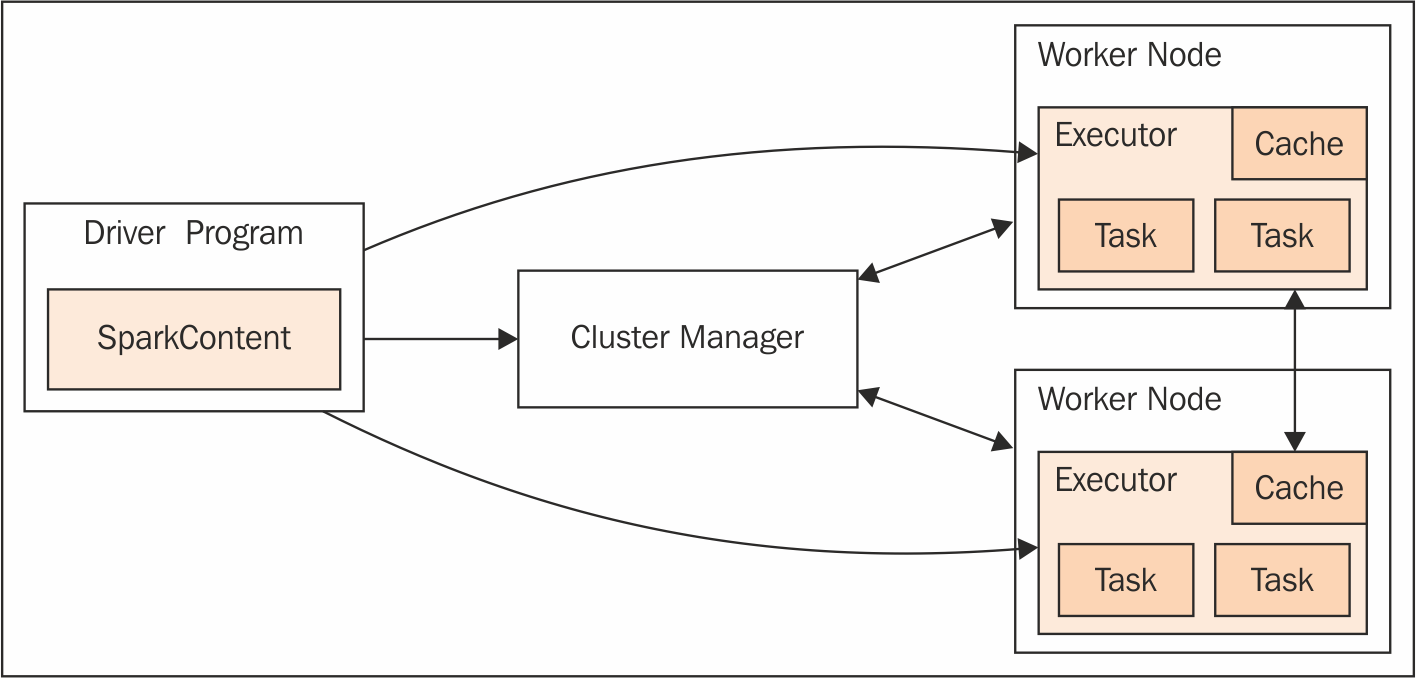
Generally, firewalls, while adding security to the cluster, also increase the complexity. Ports between system components need to be opened up so that they can talk to each other. For instance, Zookeeper is used by many components for configuration. Apache Kafka, the publish/subscribe messaging system, uses Zookeeper to configure its topics, groups, consumers, and producers. So, client ports to Zookeeper, potentially across the firewall, need to be open.
Finally, the allocation of systems to cluster nodes needs to be considered. For instance, if Apache Spark uses Flume or Kafka, then in-memory channels will be used. The size of these channels, and the memory used, caused by the data flow, need to be considered. Apache Spark should not be competing with other Apache components for memory usage. Depending upon your data flows and memory usage, it might be necessary to have Spark, Hadoop, Zookeeper, Flume, and other tools on distinct cluster nodes. Alternatively, resource managers such as YARN, Mesos, or Docker can be used to tackle this problem. In standard Hadoop environments, YARN is most likely.
Generally, the edge nodes that act as cluster NameNode servers or Spark master servers will need greater resources than the cluster processing nodes within the firewall. When many Hadoop ecosystem components are deployed on the cluster, all of them will need extra memory on the master server. You should monitor edge nodes for resource usage and adjust in terms of resources and/or application location as necessary. YARN, for instance, is taking care of this.
This section has briefly set the scene for the big data cluster in terms of Apache Spark, Hadoop, and other tools. However, how might the Apache Spark cluster itself, within the big data cluster, be configured? For instance, it is possible to have many types of the Spark cluster manager. The next section will examine this and describe each type of the Apache Spark cluster manager.